Schema Item Matters in Knowledge Base Question Answering.
IJCNN(2023)
摘要
Knowledge base question answering is a challenging task that aims to answer questions by querying knowledge bases. Recently, state-of-the-art methods tend to include an enumerator module and a ranker module. They first enumerate by searching the knowledge base and then rank the candidates to select the target logical form. However, these methods sometimes fail to cover the candidates which involve more complex combinations. A recent solution to this issue is to add a generator module after the ranker to generate the uncovered target logical form. However, the enumerator and ranker always discard partial ground truth schema items. Consequently, the lack of them in the generator input results in the failure to generate the target logical form. To address this problem, we present a novel framework, SIMQA, to reuse the neglected schema items, i.e., classes and relations. Specifically, we adopt a matcher module to select the most related schema items for the given question, and feed them to the generator. On this basis, we propose a novel generation model based on contrastive learning to force the model to focus on the supplemental schema items. Experiment results on GRAILQA and WEBQSP datasets demonstrate the highly competitive performance of the proposed method, and verify that schema item matters in KBQA.
更多查看译文
关键词
enumerate,enumerator module,generation model,generator input results,generator module,knowledge base question answering,matcher module,neglected schema items,partial ground truth schema items,ranker module,related schema items,schema item matters,supplemental schema items,uncovered target logical form
AI 理解论文
溯源树
样例
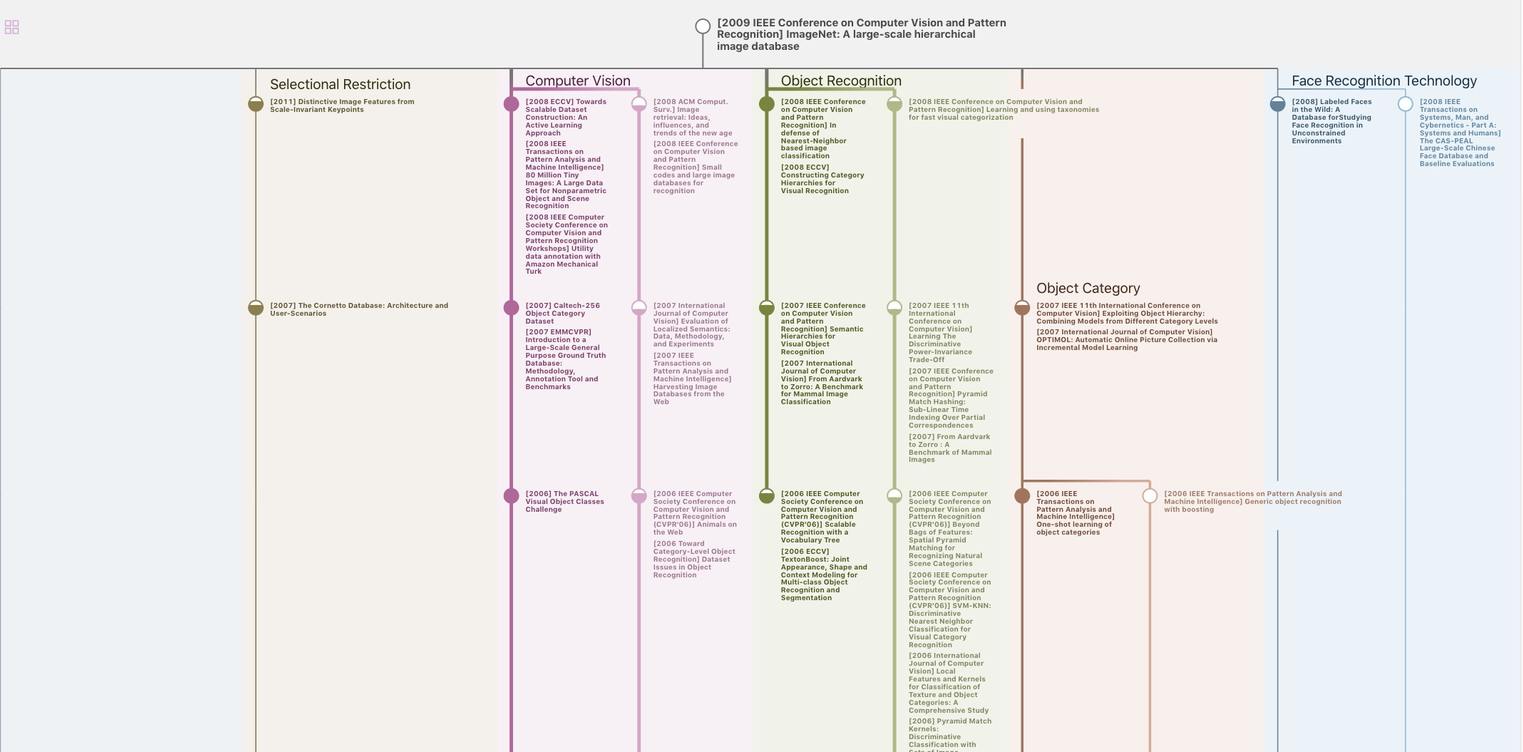
生成溯源树,研究论文发展脉络
Chat Paper
正在生成论文摘要