A Comparison of Demographic Attributes Detection from Handwriting Based on Traditional and Deep Learning Methods.
ICDAR Workshops (2)(2023)
摘要
Analyzing handwritten documents and detecting demographic attributes of writers from handwritten samples has received enormous attention from various fields of research, including psychology, computer science and artificial intelligence. Automatic detection of age, gender, handedness, nationality, and qualification of writers based on handwritten documents has several real-world applications, such as forensics and psychology. This paper proposes two simple but effective methods to detect the demographic information of writers from offline handwritten document images. The proposed methods are based on traditional and deep learning approaches. In the traditional machine learning method, the Rank Transform feature extraction method is used for measuring the intensity in handwriting images. The extracted handcrafted features are then fed into Support Vector Machine based classifiers to predict the demographical attributes of writers. In the deep learning method, a Convolutional Neural Network model based on the ResNet architecture with a fully connected layer, followed by a softmax layer is used to provide probability scores to facilitate demographic information detection. To evaluate the proposed methods and compare the results with the results in the literature, a comprehensive set of experiments was conducted on a frequently used benchmark database, KHATT. Both methods performed relatively well in predicting different demographic attributes. However, considering the settings in our experiments, the results obtained from the traditional model indicated better demographic detection compared to the deep learning models in all the tasks.
更多查看译文
关键词
demographic attributes detection,handwriting,deep learning
AI 理解论文
溯源树
样例
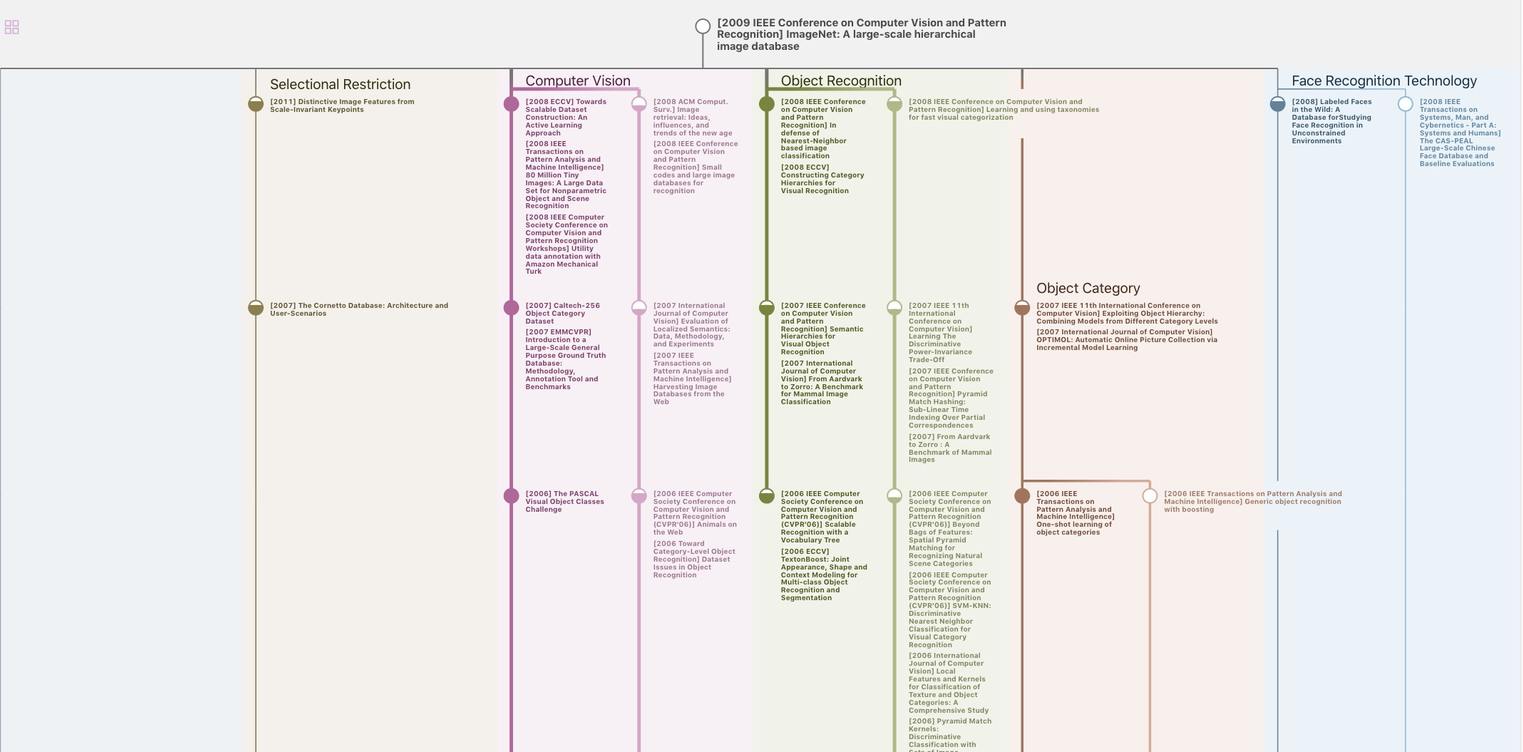
生成溯源树,研究论文发展脉络
Chat Paper
正在生成论文摘要