Surrogate Modeling for Soliton Wave of Nonlinear Partial Differential Equations via the Improved Physics-Informed Deep Learning.
ICIC (2)(2023)
摘要
In recent years, the application of artificial intelligence to natural science research has gained significant attention, particularly the use of deep learning for solving partial differential equations (PDEs). Traditional methods of solving PDEs are often computationally expensive, time-consuming, and complex to implement. In contrast, deep learning-based methods offer simplicity, flexibility, and efficiency. In this area, physics-informed neural networks (PINNs) have been successfully applied to solve PDEs, demonstrating great potential. Solitary waves are an important component of nonlinear scientific research, with theoretical applications in many fields. In this paper, we improve the original PINNs by incorporating gradient information constraints, which provide stronger physical constraints. Moreover, adaptive learning methods are used to update the weight coefficients of the loss function and dynamically adjust the proportion of each constraint term in the loss function to expedite training and enhance effectiveness. In the experimental component, we apply the improved PINN to the numerical simulation of solitary waves for partial differential equations. In particular, we focus on the Caudrey-Dodd-Gibbon-Sawada-Kotera (CDGSK) equation’s single soliton and multiple soliton solutions and evaluate the accuracy of the solitary wave simulation results using error analysis. The experimental findings demonstrate that the improved PINNs outperform traditional PINNs, particularly in the CDGSK equation, with shorter training times and more accurate prediction results.
更多查看译文
关键词
soliton wave,nonlinear partial differential equations,surrogate modeling,differential equations,physics-informed
AI 理解论文
溯源树
样例
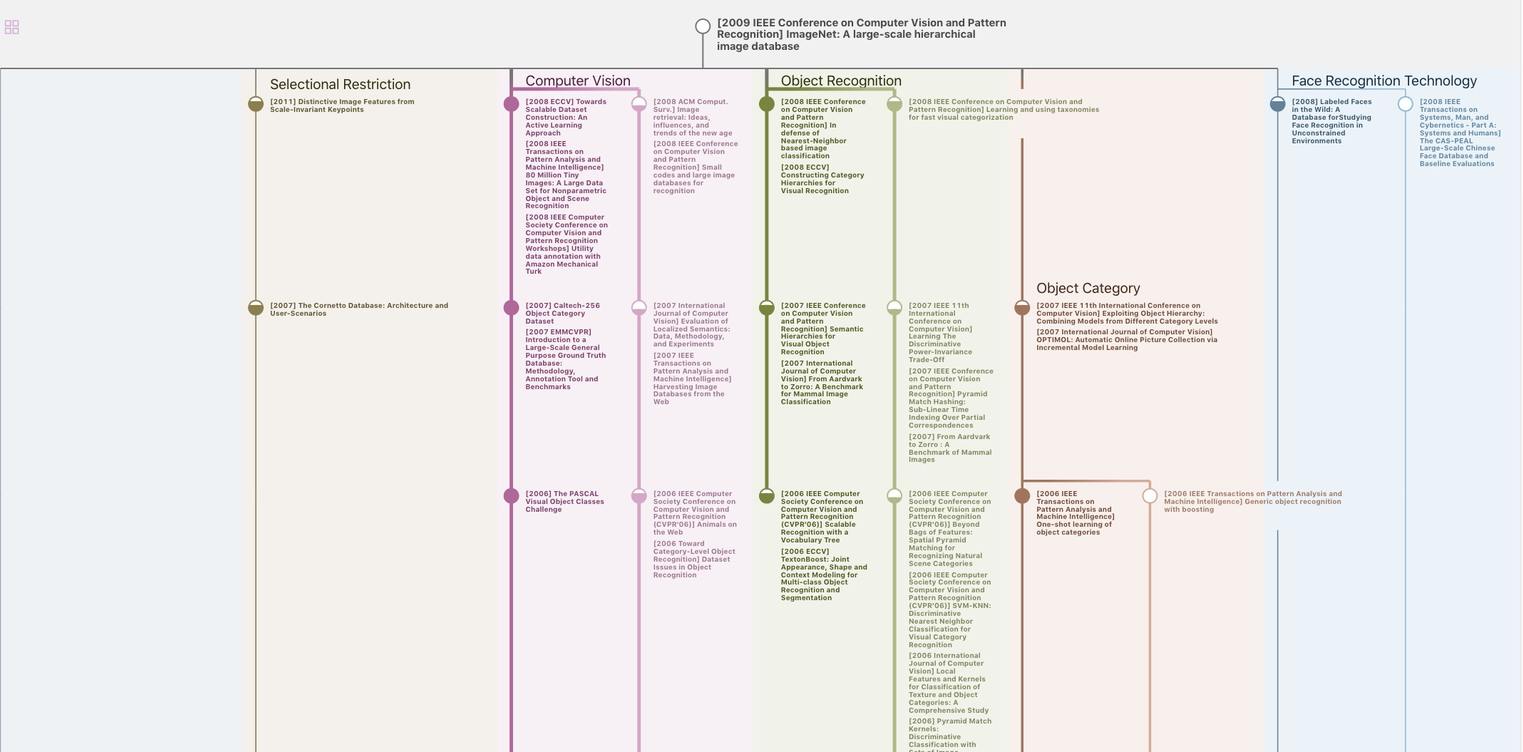
生成溯源树,研究论文发展脉络
Chat Paper
正在生成论文摘要