Study on transfer learning of shield tunneling parameters based on LSTM model.
EITCE(2022)
摘要
During the excavation of the shield tunneling machine, the performance of the existing methods for shield tunneling machine parameter prediction often require a large amount of data to train a relatively accurate prediction model, which is in great contradiction with the limited data collected when the subway line is beginning to be constructed. In this paper, a set of transfer learning model based on long short-term memory (LSTM) is designed and built to improve the prediction process of shield tunneling machine parameter when the amount of data is small. The model selects the cutterhead torque as the prediction target and uses the Pearson coefficient method to filter the input features that are highly correlated with the prediction target. During transfer learning, the pre-training of the transfer model was first performed on the Beijing subway dataset. The best transfer learning scheme was selected by freezing the pre-training models with different layers and the optimal transfer learning model was transferred to the Tianjin Metro. Comparing the results of transfer learning and directly using LSTM model training with different data volumes, the results show that the use of transfer learning can effectively improve the accuracy of the prediction model when the data volume is small.
更多查看译文
AI 理解论文
溯源树
样例
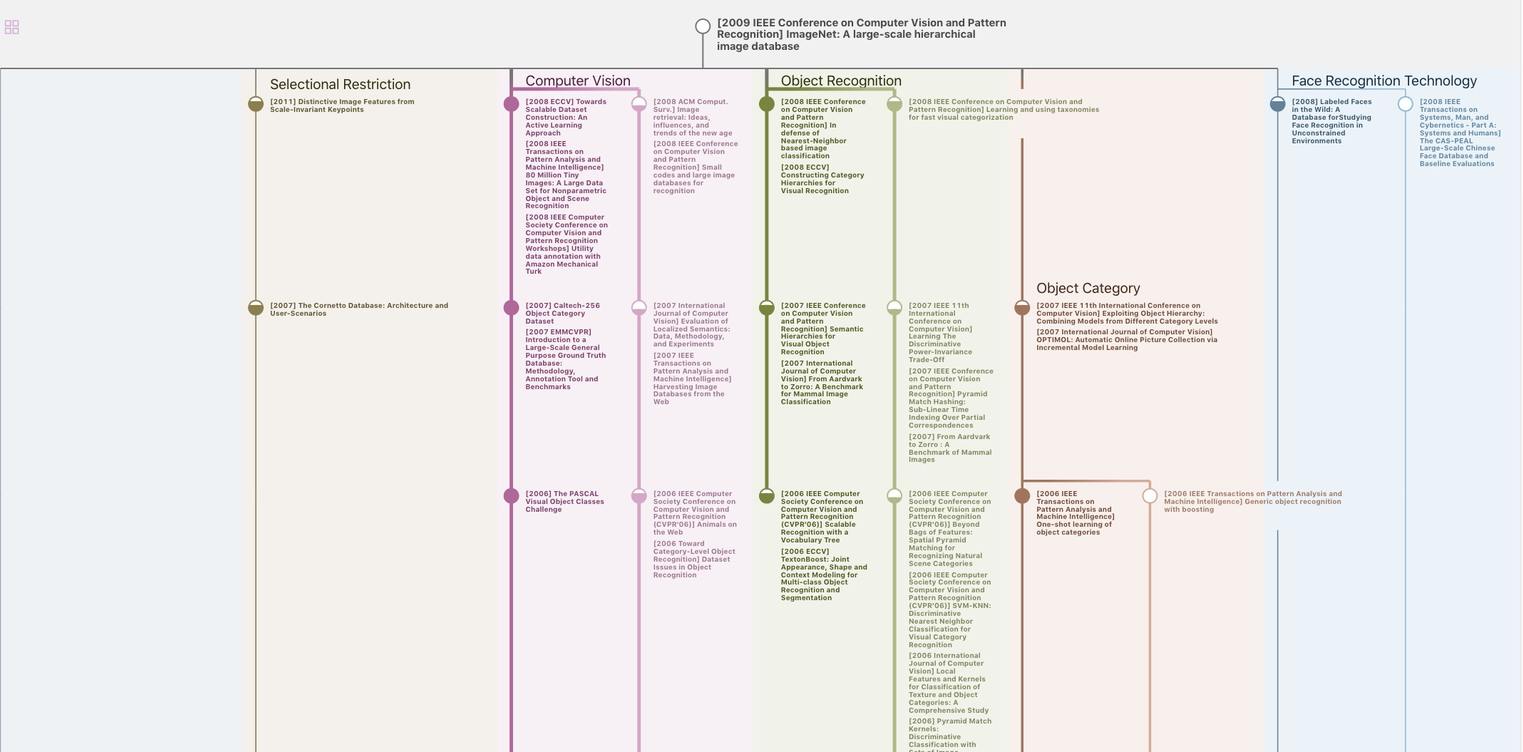
生成溯源树,研究论文发展脉络
Chat Paper
正在生成论文摘要