Explainable Sequential Anomaly Detection via Prototypes.
IJCNN(2023)
摘要
Sequential anomaly detection has received more and more attention because of its wide applications in various domains, such as debugging system failures via logs. Researchers have recently proposed many deep learning-based approaches for sequential anomaly detection. However, these approaches work as black-boxed models, not providing explanations for detected anomalies. On the other hand, explainability is a critical requirement to build trustworthiness in detection results. Moreover, domain experts would like to learn why a sequence is labeled as an anomaly. To overcome this challenge, in this paper, we propose a framework for Explainable Sequential Anomaly Detection (ESAD) in a semi-supervised setting. As there are various normal and abnormal behaviors in sequential data, ESAD derives multiple prototypes to describe diverse normal and abnormal sequences. Each prototype can encode one type of normal or abnormal behavior. Given a new sequence, if the sequence is similar to an abnormal prototype, the sequence will be detected as abnormal. After decoding the abnormal prototype as a prototypical sequence, domain experts can further understand the newly detected abnormal sequence by examining the prototypical sequence. We conduct experiments on one log dataset and two text datasets. Experimental results including quantitative and qualitative analysis on three datasets show the effectiveness of our model.
更多查看译文
关键词
sequential data,anomaly detection,explanations
AI 理解论文
溯源树
样例
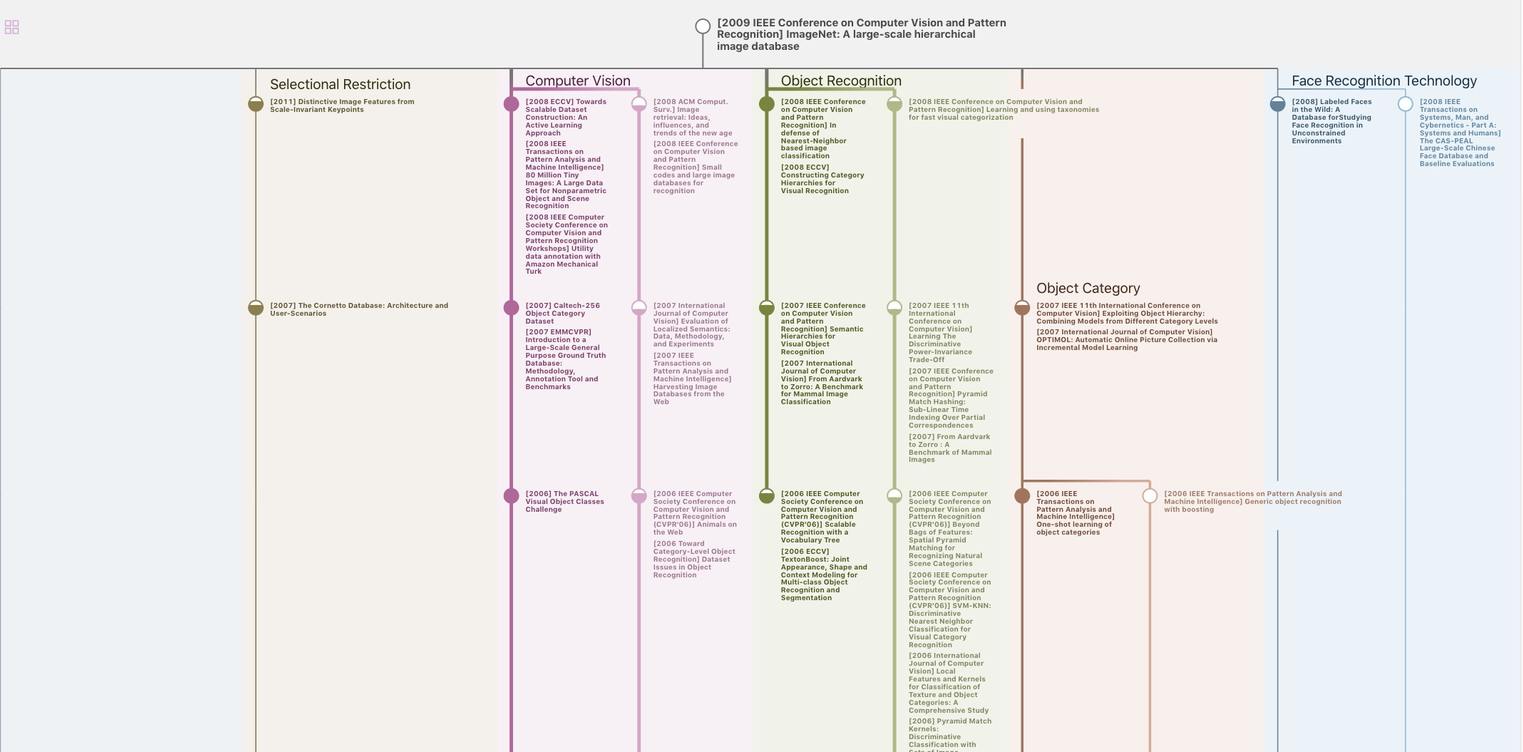
生成溯源树,研究论文发展脉络
Chat Paper
正在生成论文摘要