STAR: Sparse Thresholded Activation under partial-Regularization for Activation Sparsity Exploration.
CVPR Workshops(2023)
摘要
Brain-inspired event-driven processors execute deep neural networks (DNNs) in a sparsity-aware manner. Specifically, if more zeros are induced in the activation maps, less computation will be performed in the succeeding convolution layer. However, inducing activation sparsity in DNNs remains a challenge. To address this, we propose a training approach STAR (Sparse Thresholded Activation under partial-Regularization), which combines activation regularization with thresholding, to overcome the barrier of a single threshold- or regularization-based method in sparsity improvement. More precisely, we employ the sparse penalty on the near-zero activations to fit the activation learning behaviour in accuracy recovery, followed by thresholding to further suppress activations. Experimental results with SOTA networks (ResNet50/MobileNetV2, SSD, YOLOX and DeepLabV3+) on various datasets (Cifar-100, ImageNet, KITTI, VOC2007 and CityScapes) show that STAR can reduce on average 54% more activations compared to ReLU suppression. It outperforms the state-of-the-art by a significant margin of 35% in activation suppression without compromising accuracy loss. Additionally, a case study for a commercially-available event-driven hardware architecture, Neuronflow [29], demonstrates that the boosted activation sparsity in ResNet50 can be efficiently translated into latency reduction by up to 2.78×, FPS improvement by up to 2.80x, and energy savings by up to 2.09x. STAR elevates event-driven processors as a superior alternative to GPUs for Edge computing.
更多查看译文
关键词
activation learning behaviour,activation mapping,activation sparsity exploration,activation suppression,brain-inspired event-driven processors,Cifar-100 datasets,CityScapes datasets,deep neural networks,DeepLabV3+ networks,DNN,edge computing,event-driven hardware architecture,GPU,ImageNet datasets,inducing activation sparsity regularization,KITTI datasets,near-zero activations,Neuronflow,ReLU suppression,ResNet50-MobileNetV2 networks,SOTA networks,sparse penalty,sparse thresholded activation,sparse thresholded activation under partial-regularization-based method,sparsity-aware manner,SSD networks,training approach STAR,VOC2007 datasets,YOLOX networks
AI 理解论文
溯源树
样例
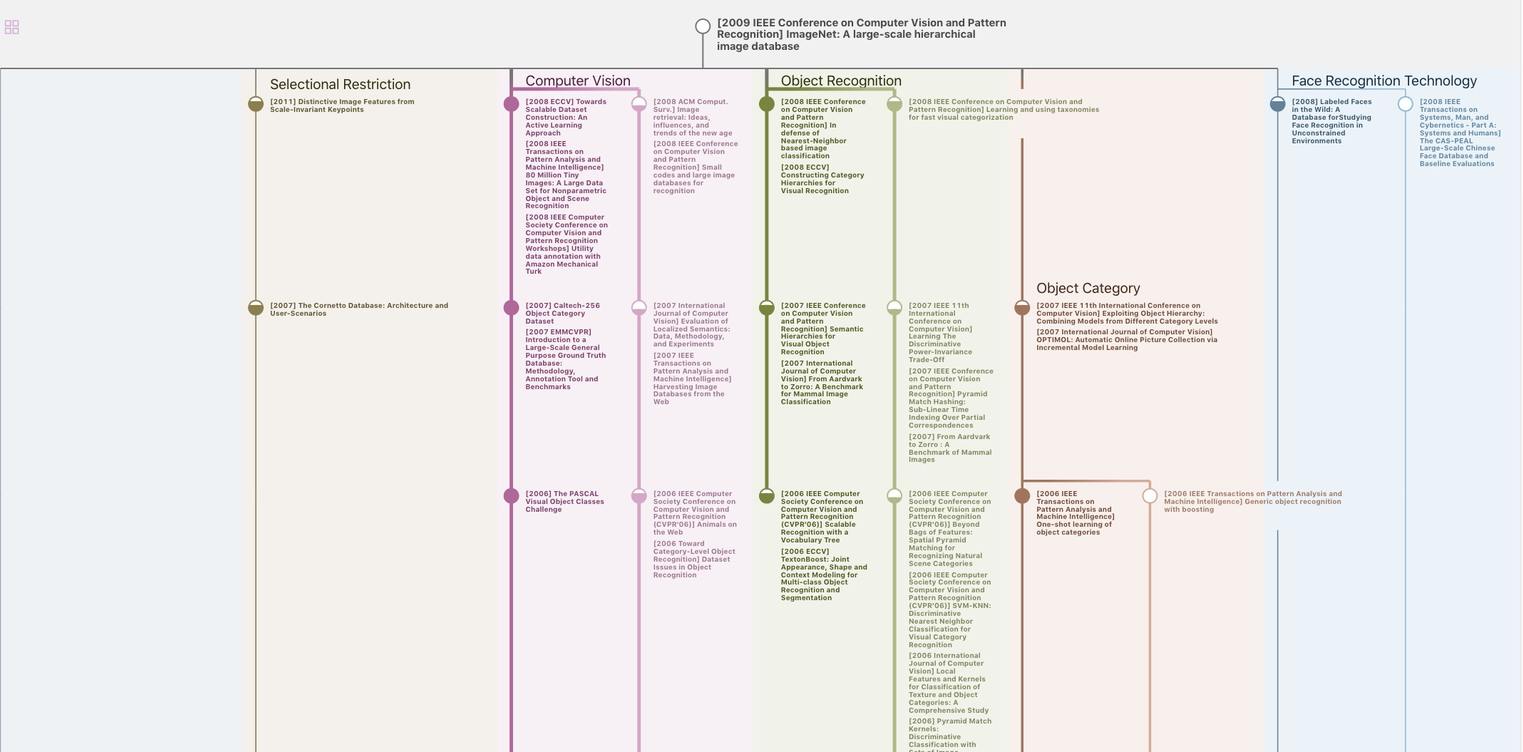
生成溯源树,研究论文发展脉络
Chat Paper
正在生成论文摘要