Hyper-Stacked: Scalable and Distributed Approach to AutoML for Big Data.
CD-MAKE(2023)
摘要
The emergence of Machine Learning (ML) has altered how researchers and business professionals value data. Applicable to almost every industry, considerable amounts of time are wasted creating bespoke applications and repetitively hand-tuning models to reach optimal performance. For some, the outcome may be desired; however, the complexity and lack of knowledge in the field of ML become a hindrance. This, in turn, has seen an increasing demand for the automation of the complete ML workflow (from data preprocessing to model selection), known as Automated Machine Learning (AutoML). Although AutoML solutions have been developed, Big Data is now seen as an impediment for large organisations with massive data outputs. Current methods cannot extract value from large volumes of data due to tight coupling with centralised ML libraries, leading to limited scaling potential. This paper introduces Hyper-Stacked, a novel AutoML component built natively on Apache Spark. Hyper-Stacked combines multi-fidelity hyperparameter optimisation with the Super Learner stacking technique to produce a strong and diverse ensemble. Integration with Spark allows for a parallelised and distributed approach, capable of handling the volume and complexity associated with Big Data. Scalability is demonstrated through an in-depth analysis of speedup, sizeup and scaleup.
更多查看译文
关键词
automl,big data,hyper-stacked
AI 理解论文
溯源树
样例
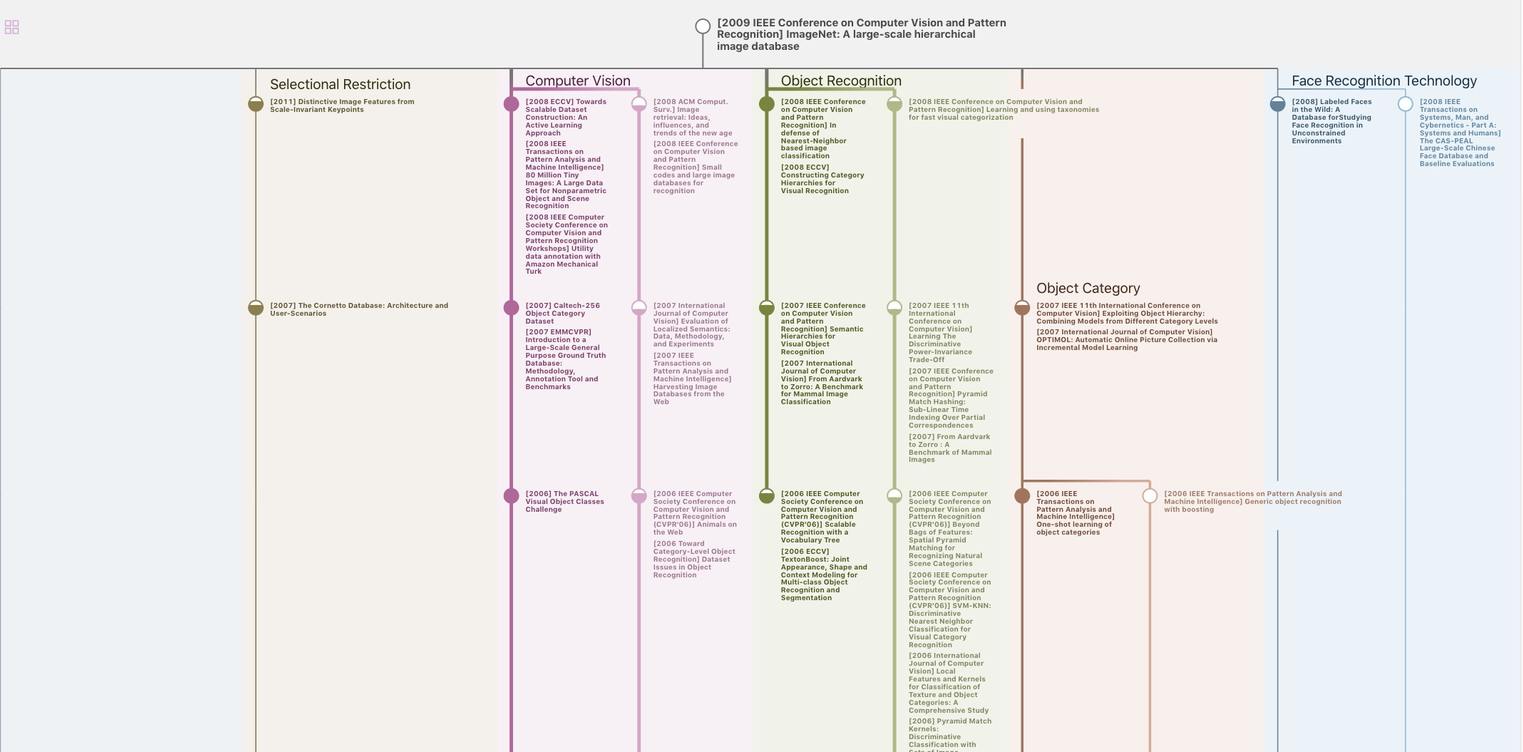
生成溯源树,研究论文发展脉络
Chat Paper
正在生成论文摘要