On Hyperdimensional Computing-based Federated Learning: A Case Study.
IJCNN(2023)
摘要
Federated learning is a decentralized machine learning strategy that trains the model by using data stored across multiple decentralized edge devices or servers. Studies on federated learning currently focus primarily on neural network-based learning methods, which usually require powerful hardware and are relatively not energy-efficient. Recently, hyperdimensional computing (HDC) emerges as a potential alternative solution to neural networks, particularly on resource-constrained platforms such as edge intelligence systems. HDC mimics the "human brain" at the functionality level that learns with the attributes of brain circuits, including high-dimensionality and fully distributed holographic representation. Although there are existing works related to HDC-based federated learning, a comprehensive study on how HDC-based federated learning performs in different settings is still absent. To bridge this gap, we present a comprehensive case study on federated learning using HDC under two model aggregation strategies: hypervector aggregation and associative memory aggregation. We also perform extensive experiments with various settings, including data distribution, number of clients, and local training epochs. We also analyze their communication costs under these settings. Our results show that using the strategy of associative memory aggregation can achieve up to 95% communication cost reduction compared to hypervector aggregation. In addition, HDC-based federated learning system shows high robustness in training with Non-IID data. This study aims to shed light and provide guidance in opening up new directions and challenges for future HDC-based federated learning system design and optimization.
更多查看译文
关键词
associative memory aggregation,decentralized machine learning strategy,HDC-based federated learning,hyperdimensional computing-based federated learning,hypervector aggregation,multiple decentralized edge devices,neural network-based learning methods,nonIID data
AI 理解论文
溯源树
样例
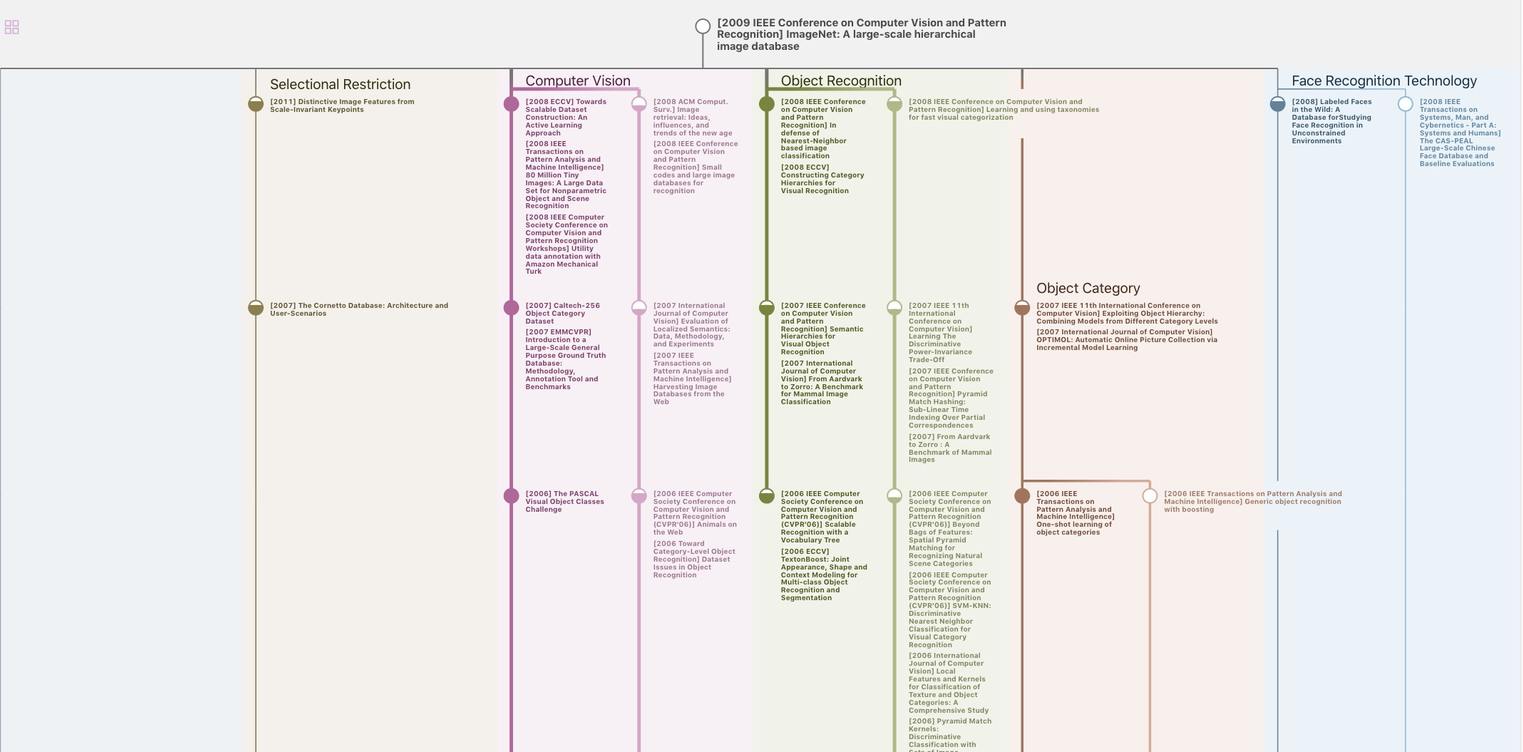
生成溯源树,研究论文发展脉络
Chat Paper
正在生成论文摘要