An Adaptive TinyML Unsupervised Online Learning Algorithm for Driver Behavior Analysis.
MetroAutomotive(2023)
摘要
The Internet of Things (IoT) has significantly impacted various industries, particularly the automotive sector, due to the growing integration of IoT technologies in vehicles. As a result, the volume of data generated by vehicular sensors has increased, leading to a surge in studies focusing on driver behavior to enhance road safety and optimize transportation networks. However, traditional approaches to analyzing driver behavior have relied on supervised offline learning models, which are unsuitable for handling data streams in online learning environments. This study introduces an unsupervised online k-fix AutoCloud algorithm for detecting driver behavior patterns, leveraging the concepts of typicity and eccentricity while considering the historical-temporal relationships between samples. Furthermore, the algorithm autonomously and adaptively evolves without requiring a supervised training phase, making it compatible with the TinyML concept, encompassing Artificial Intelligence algorithms designed for low-power IoT devices. To validate the proposed method, a real case study was conducted over four days using a vehicle to compare the quantity and quality of clusters generated by the algorithm. The findings demonstrate the potential of the proposed approach for optimizing data processing with minimal computational power.
更多查看译文
关键词
Internet of Things,driver behavior,unsupervised learning,k-fix AutoCloud algorithm,TinyML,online learning,road safety,data processing
AI 理解论文
溯源树
样例
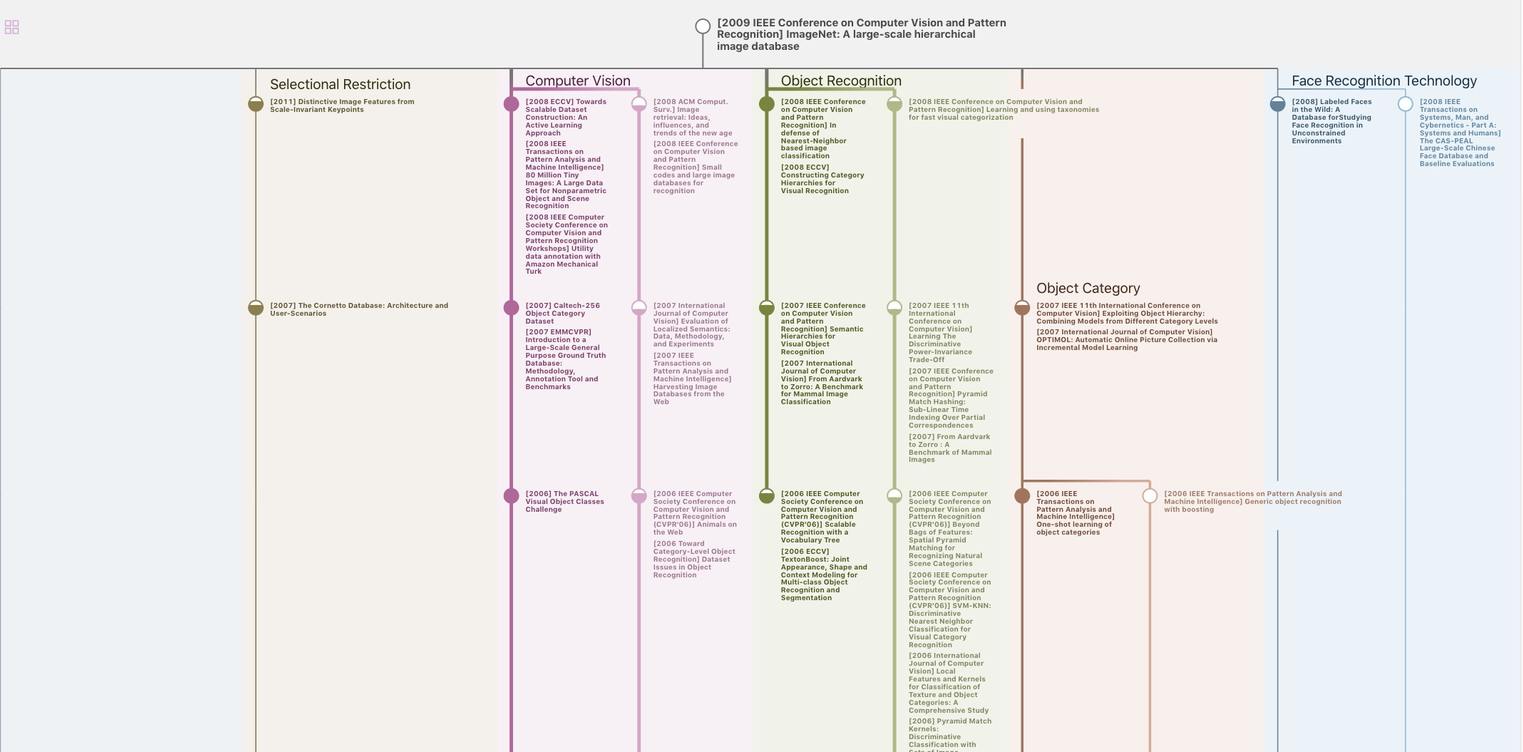
生成溯源树,研究论文发展脉络
Chat Paper
正在生成论文摘要