Simple but Effective: Keyword-Based Metric Learning for Event Sentence Coreference Identification.
ICIC (4)(2023)
摘要
Event sentence coreference identification (ESCI) is a fundamental task of news event detection and tracking which aims to group sentences according to events they refer to. Most recent efforts address this task by means of identifying coreferential event sentence pairs. Currently, frameworks based on pre-trained language models like Sentence-BERT (SBERT) are widely used for sentence pair tasks. However, SBERT lacks keyword awareness, while the local features of sentences can demonstrate a strong correlation with the event topic. In addition, the strategy of encoding the whole sentence is less flexible and more time-consuming. After reconsidering the significance of keywords in ESCI task, we propose KeyML, a simple keyword-based metric learning approach which leverages both lexical and semantic features of keywords to capture subject patterns of events. Specifically, a Siamese network is adapted to optimize distance metrics of keyword embeddings, resulting in more separable similarity of event sentence pairs. Then, KeyML considers keywords of data with different granularity and exploits three training strategies, along with their corresponding sampling methods, to investigate co-occurrence relationships. Experimental results show that KeyML outperforms SBERT and SimCSE on three datasets and demonstrate the effectiveness and rationality of our method.
更多查看译文
关键词
metric learning,event,keyword-based
AI 理解论文
溯源树
样例
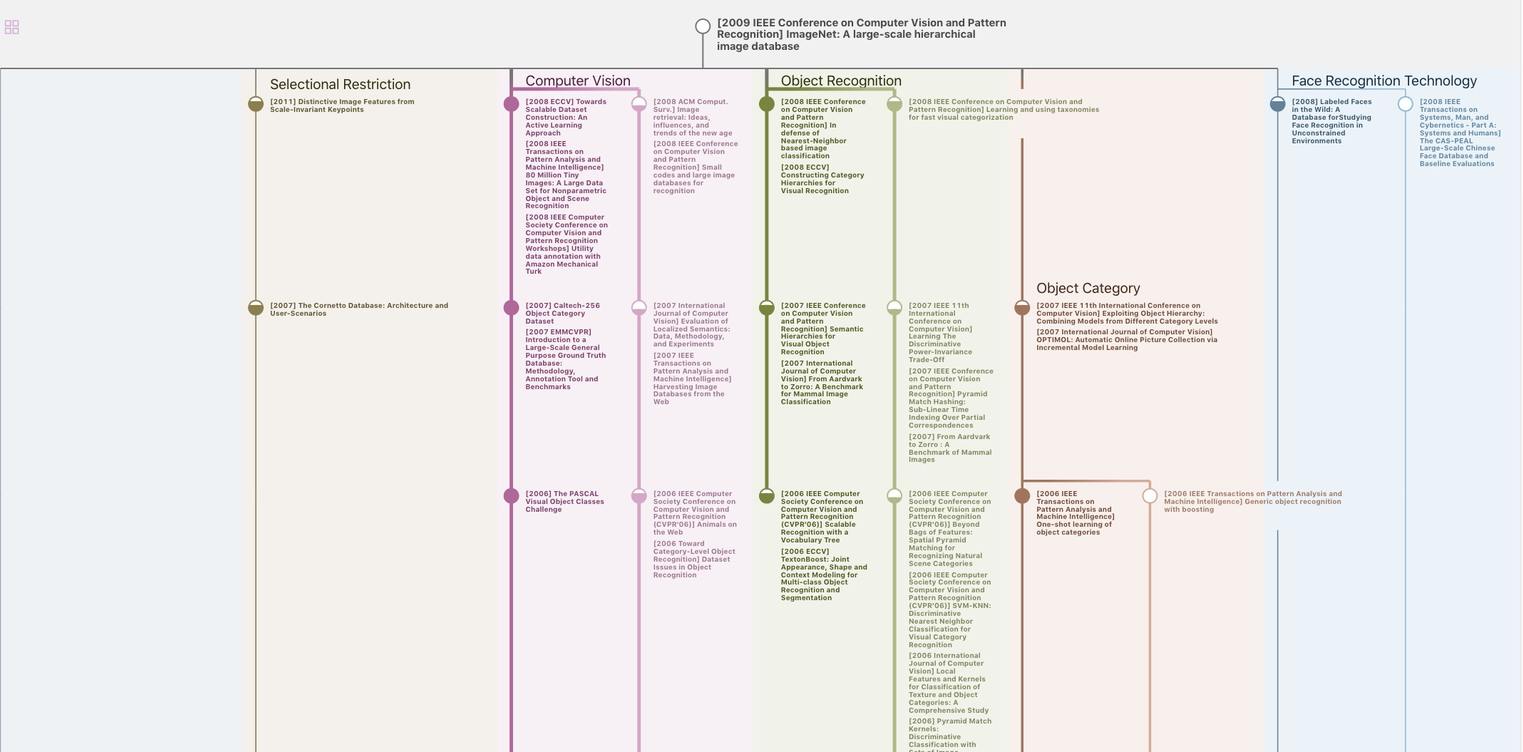
生成溯源树,研究论文发展脉络
Chat Paper
正在生成论文摘要