Exponential Lower Bounds for Threshold Circuits of Sub-Linear Depth and Energy.
MFCS(2023)
摘要
In this paper, we investigate computational power of threshold circuits and other theoretical models of neural networks in terms of the following four complexity measures: size (the number of gates), depth, weight and energy. Here the energy complexity of a circuit measures sparsity of their computation, and is defined as the maximum number of gates outputting non-zero values taken over all the input assignments. As our main result, we prove that any threshold circuit $C$ of size $s$, depth $d$, energy $e$ and weight $w$ satisfies $\log (rk(M_C)) \le ed (\log s + \log w + \log n)$, where $rk(M_C)$ is the rank of the communication matrix $M_C$ of a $2n$-variable Boolean function that $C$ computes. Thus, such a threshold circuit $C$ is able to compute only a Boolean function of which communication matrix has rank bounded by a product of logarithmic factors of $s,w$ and linear factors of $d,e$. This implies an exponential lower bound on the size of even sublinear-depth threshold circuit if energy and weight are sufficiently small. For other models of neural networks such as a discretized ReLE circuits and decretized sigmoid circuits, we prove that a similar inequality also holds for a discretized circuit $C$: $rk(M_C) = O(ed(\log s + \log w + \log n)^3)$.
更多查看译文
关键词
threshold circuits,sub-linear
AI 理解论文
溯源树
样例
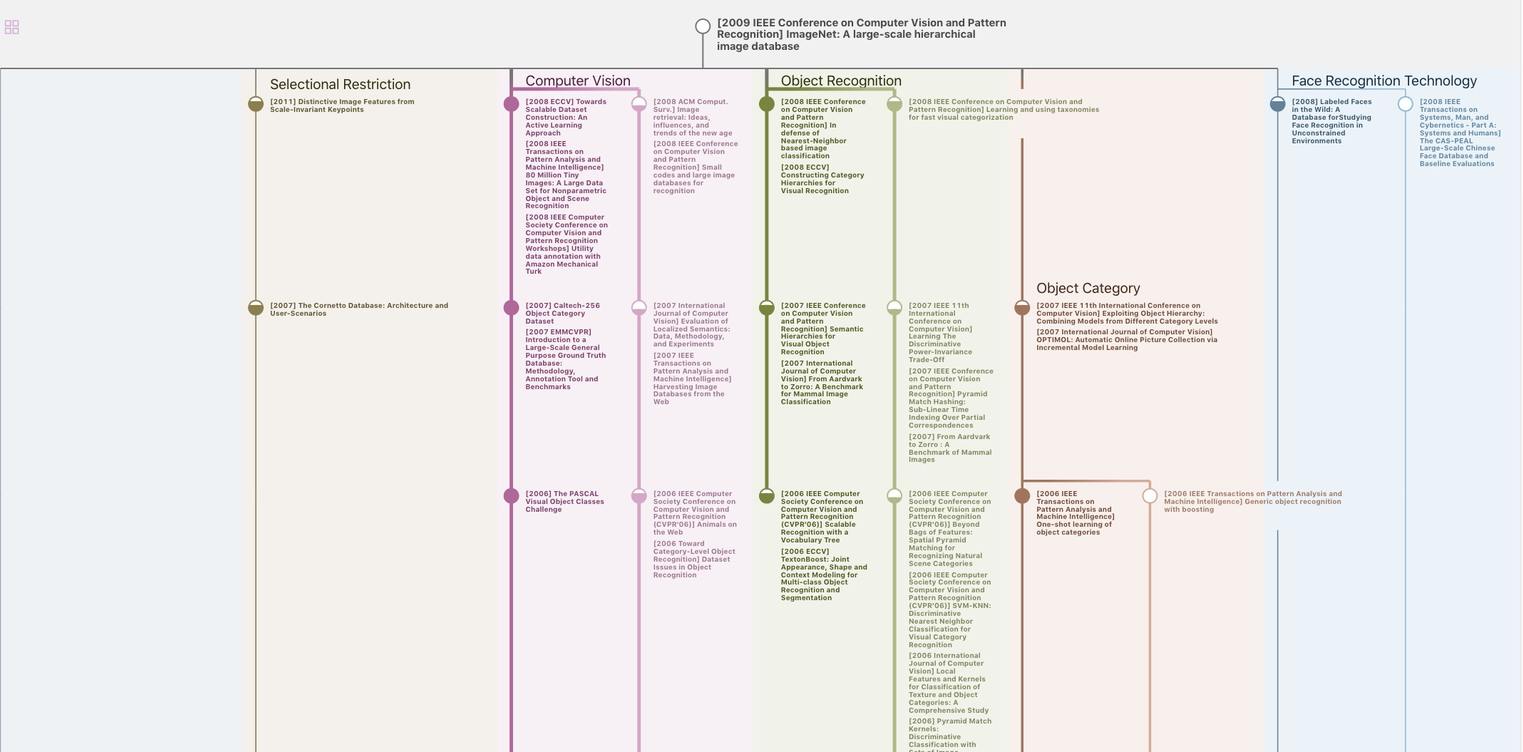
生成溯源树,研究论文发展脉络
Chat Paper
正在生成论文摘要