Pointwise Sliced Mutual Information for Neural Network Explainability
ISIT(2023)
摘要
When deploying deep learning models such as convolutional neural networks (CNNs) in safety-critical domains, it is important to understand the predictions made by these black-box models. While many different approaches have been taken to improve the interpretability of deep models, most methods lack theoretical properties, making it hard to justify the correctness of the explanations provided. In this paper, we take an information-theoretic approach to understand why CNNs make their predictions. Building upon sliced mutual information, we propose pointwise sliced mutual information (PSI) as a tool for measuring the amount of useful information that a feature has about the label for a single instance. We theoretically justify the use of PSI for explaining predictions made by CNNs through the connection with margin. We show that PSI works as an explainability tool in two ways: (i) Fiber-wise PSI constructs a saliency map that highlights regions in the image which are important in predicting the labels; (ii) Sample-wise PSI provides confidence scores for predictions on the labels.
更多查看译文
关键词
black-box models,CNNs,convolutional neural networks,deep models,deploying deep learning models,explainability tool,Fiber-wise PSI constructs,information-theoretic approach,neural network explainability,pointwise sliced mutual information,safety-critical domains
AI 理解论文
溯源树
样例
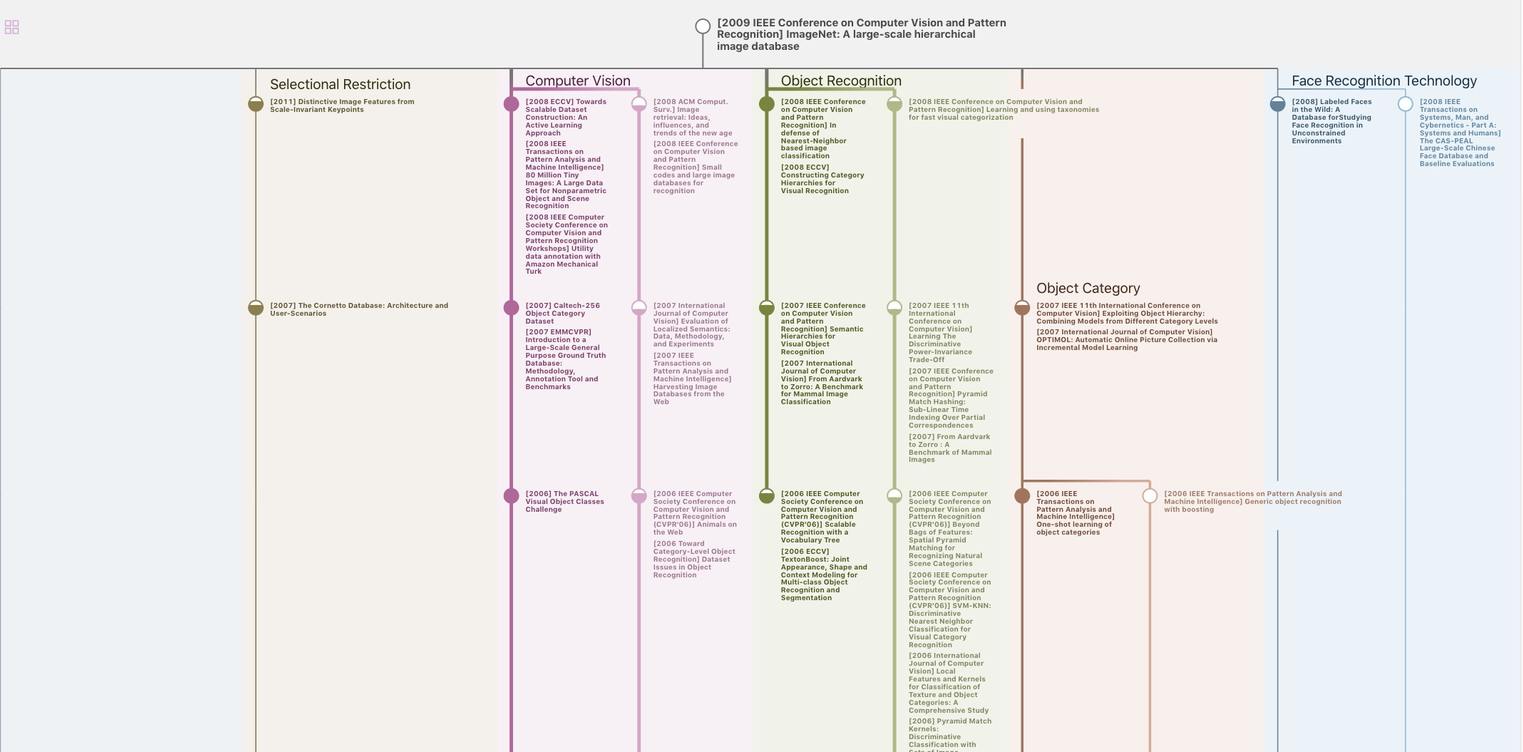
生成溯源树,研究论文发展脉络
Chat Paper
正在生成论文摘要