Sim-to-Real Policy and Reward Transfer with Adaptive Forward Dynamics Model.
ICRA(2023)
摘要
Deep reinforcement learning has shown promise in learning robust skills for robot control, but typically requires a large amount of samples to achieve good performance. Sim-to-real transfer learning has been developed to solve this problem, but the policy trained in simulation usually has unsatisfactory performance in the real world because simulators inevitably model the dynamics of reality imperfectly. To enable sample-efficient learning in the real world, we proposed progressive policy transfer with adaptive dynamics model (PPTADM). PPTADM assumes the dynamics of simulation and real world do not match but the state space is the same, transfers policy from simulation via progressive neural network (PNN) and further improves the policy with a learned forward dynamics model in reality. In addition, for real-world tasks in which reward functions are difficult or even impossible to define and verify the effectiveness, PPTADM can learn in real world solely from a transferred reward function that is estimated from simulation even though their dynamics do not match. Our results in five simulated tasks and on a real robot arm show that with PPTADM, the robot's learning efficiency and performance in the real world can be significantly improved.
更多查看译文
关键词
adaptive dynamics model,adaptive forward dynamics model,deep reinforcement learning,learned forward dynamics model,PPTADM,progressive neural network,progressive policy transfer,real-world tasks,reward functions,reward transfer,robot arm show,robot control,robust skills,sample-efficient learning,sim-to-,sim-to-real transfer learning,simulated tasks,transferred reward function,transfers policy,unsatisfactory performance
AI 理解论文
溯源树
样例
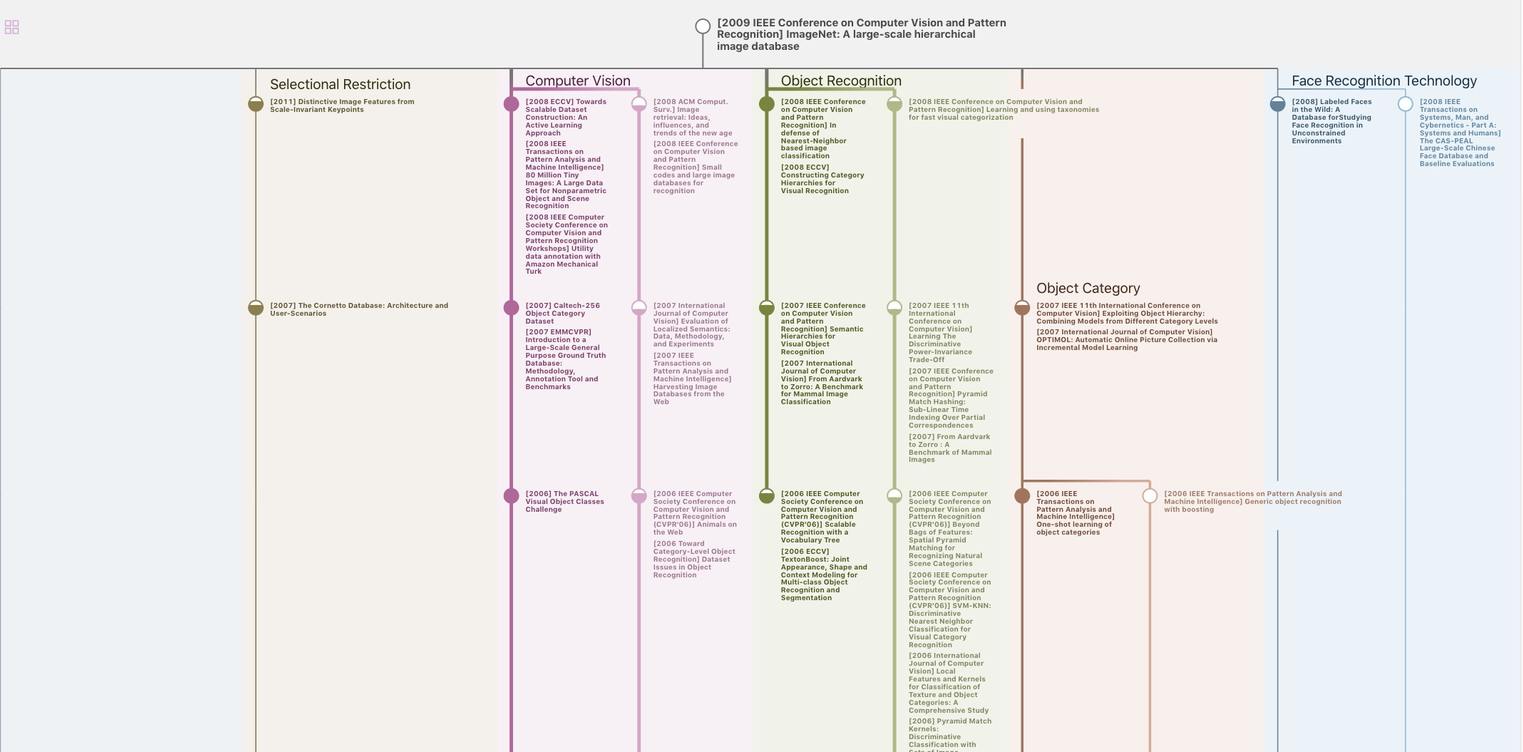
生成溯源树,研究论文发展脉络
Chat Paper
正在生成论文摘要