Hyper-heuristic Ant Colony Optimization Algorithm for Multi-objective Two-Echelon Vehicle Routing Problem with Time Windows.
ICIC (1)(2023)
摘要
In this paper, a hyper-heuristic ant colony optimization algorithm (HHACOA) is proposed to solve the multi-objective two-echelon vehicle routing problem with time windows (MO2E-VRPTW). Minimizing the total carbon emissions and maximizing the customer satisfaction are the objectives that the MO2E-VRPTW needs to optimize. On the one hand, at the low-level of HHACOA, the quality of the initial population is improved by using two problem-based heuristic rules, and a permutation consisting of nine neighborhood operations is used as an algorithm to perform a series of operations on each individual in the low-level population. Then HHACOA can be driven to search different regions in the solution space. On the other hand, at the high-level of HHACOA, the ant colony algorithm is used to learn the permutation information acting on each low-level individual, and the new permutation are generated based on the probabilistic transition matrix. A permutation is an individual in the high-level population. Then HHACOA can be quickly guided to reach regions where high-quality non-dominated solutions are concentrated. Finally, the simulation experiments and algorithm comparisons verify the effectiveness of the proposed HHACOA.
更多查看译文
关键词
ant colony optimization,routing,hyper-heuristic,multi-objective,two-echelon
AI 理解论文
溯源树
样例
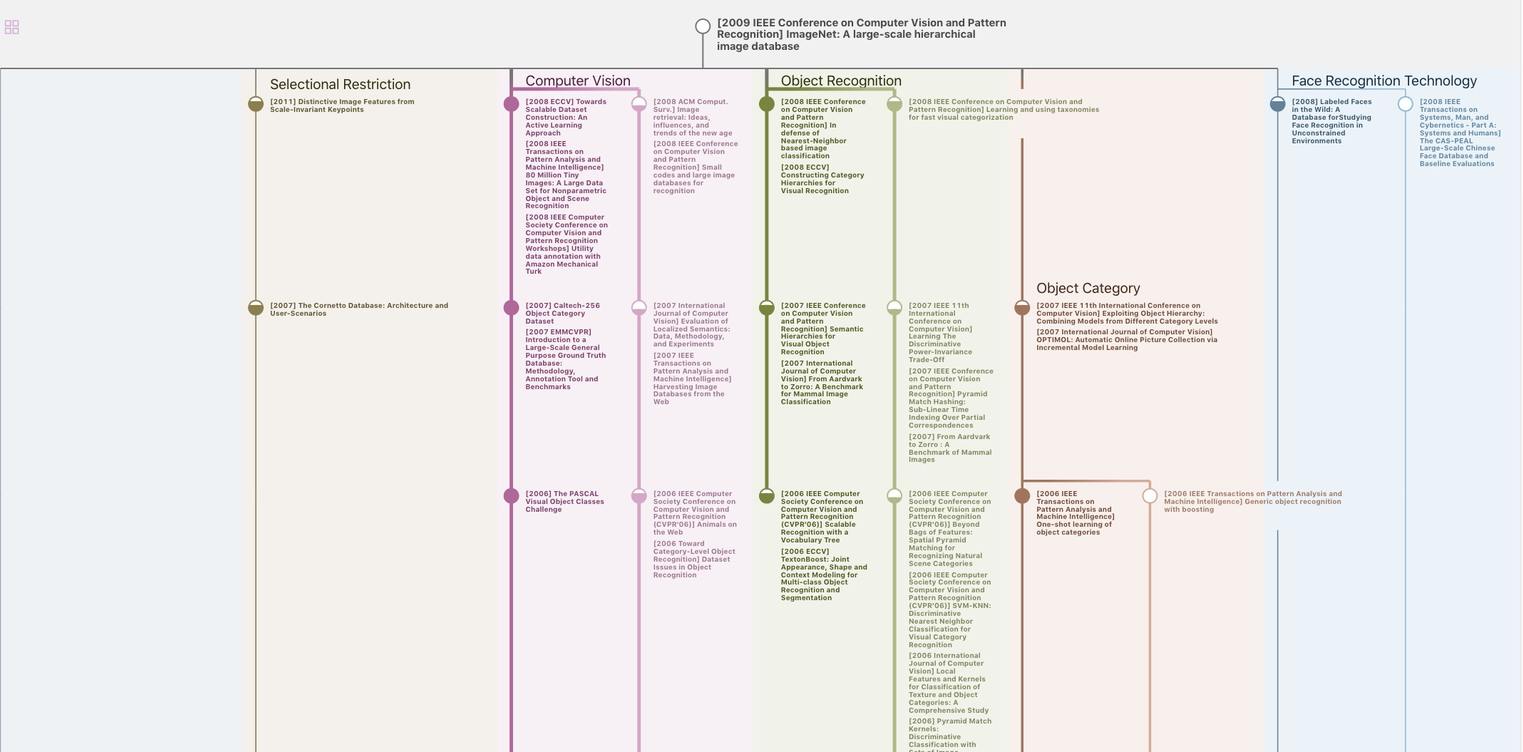
生成溯源树,研究论文发展脉络
Chat Paper
正在生成论文摘要