GNN-Based Point Cloud Maps Feature Extraction and Residual Feature Fusion for 3D Object Detection.
ICRA(2023)
摘要
LiDAR detection of long-range vehicles is challenging because very few and sparse points are measured in long distances and vehicles with similar shapes of targets could lead to false positives easily. To tackle these challenges, taking the environment information (HD maps) into account could be beneficial to predetermine where targets are more or less likely to appear. Compared with semantic maps, HD maps formed by point clouds provide much richer information from surrounding static objects and scenes. In this work, we construct a GNN-based feature extraction of point cloud maps to increase the receptive fields of learning map features. Our work is based on PVRCNN, the state-of-the-art LiDAR object detection method. With point-wise and voxel-wise features obtained from PVRCNN, residual feature fusion is proposed to fuse the features from PVRCNN and the map features from GNN. Our approach is evaluated on NuScenes dataset. It achieves a 24.78% average precision improvement for long-range objects at 40-50 meters, the farthest areas with ground truth annotation. Our approach also has a 4.22% reduction of false positives in the entire sensing areas.
更多查看译文
关键词
LiDAR, Object Detection, Self-Driving Cars, Point Cloud Maps
AI 理解论文
溯源树
样例
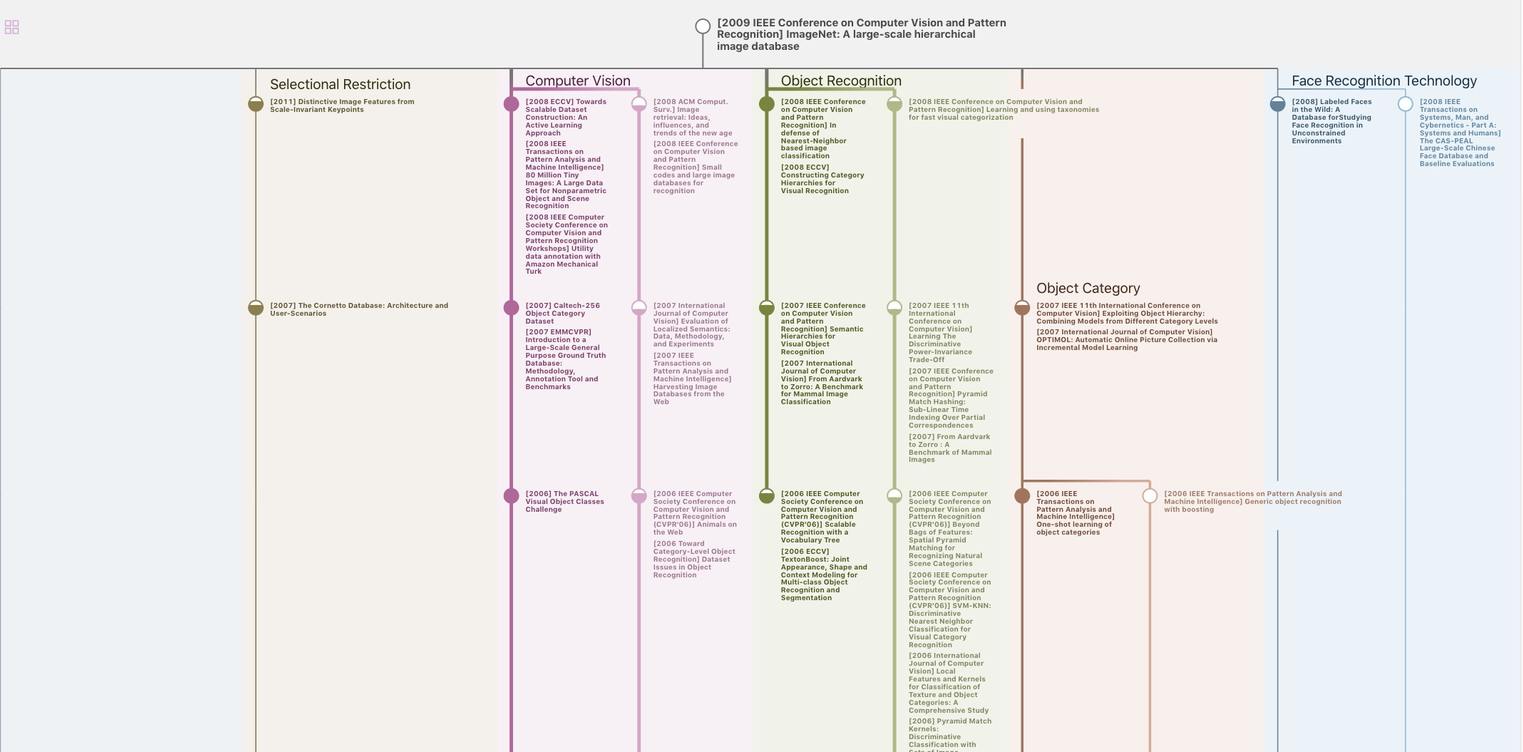
生成溯源树,研究论文发展脉络
Chat Paper
正在生成论文摘要