SimDE: A Simple Domain Expansion Approach for Single-source Domain Generalization.
CVPR Workshops(2023)
Abstract
Single domain generalization challenges model generalizability to unseen target domains when only one source domain is provided for training. To tackle this problem, domain expansion is adopted to learn domain-invariant information by exposing the model to more domain variations, which is still under-explored in previous work. In this paper, we propose a new and simplified objective for learning the desirable domain expansions by generating unconfident samples through the combination of entropy maximization and cross-entropy minimization. We further devise a novel framework that trains a pair of generators from different views by switching the guidance from the dual classifiers. In this way, the resulting method called Simple Domain Expansion (SimDE) can learn diverse domain expansions effectively and efficiently. Extensive experiments on prevalent single domain generalization benchmarks demonstrate the superiority of our method by offering improved results over the state-of-the-arts methods.
MoreTranslated text
Key words
cross-entropy minimization,desirable domain expansions,diverse domain expansions,domain variations,domain-invariant information,prevalent single domain generalization benchmarks,SimDE,simple domain expansion approach,single domain generalization challenges model generalizability,single-source domain generalization,unseen target
AI Read Science
Must-Reading Tree
Example
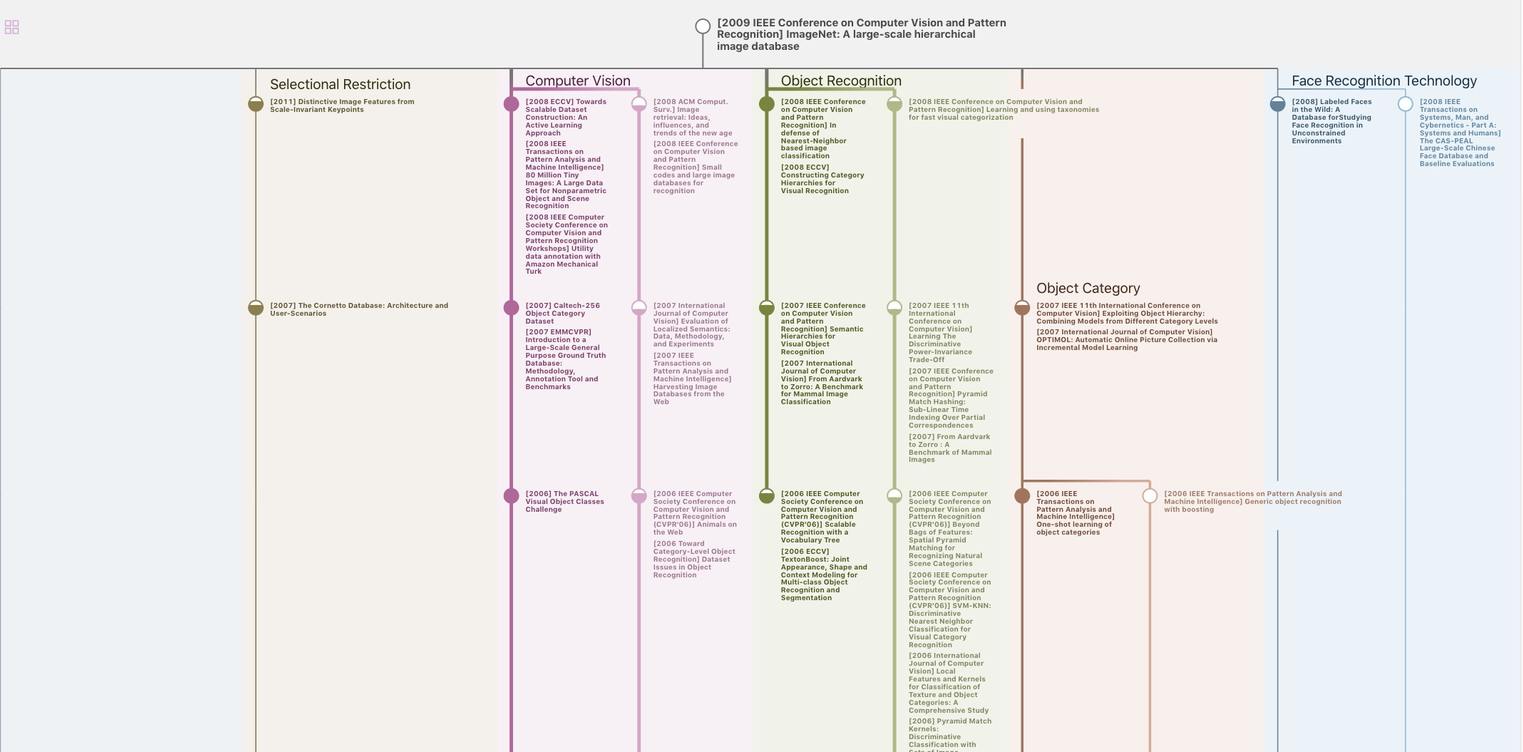
Generate MRT to find the research sequence of this paper
Chat Paper
Summary is being generated by the instructions you defined