MPNet: Multiscale predictions based on feature pyramid network for semantic segmentation.
ICUFN(2023)
摘要
Semantic segmentation is a complex topic where they assign each pixel of an image with a corresponding class and demand accuracy at objective boundaries. The method plays a vital role in scene-understanding scenarios. For self-driving applications, the input source includes various types of objects such as trucks, people, or traffic signs. One receptive field is only effective in capturing a short range of sizes. Feature pyramid network (FPN) utilizes different fields of view to extract information from the input. The FPN approach obtains the spatial information from the high-resolution feature map and the semantic information from the lower scales. The final feature representation contains coarse and fine details, but it has some drawbacks. They burden the system with extensive computation and reduce the semantic information. In this paper, we devise an effective multiscale predictions network (MPNet) to address these issues. A multiscale pyramid of predictions effectively processes the prominent characteristics of each feature. A pair of adjacent features is combined together to predict the output separately. A lower-scale feature of each prediction is assigned as the contextual contributor, and the other provides coarser information. The contextual branch is passed through the atrous spatial pyramid pooling to improve performance. The segmentation scores are fused to obtain advantages from all predictions. The model is validated by a series of experiments on open data sets. We have achieved good results 76.5% mIoU at 50 FPS on Cityscapes and 43.9% mIoU on Mapillary Vistas.
更多查看译文
关键词
Semantic segmentation,feature pyramid network,multiscale prediction,real-time application
AI 理解论文
溯源树
样例
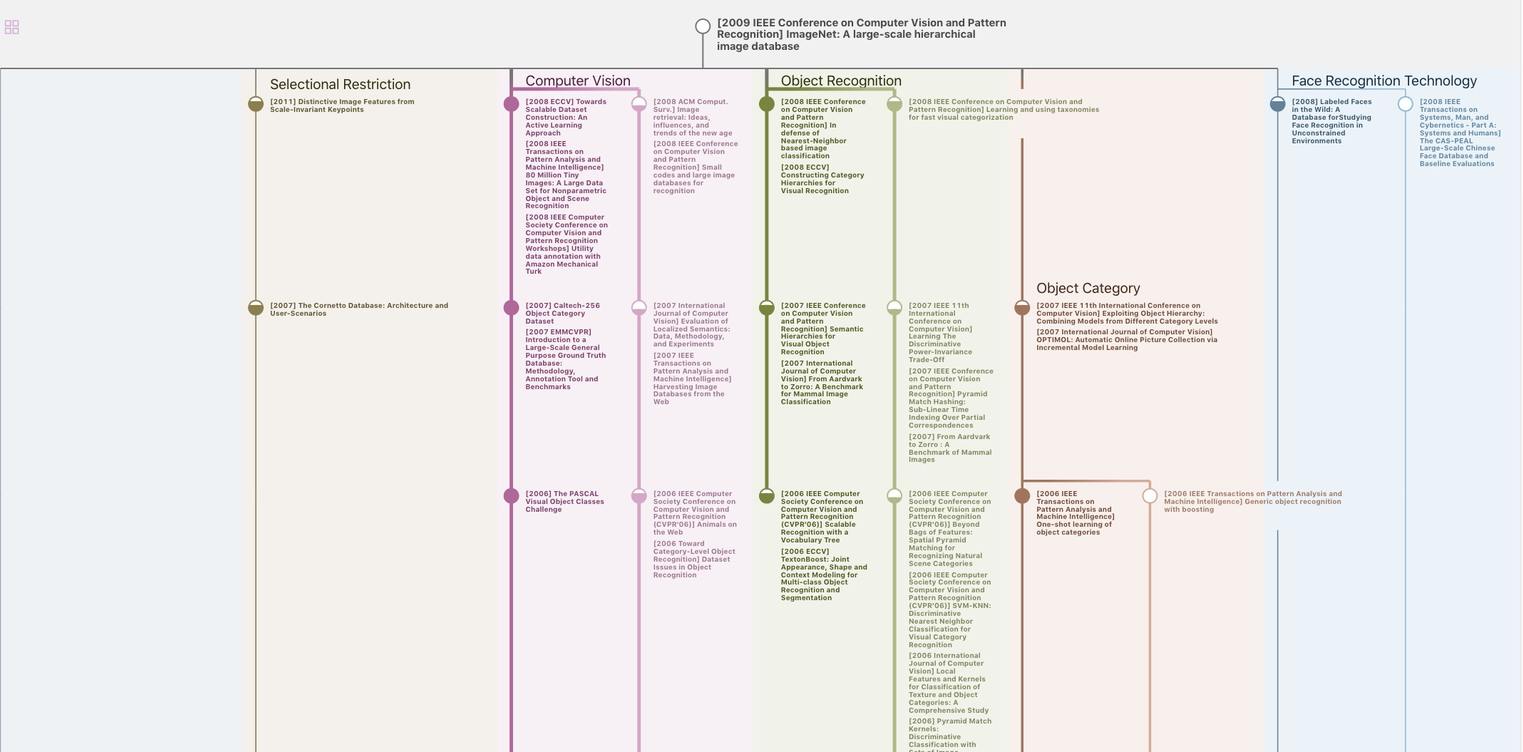
生成溯源树,研究论文发展脉络
Chat Paper
正在生成论文摘要