A Simple Mixed-Supervised Learning Method for Salient Object Detection.
ICIC (5)(2023)
摘要
Weakly supervised salient object detection aims to address the limitations of fully supervised methods that heavily rely on pixel-level data. However, the sparse nature of weak labels often results in suboptimal detection accuracy. Drawing inspiration from human visual attention mechanisms, we propose a Mixed-Supervised Learning method to mitigate this issue. Mixed-Supervised Learning refers to training a neural network with hybrid data. Specifically, we propose a two-stage training strategy. In stage I, the model is supervised by a large number of scribble annotations so that it can roughly locate salient objects. In stage II, a small number of pixel-level labels are used for learning to endow the model with detail decoding capability. Our training strategy decomposes the SOD task into two sub-tasks, object localization and detail refinement, and we design a corresponding network, LRNet, which includes the supplementary detail information, a Feature Attention module (FA), and a Detail Refinement module (DF). The two-stage training strategy is simple and generalizable. Extensive experiments demonstrate the effectiveness of the training strategy, and our model detection accuracy surpasses the existing state-of-the-art models of weakly supervised learning, even reaching fully supervised results. Besides, experiments on COD and RSI SOD tasks demonstrate the generality of our method. Our code will be released at https://github.com/nightmengna/LRNet .
更多查看译文
关键词
detection,learning method,object,mixed-supervised
AI 理解论文
溯源树
样例
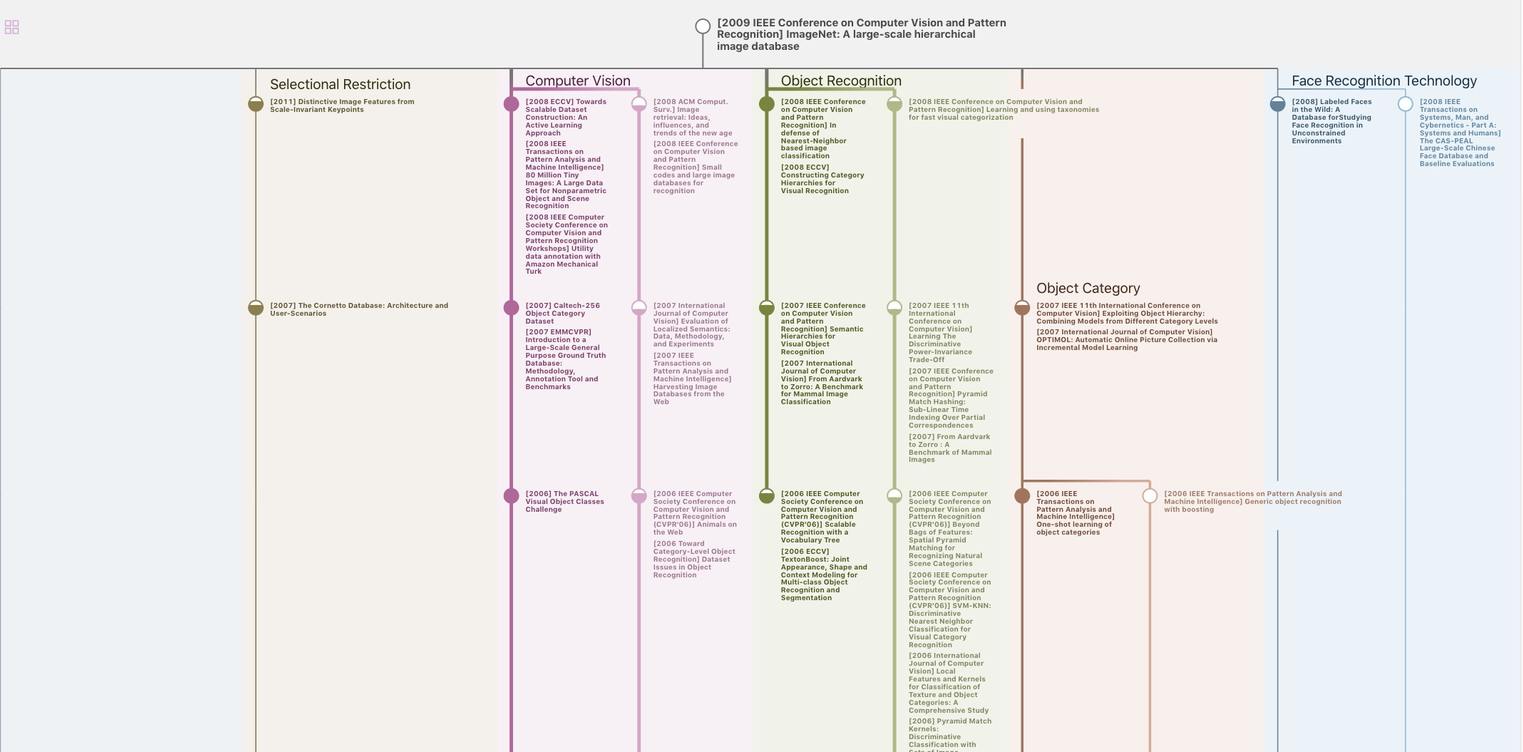
生成溯源树,研究论文发展脉络
Chat Paper
正在生成论文摘要