Adaptive weighted ensemble classifier for improving breast tumors classification based on ultrasound RF data.
AI2A(2023)
摘要
In the classification of benign and malignant breast tumors, in addition to the extraction of features, the selection of classifier is also an important factor affecting the accuracy of classification. At present, the ensemble classifier has limitations of local optimization and weak scalability in the integration process, which affect the accuracy and stability of classification. In this study, an ensemble classifier based on adaptive weighted ensemble is proposed to classify benign and malignant breast tumors. First, the original ultrasonic RF signal is preprocessed by down-sampling, dilation, and adaptive decomposition. Then, based on the intrinsic modal function obtained by decomposition, the ring region of interest (ROI) containing the tissue surrounding the tumor is determined. Next, texture features are extracted based on ring ROIs. Weighted k-nearest neighbors (KNN), bagged trees, and Gaussian Naive Bayes (NB) are integrated by bagging method, and each classifier is adaptively weighted based on genetic algorithms during the integration process. Finally, benign and malignant breast tumors are classified by an ensemble classifier based on adaptive weights. Experiments based on the Open Access Series of Breast Ultrasound Data database show that the classification accuracy of the proposed adaptive weight-based ensemble classifier is 90%, which is improved by 10.77%, 8.43%, 1.12% and 5.88% compared with the weighted KNN, bagged trees and Gaussian NB alone, and voting-based ensemble classifier. The conclusion is that the proposed ensemble classifier based on adaptive weighting can effectively improve the classification accuracy of breast tumors, which is helpful for the diagnosis of clinical breast tumors.
更多查看译文
AI 理解论文
溯源树
样例
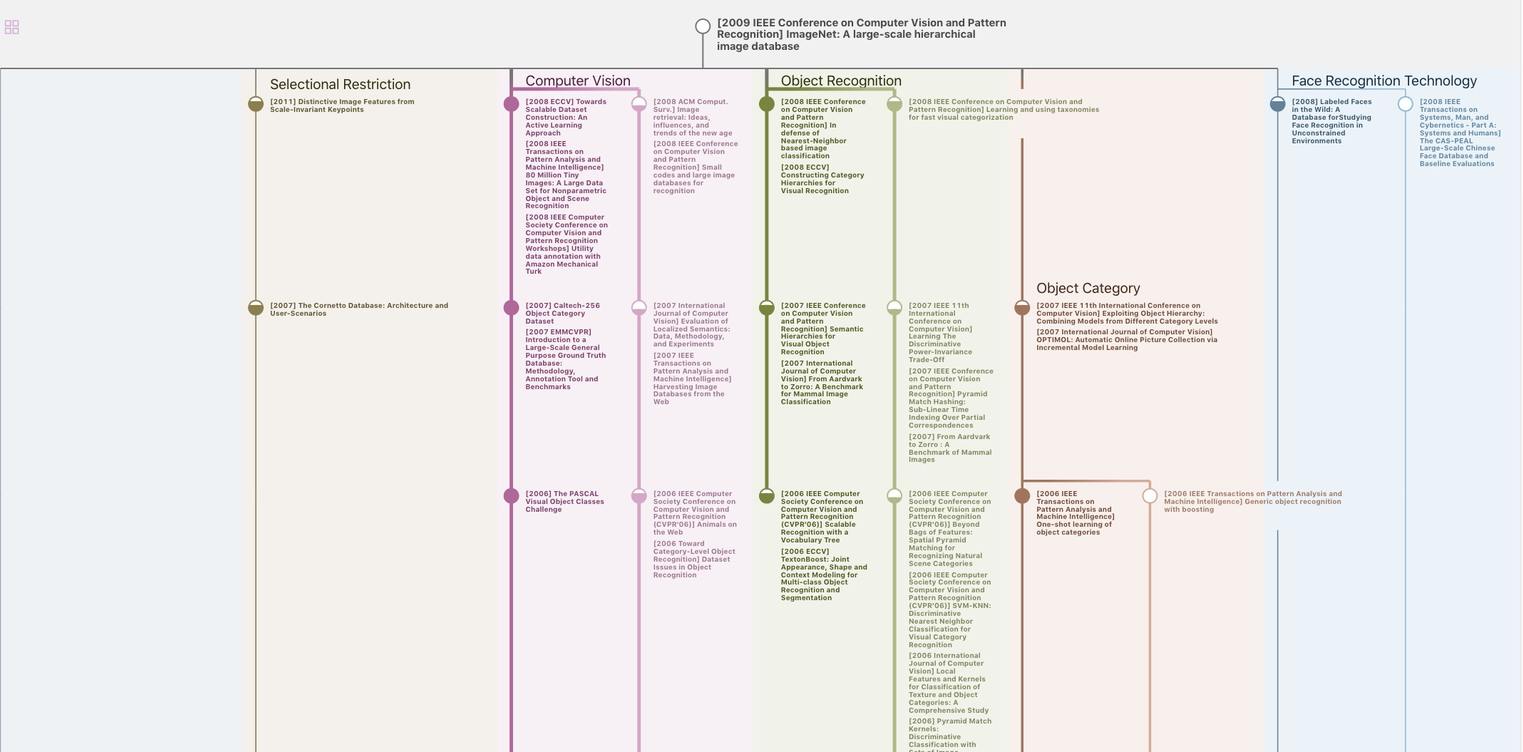
生成溯源树,研究论文发展脉络
Chat Paper
正在生成论文摘要