Music Emotion Recognition Using Multi-head Self-attention-Based Models.
ICIC (4)(2023)
摘要
Music Emotion Recognition (MER) has been a major challenge in Music Information Retrieval (MIR) and is essential in many fields, such as music psychotherapy, individualized instruction, and music recommendation. Some existing approaches aim to extract emotion-related features through deep neural networks. Unfortunately, these methods perform poorly in outputting music emotion continuously, which is important for emotion perception. More recently, self-attention-based models have been proposed to learn emotion-related information by determining which part should be the focus and ignoring irrelevant and noisy data. However, since music emotion has much multi-dimensional semantic information, they cannot fully excavate the multi-dimensional implicit semantic information of the feature sequence and will ignore some of the emotion-related information. Aiming at addressing these issues, we present a new approach for MER that can extract continuous features and then excavate multi-dimensional information sensitive to emotion effectively. Firstly, the study suggests a neural network-based method to extract continuous features, intending to mine emotion-abundant features. After that, we design a classifier with the multi-head self-attention-based model to excavate multi-dimensional information for music emotion recognition. Finally, we conduct experiments on a real dataset EMOPIA. Experimental results show our methods surpass the baseline on the corresponding modalities (symbolic and acoustic) at an accuracy of 6.1% and 4.4% on the same dataset, which verifies the superiority of our method.
更多查看译文
关键词
emotion,music,multi-head,self-attention-based
AI 理解论文
溯源树
样例
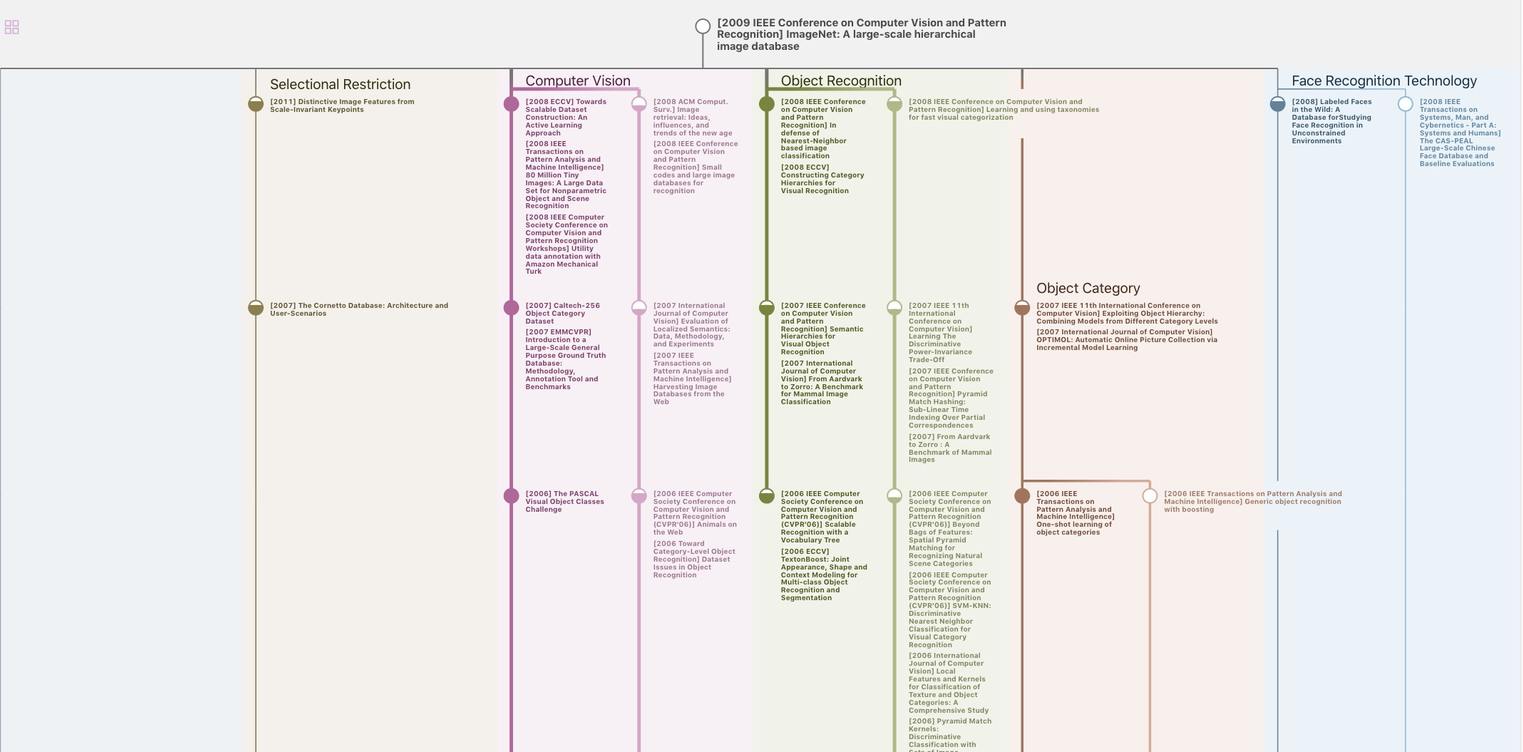
生成溯源树,研究论文发展脉络
Chat Paper
正在生成论文摘要