MASZSL: A Multi-Block Attention-Based Description Generative Adversarial Network for Knowledge Graph Zero-Shot Relational Learning
IJCNN(2023)
摘要
In the real world, the Knowledge Graph(KG) is dynamic and new entities are added at any time. Therefore, open-world Knowledge Graph Completion(KGC) was proposed to approach new-added entities, but previous approaches often introduced too much noise when introducing external text resources for new entities. To alleviate this problem, knowledge graph zero-shot relational learning (KGZSL) has recently attracted more attention. Generative Adversarial Networks (GANs) are frequently used in KGZSL to connect existing relation descriptions to the domain of knowledge graphs. However, these methods ignore the impact of existing entities on embeddings for unidentified relational representations and in-stead concentrate on examining the connection between relational textual texts and knowledge network structures. In this work, we propose a multi-block attention framework using relation Description Generative Adversarial Networks (desGAN) jointing KG and text representation and address model collapse and training stability problems in previous studies. The core idea of our method is to obtain the background knowledge graph information and the relation representation through and multi-block attention layer and the desGAN, then a connection between the structured KG semantic space and the unstructured text semantic space of the new entity is established, forcing the entity pair to be closer to their real relation. Experimental results on the knowledge graph zeroshot relational learning dataset demonstrate that our MASZSL has a faster convergence speed and achieves state-of-the-art performance on this task.
更多查看译文
关键词
Knowledge Graph,Zero-shot Relational Learning,Generative Adversarial Networks
AI 理解论文
溯源树
样例
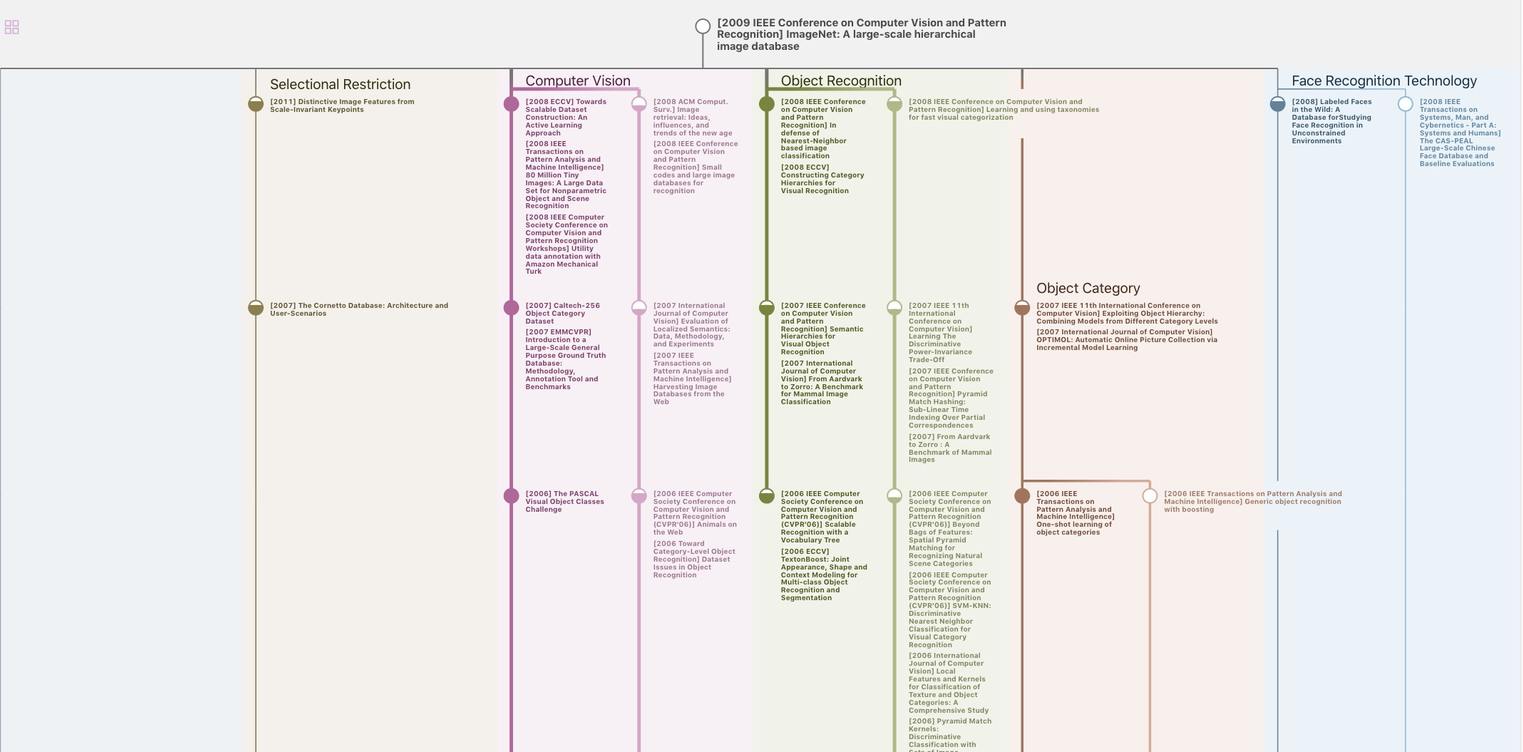
生成溯源树,研究论文发展脉络
Chat Paper
正在生成论文摘要