Segmentation of fatigue cracks in ancillary steel structures using deep learning convolutional neural networks.
AIM(2023)
摘要
Regular structural inspections to detect cracks in ancillary structures are necessary to prevent fatigue cracks from compromising a structure's safety and durability. As the most common inspection, visual methods for ancillary structures are limited because they are time-consuming, costly, and require a great deal of experience. The inspection can benefit greatly from automation through implementation of deep learning. However, there is no comprehensive annotated dataset for deep learning to detect cracks in ancillary structures. In this work, a dataset containing 250 images were collected from previous studies and 30 images were collected of in-service ancillary structures. This dataset was annotated by labeling image tiles and bounding box for AlexNet, and Faster RCNN (FRCNN), respectively. Data augmentation, such as change in color and crack orientation, were performed to increase the size of training dataset to 1400 images. Moreover, an image of a fatigue crack was superimposed on images of intact and in-service ancillary structures to increase the dataset size to 1500 sub-images. The image labeler mode was trained in fully trained, transfer learning, and classifier modes. Additionally, bounding box annotation was used to label fatigue crack as an object in 200 images with cracks. Next, FRCNN as an object detection algorithm was used to determine the location of cracks in ancillary structures. FRCNN and AlexNet with transfer learning can be used to determine the location of cracks in ancillary structures with an accuracy rate higher than 90% and 93%.
更多查看译文
关键词
fatigue crack,ancillary structure,deep learning,faster RCNN,data augmentation,structural condition assessment
AI 理解论文
溯源树
样例
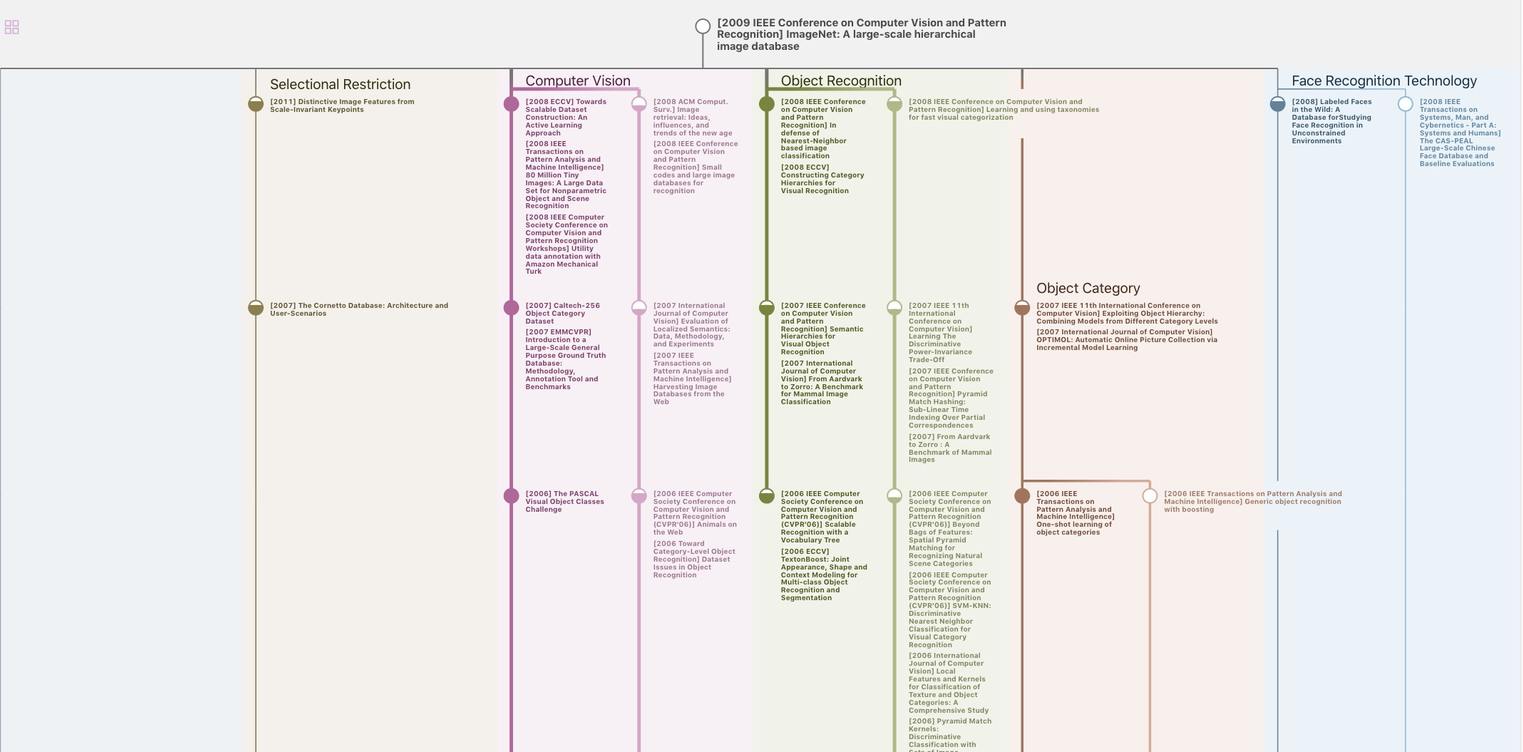
生成溯源树,研究论文发展脉络
Chat Paper
正在生成论文摘要