NExtGCN: Modeling Node Importance of Graph Convolution Network by Neighbor Excitation for Recommendation.
DEXA (2)(2023)
摘要
To alleviate information overload, the recommender system is pushing personalized contents to users and improving the efficiency of information distribution. Graph Convolution Networks (GCNs), which can better gather structured information and becomes a new state-of-the-art for collaborative filtering. Many current works on GCNs tend to be easier to train and have better generalization ability like LightGCN. However, they care less about the importance of nodes. In this work, we propose a new model named NExtGCN (Neighbor Exci tation Graph Convolutional Network), which models the node importance of GCN by neighbor excitation. The NExtGCN can learn the importance of nodes via the global and local excitation layer which is inspired by the Squeeze-Excitation network. Furthermore, we propose a neighbor excitation layer that can fully utilize graph structure and make this model practical to large-scale datasets. Extensive experimental results on four real-world datasets have shown the effectiveness and robustness of the proposed model. Especially on the Amazon-Books dataset, our NExtGCN has improved by 10.95%, 49.36%, and 26.8% in Recall@20, MRR@20, and NDCG@20 compared to LightGCN. We also provide source code ( https://github.com/clemaph/NExtGCN.git ) to reproduce the experimental results (This job is supported by Postgraduate Research & Practice Innovation Program of Jiangsu Province, the item number is KYCX22_3071).
更多查看译文
关键词
node importance,graph convolution network,neighbor excitation,nextgcn
AI 理解论文
溯源树
样例
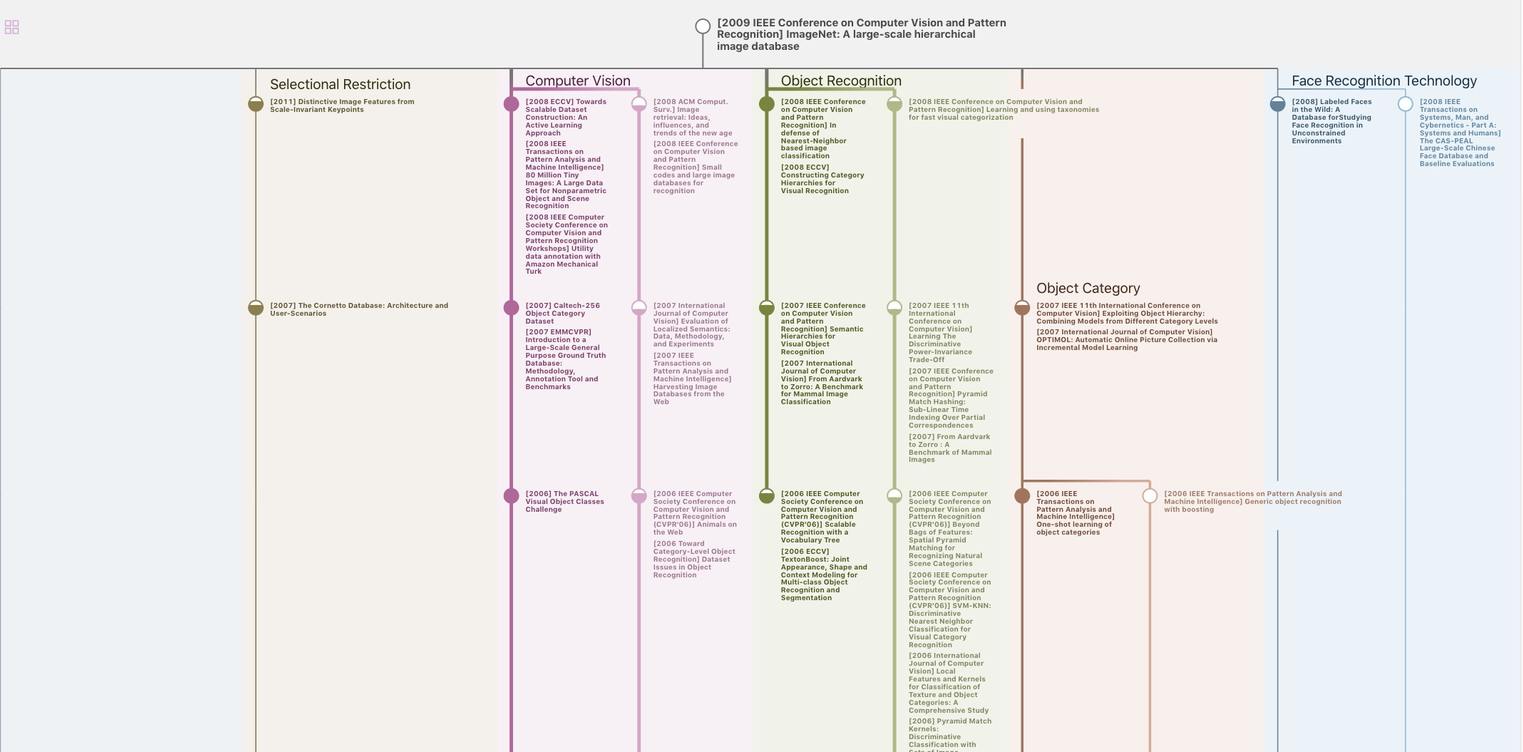
生成溯源树,研究论文发展脉络
Chat Paper
正在生成论文摘要