An Online Continuous Semantic Segmentation Framework With Minimal Labeling Efforts.
SMARTCOMP(2023)
摘要
The annotation load for a new dataset has been greatly decreased using domain adaptation based semantic segmentation, which iteratively constructs pseudo labels on unlabeled target data and retrains the network. However, realistic segmentation datasets are often imbalanced, with pseudo-labels tending to favor certain "head" classes while neglecting other "tail" classes. This can lead to an inaccurate and noisy mask. To address this issue, we propose a novel hard sample mining strategy for an active domain adaptation based semantic segmentation network, with the aim of automatically selecting a small subset of labeled target data to fine-tune the network. By calculating class-wise entropy, we are able to rank the difficulty level of different samples. We use a fusion of focal loss and regional mutual information loss instead of cross-entropy loss for the domain adaptation based semantic segmentation network. Our entire framework has been implemented in real-time using the Robotics Operating System (ROS) with a server PC and a small Unmanned Ground Vehicle (UGV) known as the ROSbot2.0 Pro. This implementation allows ROSbot2.0 Pro to access any type of data at any time, enabling it to perform a variety of tasks with ease. Our approach has been thoroughly evaluated through a series of extensive experiments, which demonstrate its superior performance compared to existing state-of-the-art methods. Remarkably, by using just 20% of hard samples for fine-tuning, our network has achieved a level of performance that is comparable (approximate to 88%) to that of a fully supervised approach, with mIOU scores of 60.51% in the In-house dataset.
更多查看译文
关键词
Semantic Segmentation, Domain Adaptation, Active Learning, Continual Learning, Robotics Operating System
AI 理解论文
溯源树
样例
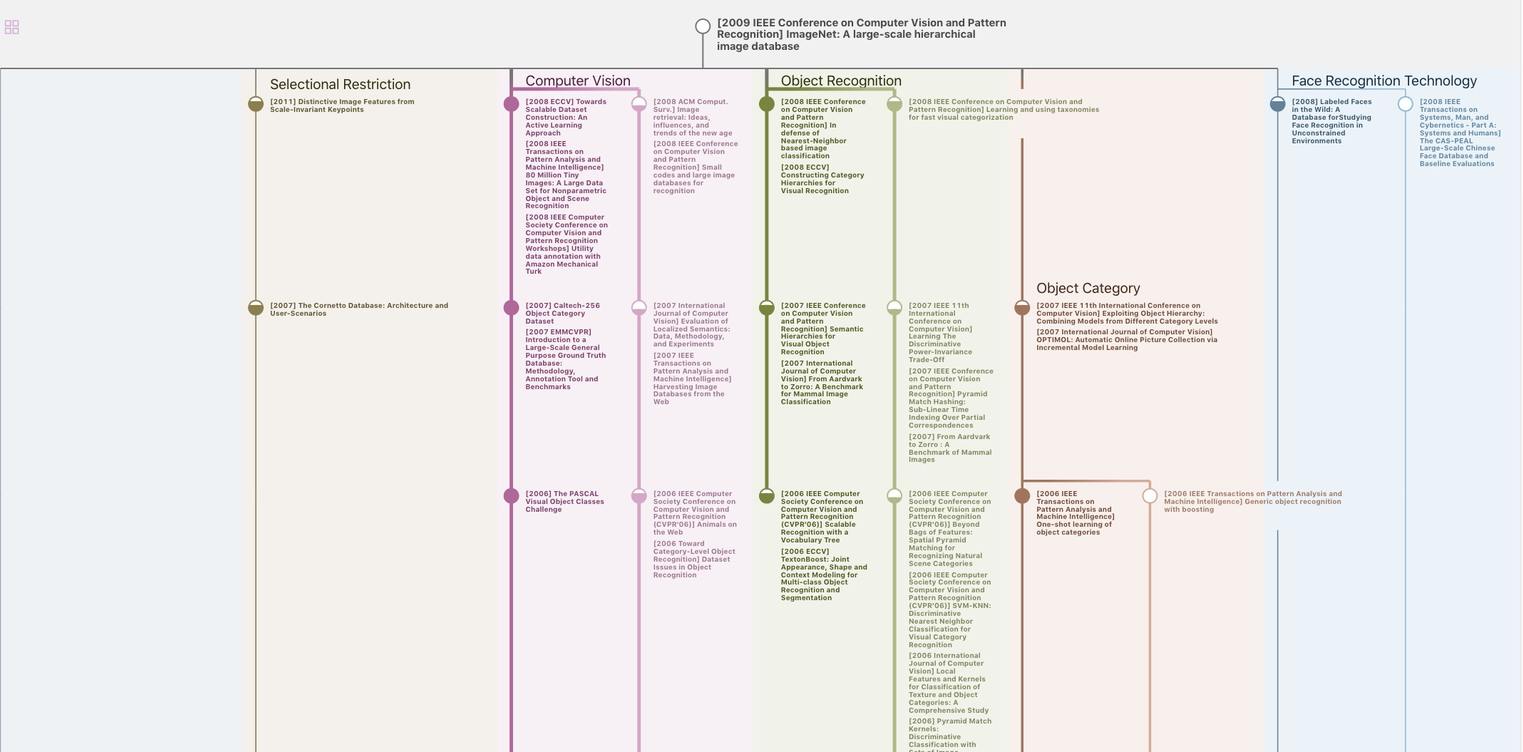
生成溯源树,研究论文发展脉络
Chat Paper
正在生成论文摘要