Denoise Enhanced Neural Network with Efficient Data Generation for Automatic Sleep Stage Classification of Class Imbalance.
IJCNN(2023)
Abstract
Signal-channel electroencephalogram (EEG) based automatic sleep stage classification with machine learning is widely used in the study of sleep quality and analysis of sleep disorders. While due to the inevitable class imbalance problem, relatively poor accuracy in the detection of the first stage of the Non-Rapid-Eye-Movement (N1) is found. In this work, we propose to use a generative adversarial network (GAN) based on transformer encoder to reduce the class imbalance problem. In order to ensure the quality of the synthetic signal, the percentage form of the Kullback-Leibler divergence (KLP) index is designed to measure the similarity of synthetic signals generated by GAN and real ones. Meanwhile, we design a Residual Shrinkage Sequence Network for Sleep Staging (RsSleepNet) as the baseline to compare other resolutions of class imbalance with ours. The performance of the new method with the combination of GAN and RsSleepNet is effectively verified on two public datasets from PhysioNet, in which the accuracy of the N1 stage can be improved by more than 10% as compared to the current state-of-the-art approaches, largely alleviating the class imbalance problem in automatic sleep stage classification.
MoreTranslated text
Key words
Sleep Staging,Class Imbalance,Data Generation
AI Read Science
Must-Reading Tree
Example
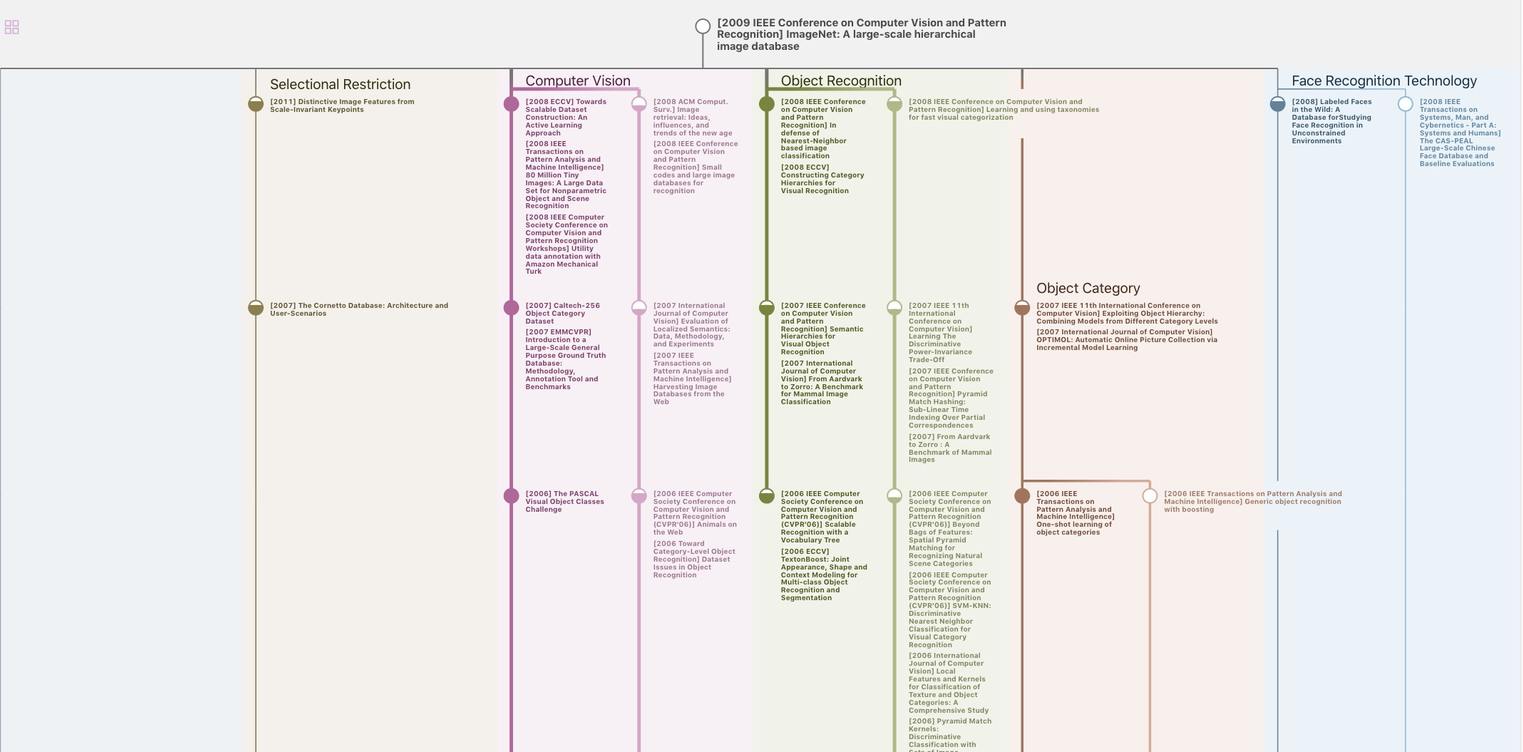
Generate MRT to find the research sequence of this paper
Chat Paper
Summary is being generated by the instructions you defined