Exploitation of Vulnerabilities: A Topic-Based Machine Learning Framework for Explaining and Predicting Exploitation.
Inf.(2023)
摘要
Security vulnerabilities constitute one of the most important weaknesses of hardware and software security that can cause severe damage to systems, applications, and users. As a result, software vendors should prioritize the most dangerous and impactful security vulnerabilities by developing appropriate countermeasures. As we acknowledge the importance of vulnerability prioritization, in the present study, we propose a framework that maps newly disclosed vulnerabilities with topic distributions, via word clustering, and further predicts whether this new entry will be associated with a potential exploit Proof Of Concept (POC). We also provide insights on the current most exploitable weaknesses and products through a Generalized Linear Model (GLM) that links the topic memberships of vulnerabilities with exploit indicators, thus distinguishing five topics that are associated with relatively frequent recent exploits. Our experiments show that the proposed framework can outperform two baseline topic modeling algorithms in terms of topic coherence by improving LDA models by up to 55%. In terms of classification performance, the conducted experiments-on a quite balanced dataset (57% negative observations, 43% positive observations)-indicate that the vulnerability descriptions can be used as exclusive features in assessing the exploitability of vulnerabilities, as the "best" model achieves accuracy close to 87%. Overall, our study contributes to enabling the prioritization of vulnerabilities by providing guidelines on the relations between the textual details of a weakness and the potential application/system exploits.
更多查看译文
关键词
text mining, exploits, fuzzy clustering, topic extraction, security vulnerabilities, machine learning
AI 理解论文
溯源树
样例
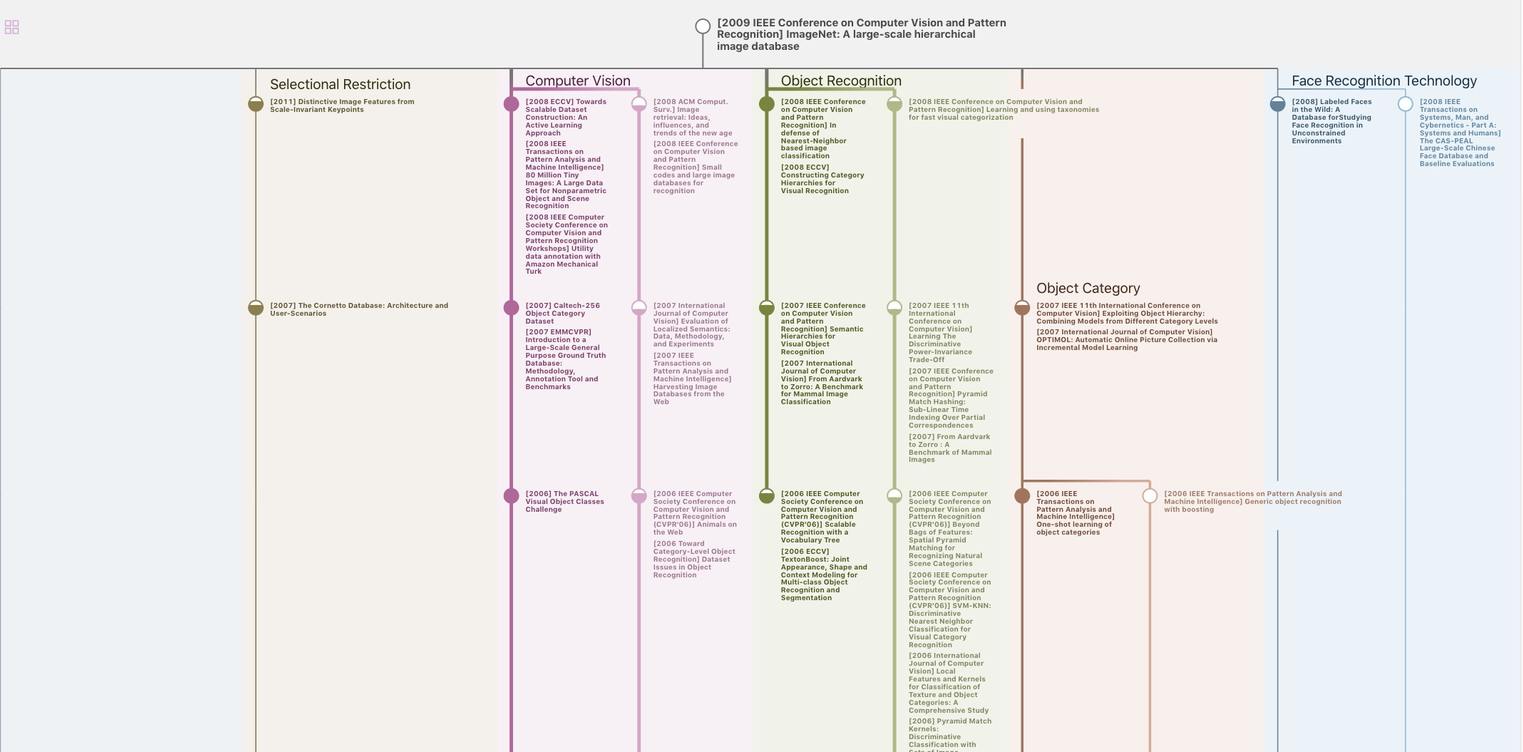
生成溯源树,研究论文发展脉络
Chat Paper
正在生成论文摘要