ParaSum: Contrastive Paraphrasing for Low-Resource Extractive Text Summarization.
KSEM (3)(2023)
摘要
Existing extractive summarization methods achieve state-of-the-art (SOTA) performance with pre-trained language models (PLMs) and sufficient training data. However, PLM-based methods are known to be data-hungry and often fail to deliver satisfactory results in low-resource scenarios. Constructing a high-quality summarization dataset with human-authored reference summaries is a prohibitively expensive task. To address these challenges, this paper proposes a novel paradigm for low-resource extractive summarization, called ParaSum. This paradigm reformulates text summarization as textual paraphrasing, aligning the text summarization task with the self-supervised Next Sentence Prediction (NSP) task of PLMs. This approach minimizes the training gap between the summarization model and PLMs, enabling a more effective probing of the knowledge encoded within PLMs and enhancing the summarization performance. Furthermore, to relax the requirement for large amounts of training data, we introduce a simple yet efficient model and align the training paradigm of summarization to textual paraphrasing to facilitate network-based transfer learning. Extensive experiments over two widely used benchmarks (i.e., CNN/DailyMail, Xsum) and a recent open-sourced high-quality Chinese benchmark (i.e., CNewSum) show that ParaSum consistently outperforms existing PLM-based summarization methods in all low-resource settings, demonstrating its effectiveness over different types of datasets.
更多查看译文
关键词
contrastive paraphrasing,text,low-resource
AI 理解论文
溯源树
样例
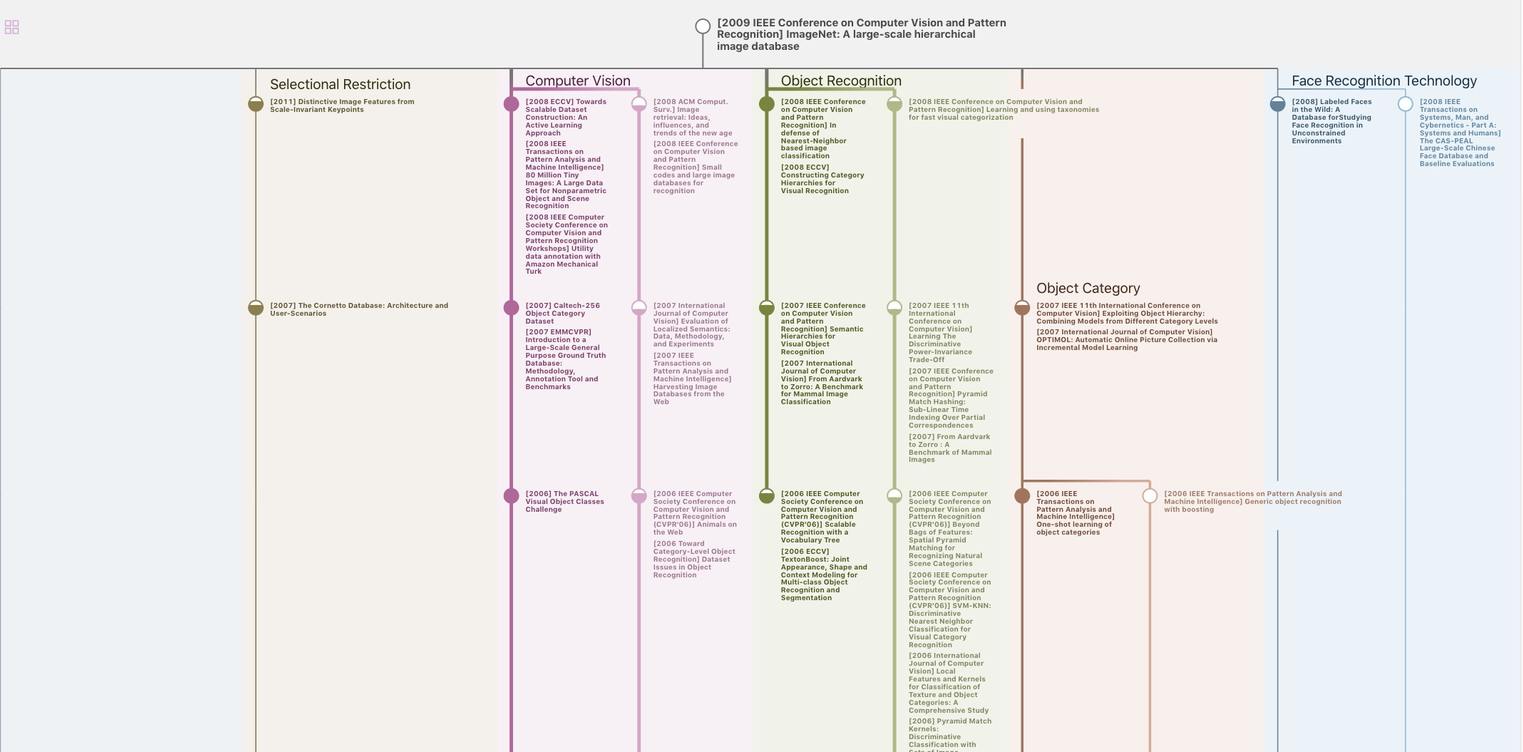
生成溯源树,研究论文发展脉络
Chat Paper
正在生成论文摘要