Seismic Data Interpolation Based on Spectrally Normalized Generative Adversarial Network.
IEEE Trans. Geosci. Remote. Sens.(2023)
摘要
Missing traces are a common problem in seismic data acquisition, which can affect the quality of subsequent processing and interpretation. Therefore, seismic data interpolation is an essential step to recover the missing information. Recently, deep learning has emerged as a powerful tool for seismic data interpolation, especially generative adversarial networks (GANs). GAN can generate realistic data by learning from existing samples. In this article, we propose an improved GAN for seismic data interpolation. The generator is set as U-Net, which could extract more features from the input data via skip connections. For the discriminator, we add a spectral normalization layer to preserve the information content of the discriminator's weights. The Wasserstein loss function is used to stabilize the training process. With those changes, the improved GAN outperforms the traditional GAN. Both synthetic and field data tests demonstrate its effectiveness. Our proposed network can intelligently interpolate seismic data with a high signal-to-noise ratio (SNR) and enhance the efficiency of seismic data processing and analysis.
更多查看译文
关键词
Deep learning,seismic data interpolation,spectral normalization generative adversarial network (SN-GAN),training set
AI 理解论文
溯源树
样例
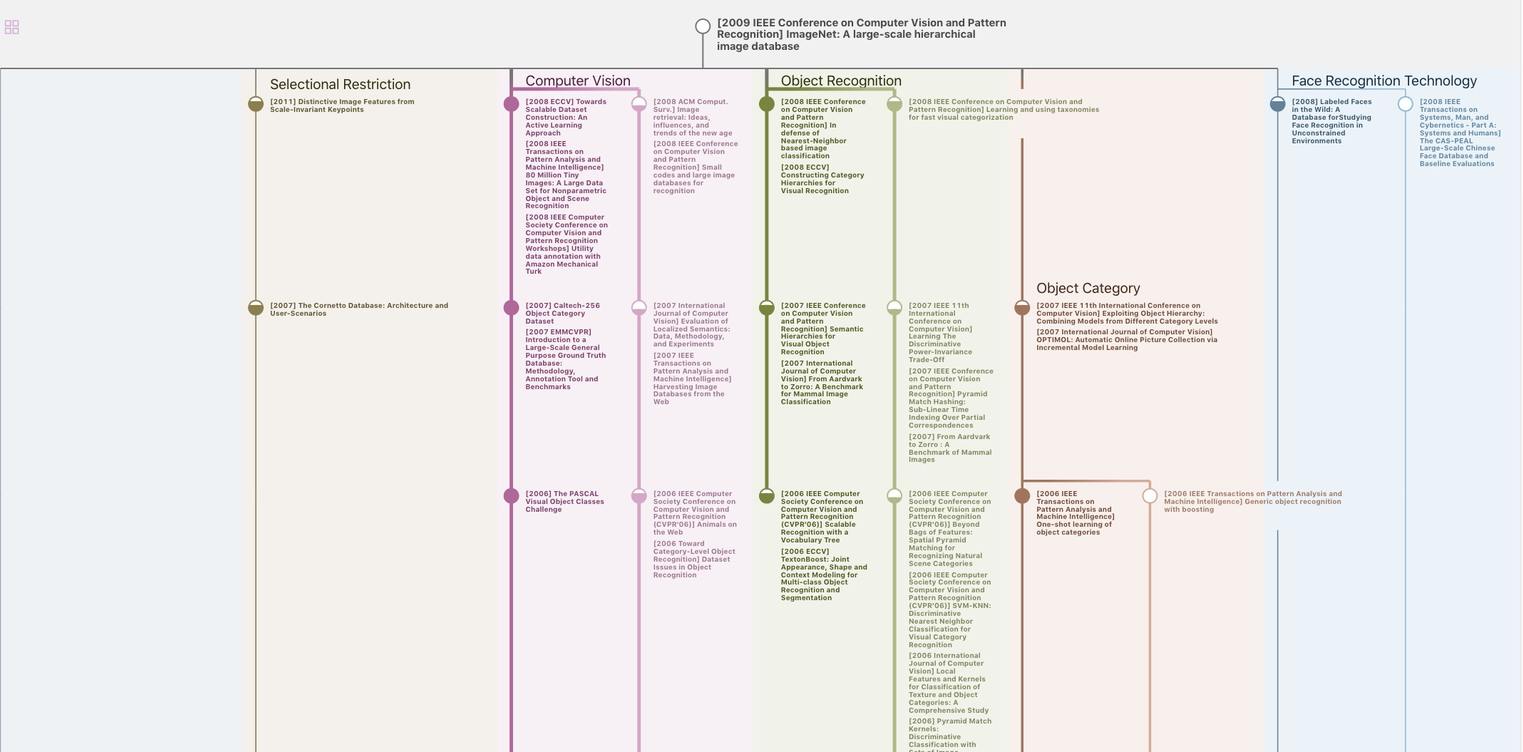
生成溯源树,研究论文发展脉络
Chat Paper
正在生成论文摘要