Memristive-based Mixed-signal CGRA for Accelerating Deep Neural Network Inference.
ACM Trans. Design Autom. Electr. Syst.(2023)
摘要
In this paper, a mixed-signal coarse-grained reconfigurable architecture (CGRA) for accelerating inference in deep neural networks (DNNs) is presented. It is based on performing dot-product computations using analog computing to achieve a considerable speed improvement. Other computations are performed digitally. In the proposed structure (called MX-CGRA), analog tiles consisting of memristor crossbars are employed. To reduce the overhead of converting the data between analog and digital domains, we utilize a proper interface between the analog and digital tiles. In addition, the structure benefits from an efficient memory hierarchy where the data is moved as close as possible to the computing fabric. Moreover, to fully utilize the tiles, we define a set of micro instructions to configure the analog and digital domains. Corresponding context words used in the CGRA are determined by these instructions (generated by a companion compiler tool). The efficacy of the MX-CGRA is assessed by modeling the execution of state-of-the-art DNN architectures on this structure. The architectures are used to classify images of the ImageNet dataset. Simulation results show that, compared to the previous mixed-signal DNN accelerators, on average, a higher throughput of 2.35 × is achieved.
更多查看译文
关键词
deep neural network inference,neural network,memristive-based,mixed-signal
AI 理解论文
溯源树
样例
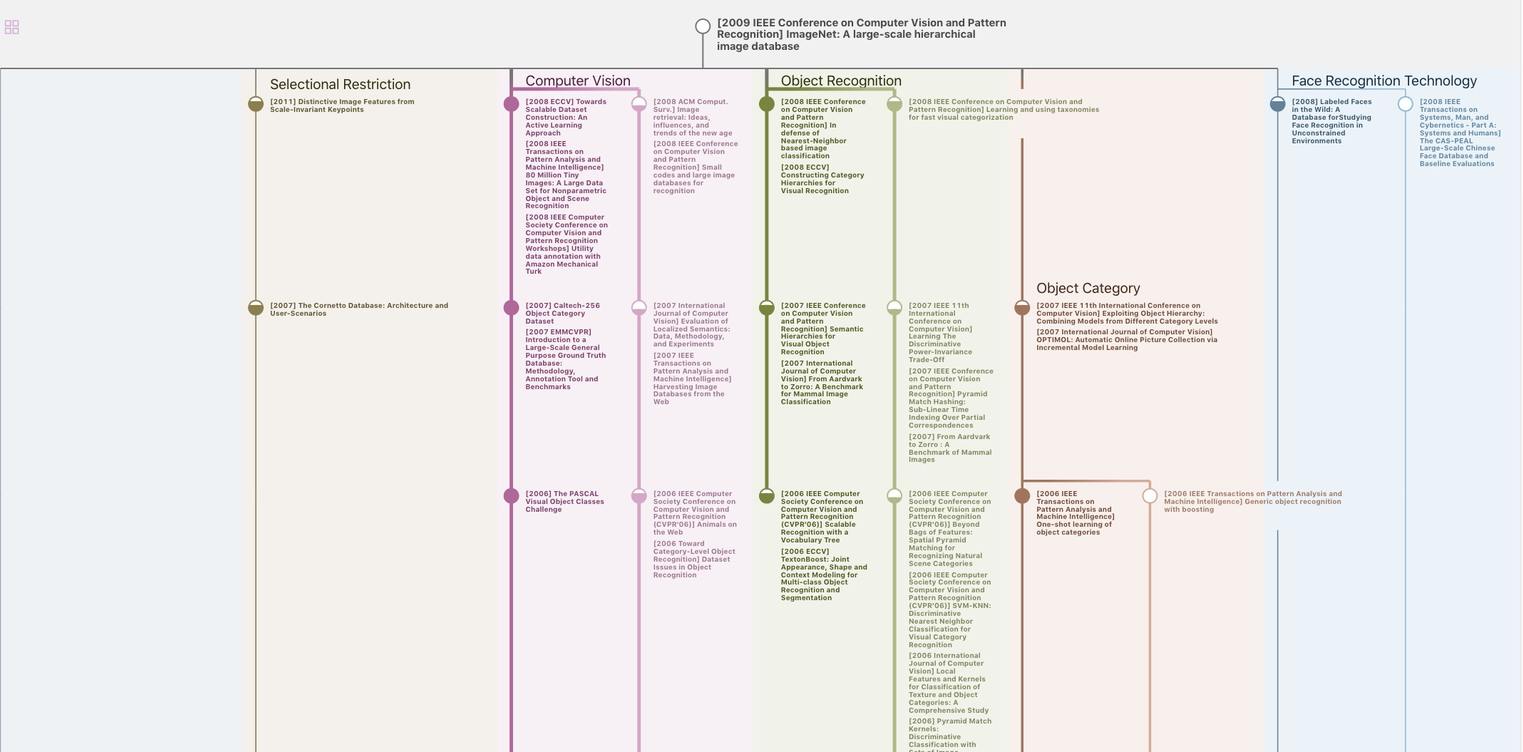
生成溯源树,研究论文发展脉络
Chat Paper
正在生成论文摘要