Rip Current Segmentation: A Novel Benchmark and YOLOv8 Baseline Results.
CVPR Workshops(2023)
摘要
Rip currents are the leading cause of fatal accidents and injuries on many beaches worldwide, emphasizing the importance of automatically detecting these hazardous surface water currents. In this paper, we address a novel task: rip current instance segmentation. We introduce a comprehensive dataset containing 2,466 images with newly created polygonal annotations for instance segmentation, used for training and validation. Additionally, we present a novel dataset comprising 17 drone videos (comprising about 24K frames) captured at 30 FPS, annotated with both polygons for instance segmentation and bounding boxes for object detection, employed for testing purposes. We train various versions of YOLOv8 for instance segmentation on static images and assess their performance on the test dataset (videos). The best results were achieved by the YOLOv8-nano model (runnable on a portable device), with an mAP50 of 88.94% on the validation dataset and 81.21% macro average on the test dataset. The results provide a baseline for future research in rip current segmentation. Our work contributes to the existing literature by introducing a detailed, annotated dataset, and training a deep learning model for instance segmentation of rip currents. The code, training details and the annotated dataset are made publicly available at https://github.com/Irikos/rip_currents.
更多查看译文
关键词
annotated dataset,comprehensive dataset,deep learning model,detailed dataset,drone videos,fatal accidents,hazardous surface water currents,injuries,instance segmentation,macro average,mAP50,newly created polygonal annotations,rip current segmentation,rip currents,test dataset,validation dataset,YOLOv8 baseline results,YOLOv8-nanomodel
AI 理解论文
溯源树
样例
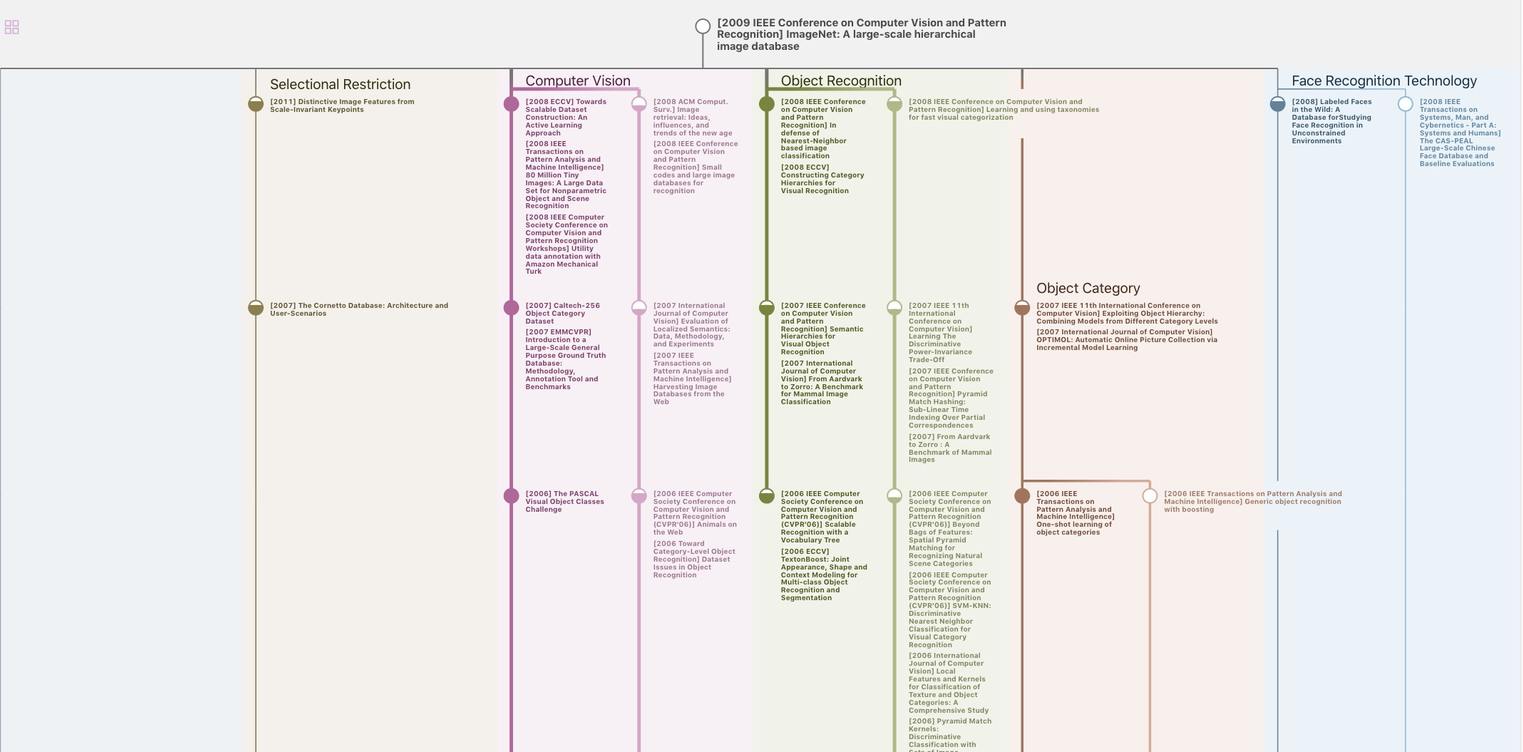
生成溯源树,研究论文发展脉络
Chat Paper
正在生成论文摘要