Using differential equation inspired machine learning for valve faults prediction.
INDIN(2023)
摘要
In an industrial plant it is necessary to monitor the operation of the equipment. Deviations from normal operation should be detected as early as possible to avoid production failures or to keep their impact as small as possible. Valves are core components of plants and critical damage can occur if they are set to switch but in reality do not switch. Therefore, plant operators would like to detect such failures as early as possible. Valves with electrodynamic actuators offer a unique diagnostics option that allows for the early detection of valve malfunctions by their intrinsic properties alone. In particular, we measure the inrush current of the valve as a function of time. From the resulting signal, we derive the information, if the valve has switched or not. Machine learning (ML) methods can be used to accurately predict these valve errors. In this paper, a long-short term memory (LSTM)-convolutional neural network (CNN) and two differential equation-inspired neural networks are presented for encoding valves function anomalies to ensure the production capability of an industrial plant. For this purpose, derivatives of the inrush currents are considered and integrated into the deep neural networks (DNNs). The implementation of this diagnostics option is cheap and robust as no extra sensor on the valve is required.
更多查看译文
关键词
machine learning, diagnostics, solenoid valve, electrodynamic valve
AI 理解论文
溯源树
样例
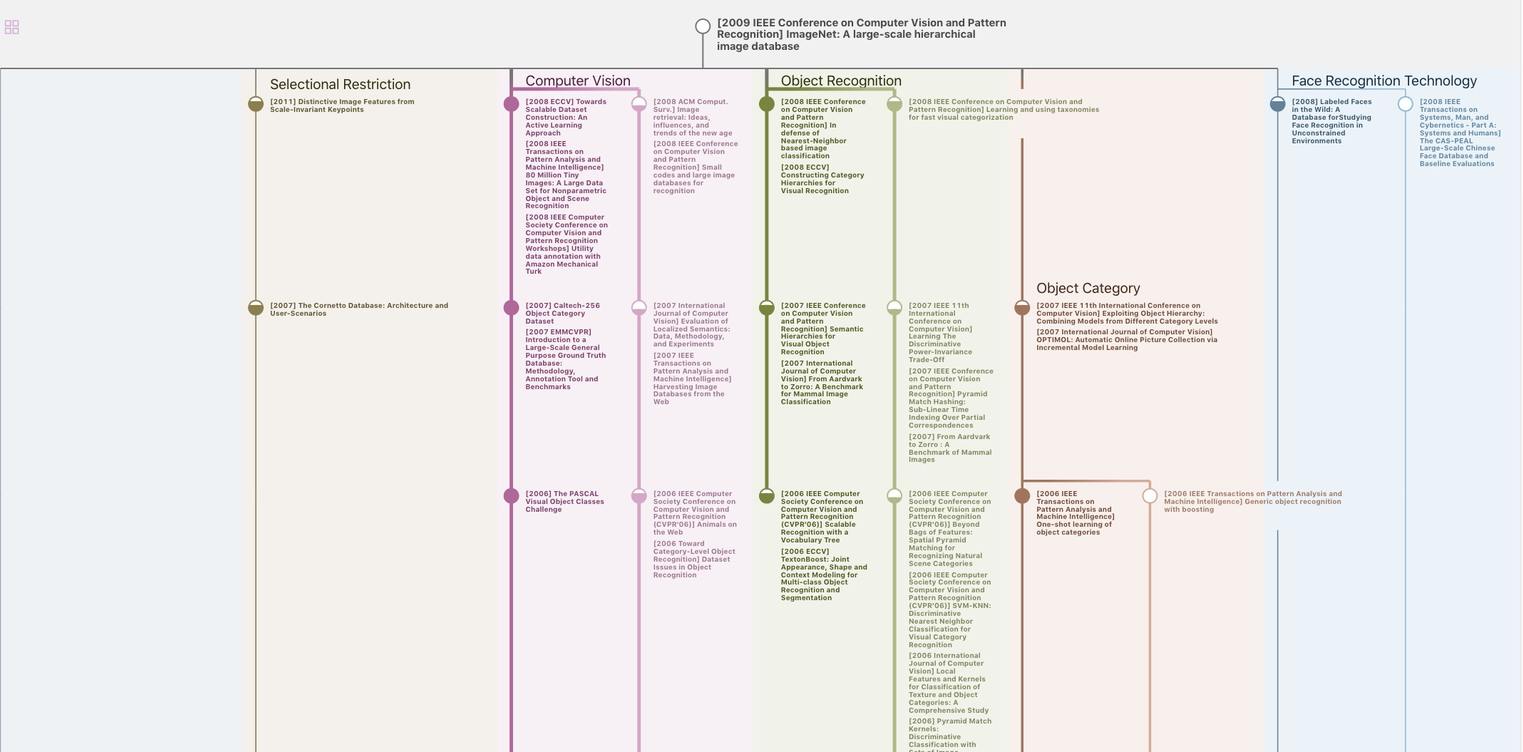
生成溯源树,研究论文发展脉络
Chat Paper
正在生成论文摘要