Scene Graph Driven Text-Prompt Generation for Image Inpainting
CVPR Workshops(2023)
摘要
Scene editing methods are undergoing a revolution, driven by text-to-image synthesis methods. Applications in media content generation have benefited from a careful set of engineered text prompts, that have been arrived at by the artists by trial and error. There is a growing need to better model prompt generation, for it to be useful for a broad range of consumer-grade applications. We propose a novel method for text prompt generation for the explicit purpose of consumer-grade image inpainting, i.e. insertion of new objects into missing regions in an image. Our approach leverages existing inter-object relationships to generate plausible textual descriptions for the missing object, that can then be used with any text-to-image generator. Given an image and a location where a new object is to be inserted, our approach first converts the given image to an intermediate scene graph. Then, we use graph convolutional networks to 'expand' the scene graph by predicting the identity and relationships of the new object to be inserted, with respect to the existing objects in the scene. The output of the expanded scene graph is cast into a textual description, which is then processed by a text-to-image generator, conditioned on the given image, to produce the final inpainted image. We conduct extensive experiments on the Visual Genome dataset, and show through qualitative and quantitative metrics that our method is superior to other methods.
更多查看译文
关键词
consumer-grade applications,consumer-grade image inpainting,engineered text prompts,existing objects,expanded scene graph,final inpainted image,graph convolutional networks,inter-object relationships,intermediate scene graph,media content generation,missing object,model prompt generation,scene editing methods,scene graph driven text-prompt generation,text prompt generation,text-to-image generator,text-to-image synthesis methods
AI 理解论文
溯源树
样例
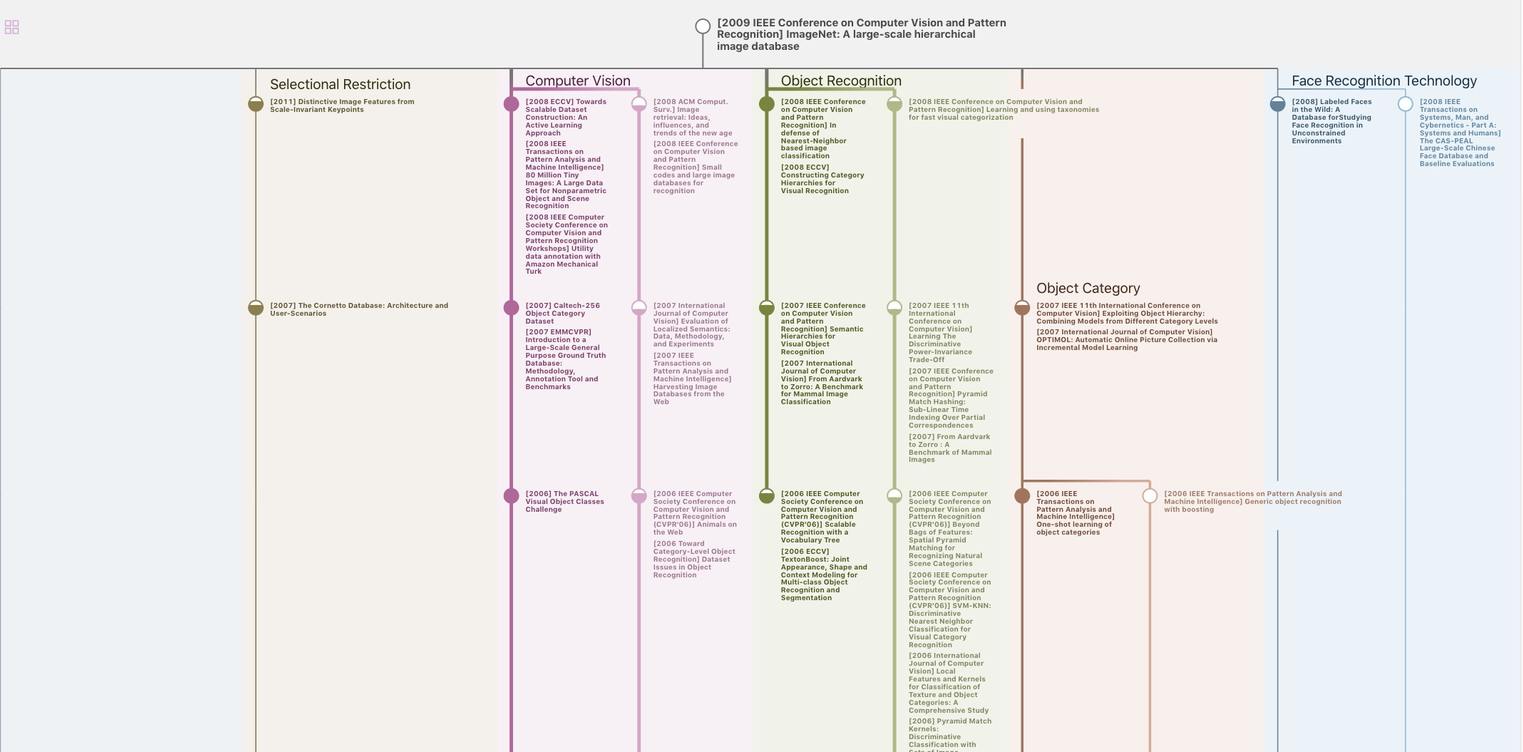
生成溯源树,研究论文发展脉络
Chat Paper
正在生成论文摘要