Homeostatic Reinforcement Learning through Soft Behavior Switching with Internal Body State.
IJCNN(2023)
摘要
The embodied autonomous agent, such as a household robot or a pet robot, is required to satisfy multiple requirements simultaneously. One possible approach would be to train the agent to simultaneously solve multiple tasks using a single physical entity (i.e., the body). However, integrating multiple tasks is not a trivial problem in the reward design paradigm of reinforcement learning (RL). Homeostatic RL treats the integration of multiple tasks as a control problem over the agent's internal bodily state. Still, learning in previous studies was extremely slow. In this study, we report novel behavior switching architectures: the Interoceptive Mixture of Experts (IMoE) and the Interoceptive Behavior Switching (IBS) for homeostatic RL agents with continuous motor control. In these architectures, the agent switches between multiple policies using internal body states (interoception). We tested IMoE and IBS in four homeostatic RL environments. We also compared IMoE and IBS with a fully connected model and a query key value switching model with full observation policies. The results indicate that the proposed architectures provide better or competitive results in all four benchmark environments.
更多查看译文
关键词
Homeostatic Reinforcement Learning, Homeostasis, Deep Reinforcement Learning, Neural Architecture
AI 理解论文
溯源树
样例
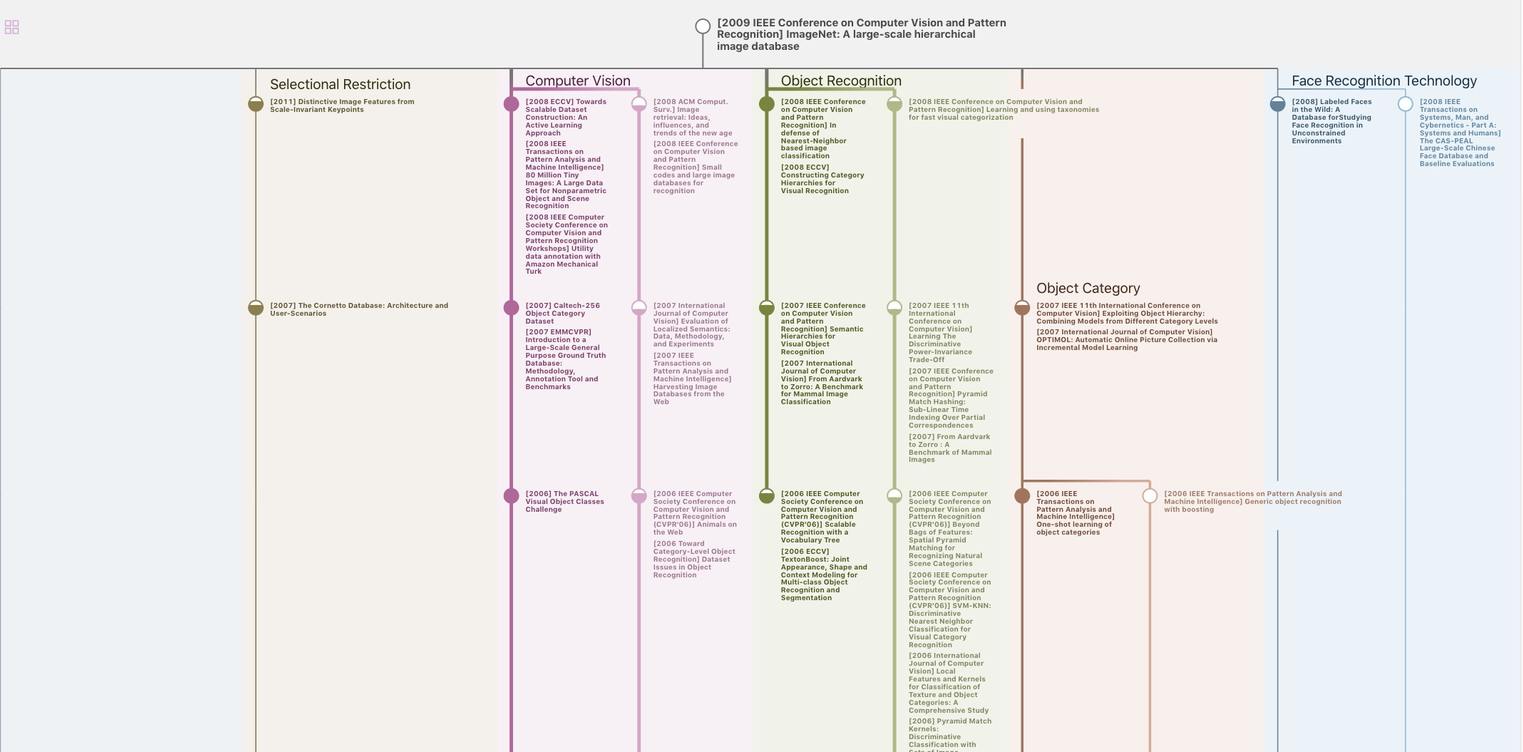
生成溯源树,研究论文发展脉络
Chat Paper
正在生成论文摘要