Hardware-Aware Latency Pruning for Real-Time 3D Object Detection.
IV(2023)
摘要
3D Object detection is a fundamental task in vision-based autonomous driving. Deep learning perception models achieve an outstanding performance at the expense of continuously increasing resource needs and, as such, increasing training costs. As inference time is still a priority, developers usually adopt a training pipeline where they first start using a compact architecture that yields a good trade-off between accuracy and latency. This architecture is usually found either by searching manually or by using neural architecture search approaches. Then, train the model and use light optimization techniques such as quantization to boost the model's performance. In contrast, in this paper, we advocate for starting on a much larger model and then applying aggressive optimization to adapt the model to the resource-constraints. Our results on large-scale settings for 3D object detection demonstrate the benefits of initially focusing on maximizing the model's accuracy and then achieving the latency requirements using network pruning.
更多查看译文
关键词
compact architecture,deep learning perception models,hardware-aware latency pruning,inference time,larger model,latency requirements,light optimization techniques,neural architecture search approaches,real-time 3D Object detection,training costs,training pipeline,vision-based autonomous driving
AI 理解论文
溯源树
样例
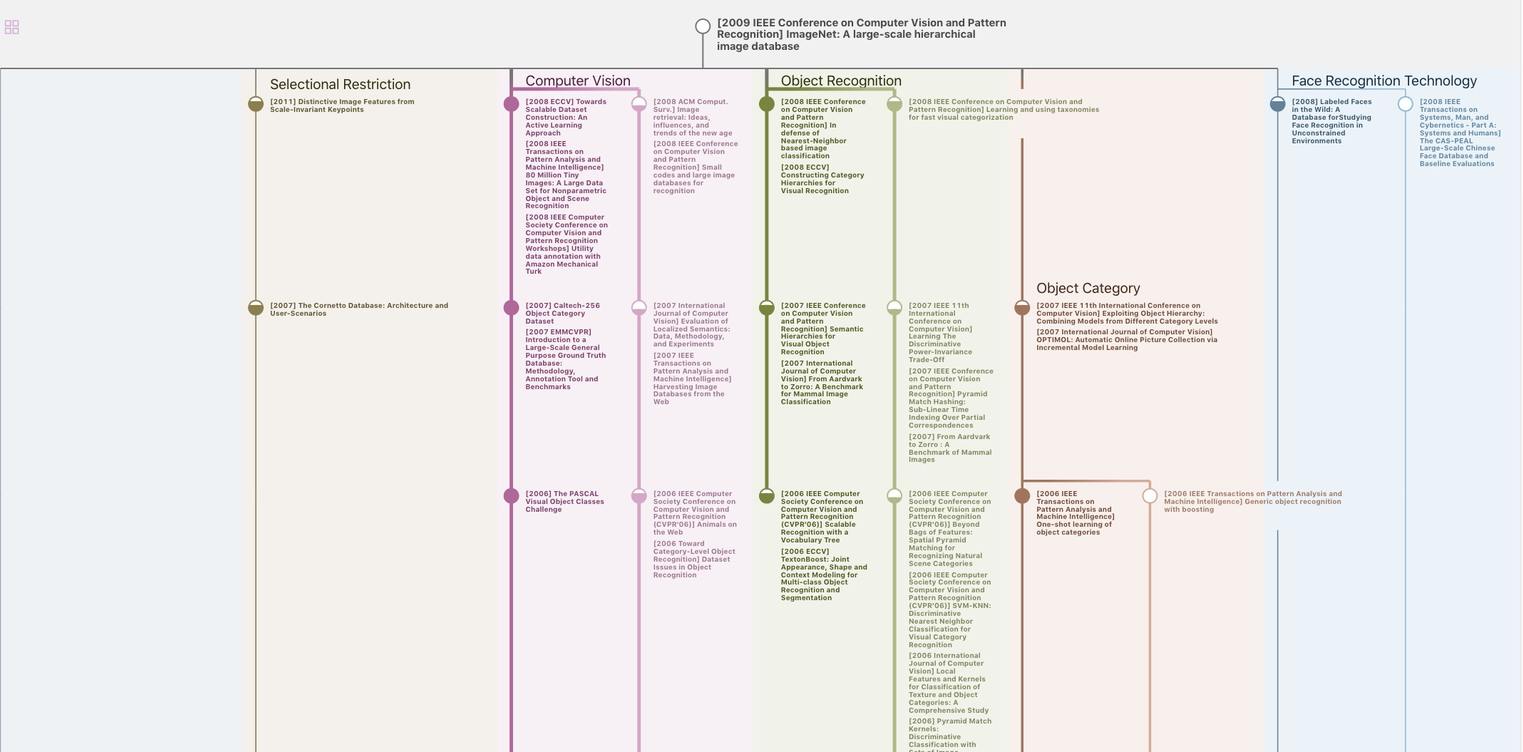
生成溯源树,研究论文发展脉络
Chat Paper
正在生成论文摘要