Historical Document Image Segmentation Combining Deep Learning and Gabor Features.
ICDAR (4)(2023)
摘要
Due to the idiosyncrasies of historical document images (HDI), growing attention over the last decades is being paid for proposing robust HDI analysis solutions. Many research studies have shown that Gabor filters are among the low-level descriptors that best characterize texture information in HDI. On the other side, deep neural networks (DNN) have been successfully used for HDI segmentation. As a consequence, we propose in this paper a HDI segmentation method that is based on combining Gabor features and DNN. The segmentation method focuses on classifying each document image pixel to either graphic, text or background. The novelty of the proposed method lies mainly in feeding a DNN with a Gabor filtered image (obtained by applying specific multichannel Gabor filters) instead of an original image as input. The proposed method is decomposed into three steps: a) filtered image generation using Gabor filters, b) feature learning with stacked autoencoder, and c) image segmentation with 2D U-Net. In order to evaluate its performance, experiments are conducted using two different datasets. The results are reported and compared with those of a recent state-of-the-art method.
更多查看译文
关键词
deep learning,image,features
AI 理解论文
溯源树
样例
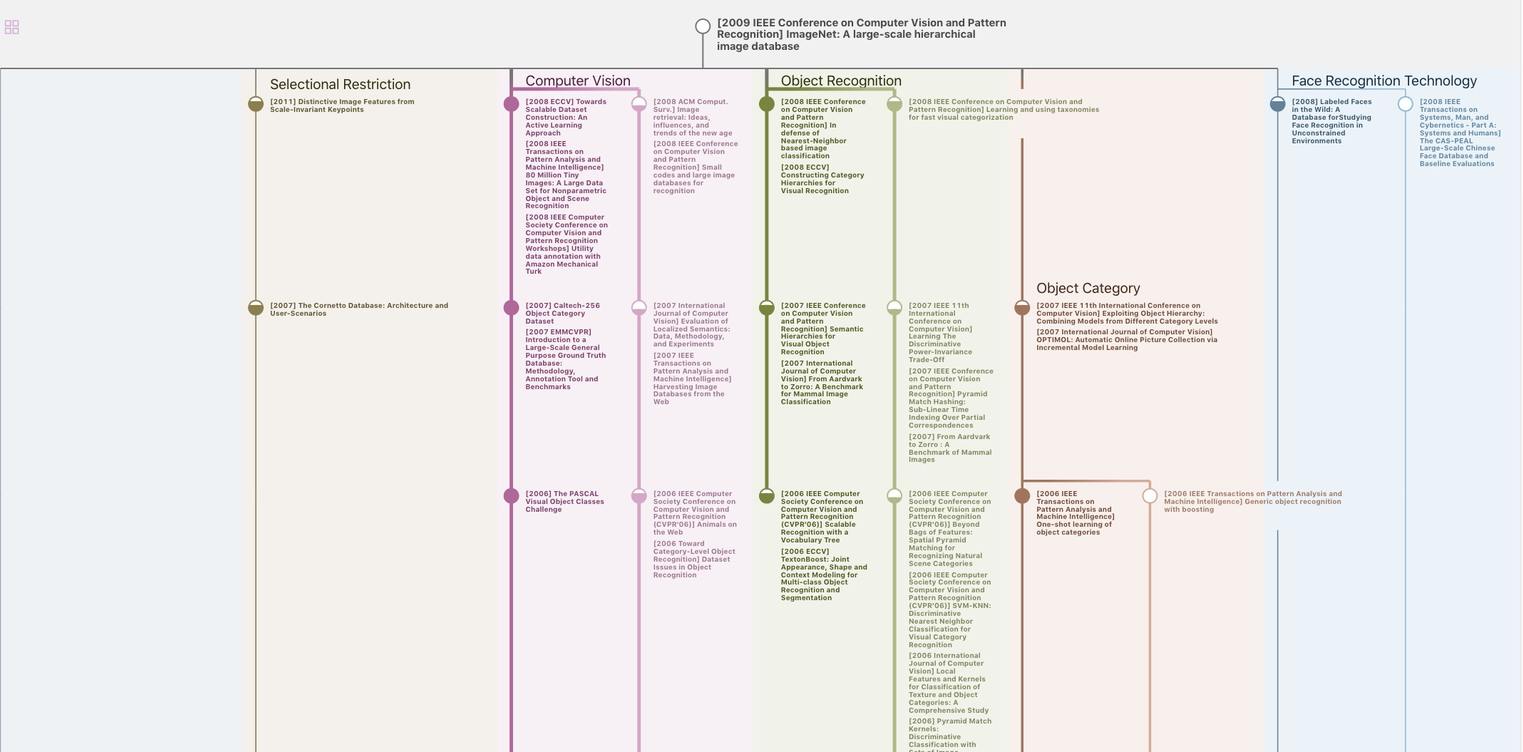
生成溯源树,研究论文发展脉络
Chat Paper
正在生成论文摘要