Multi-agent Decision-making at Unsignalized Intersections with Reinforcement Learning from Demonstrations.
IV(2023)
Abstract
Intersections are key nodes and also bottlenecks of urban road networks, so improving the traffic efficiency at intersections is beneficial to improving overall traffic throughput and mitigating traffic congestion. Previous methods such as rule-based, planning-based, and single-agent reinforcement learning usually oversimplify the policies of the surrounding vehicles and thus have difficulty modeling the complex interaction behaviors between vehicles, which limits the performance of these methods to some extent. Instead, we adopt a multi-agent reinforcement learning (MARL) approach to train and coordinate the policies of all vehicles to handle unsignalized intersection scenarios. Nevertheless, due to complex interactions between multiple agents, it is challenging to efficiently explore the environment and obtain high-reward samples. We therefore propose to pre-train the policy using demonstration data consisting of expert data and interaction data to improve the initial performance of agents and improve exploration, as well as to reduce the distributional shift between the demonstration data and the environmental interaction data. We experimentally prove that using interaction data generated by the algorithm in the demonstration data improves training stability. The proposed method enables effective exploration and greatly speeds up the training process.
MoreTranslated text
Key words
complex interaction,demonstration data,environmental interaction data,expert data,high-reward samples,initial performance,key nodes,mitigating traffic congestion,multiagent decision-making,multiagent reinforcement learning,multiple agents,single-agent reinforcement,traffic efficiency,traffic throughput,training process,training stability,unsignalized intersection scenarios,unsignalized intersections,urban road networks
AI Read Science
Must-Reading Tree
Example
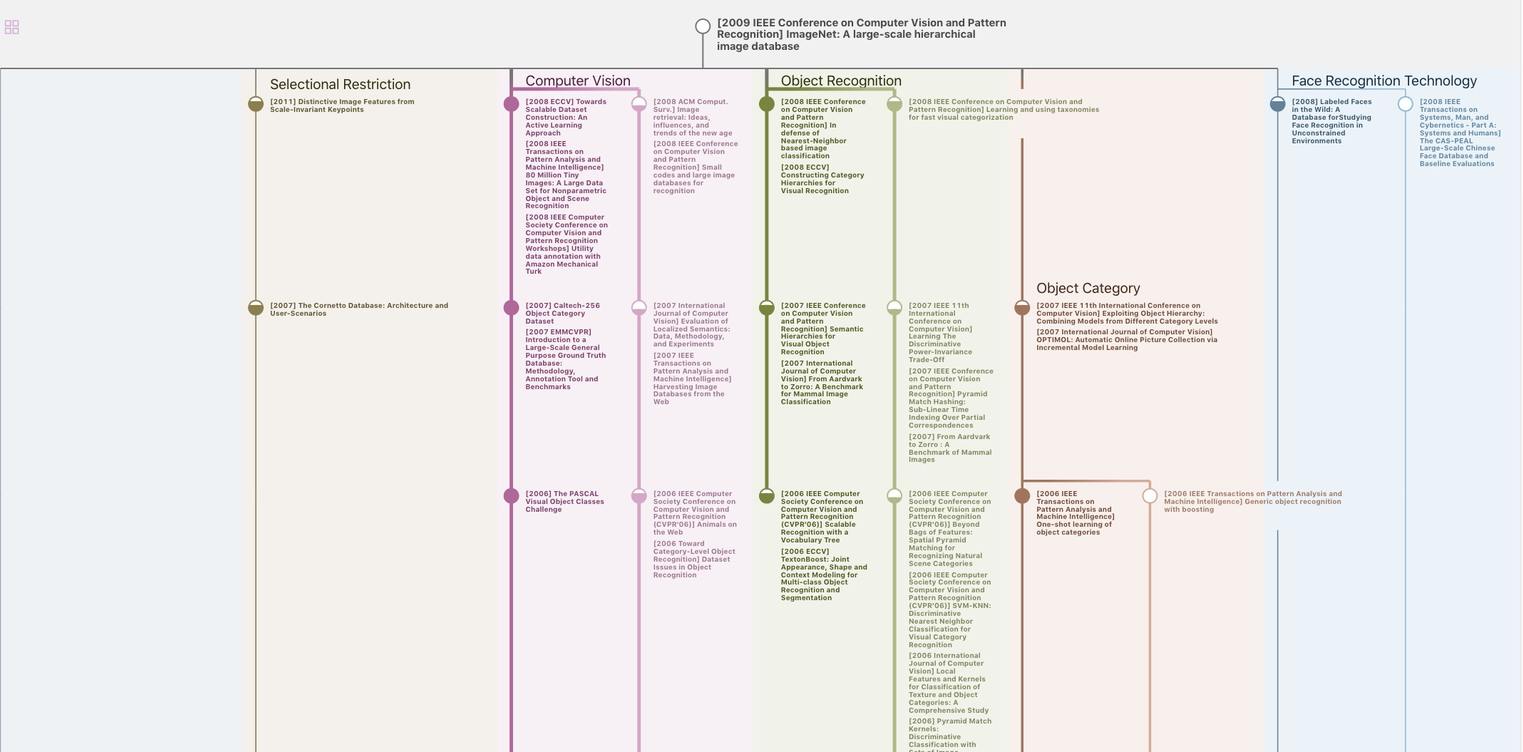
Generate MRT to find the research sequence of this paper
Chat Paper
Summary is being generated by the instructions you defined