Measurement and Identification of Informative Reviews for Automated Summarization
2023 IEEE International Conference On Artificial Intelligence Testing (AITest)(2023)
摘要
This research investigates the impact of data quality to the quality of text summarization using the software review summarization as a case study. It answers three research questions: 1. What is the most important quality dimension for measuring the quality of software reviews for fitting the review summarization purpose? Our answer is the informativeness of reviews. We propose a metric to measure informativeness and use it to identify highly informative reviews for training review summarization models. 2. How does the review quality affect the quality of review summarization? We conducted the review summarization experiments with a group of datasets that have different quality settings to answer the question. Based on the experiment results, we propose a sampling method for identifying high quality reviews, and the experiment results indicate that the method can significantly improve quality of review summarization on two large review datasets. Furthermore, the results show that the models trained on the selected dataset maintain a balance of bias and variance. 3. Do all text summarization models perform equally well on the datasets? We conduct a comparative study of review summarization on two state-of-the-art deep learning models BART and T5 to answer the question. The research results showing that identifying highly informative reviews is a new direction for improving quality of review summarization.
更多查看译文
关键词
Text summarization, abstractive summarization, information quality, software review, informativeness, generative language model
AI 理解论文
溯源树
样例
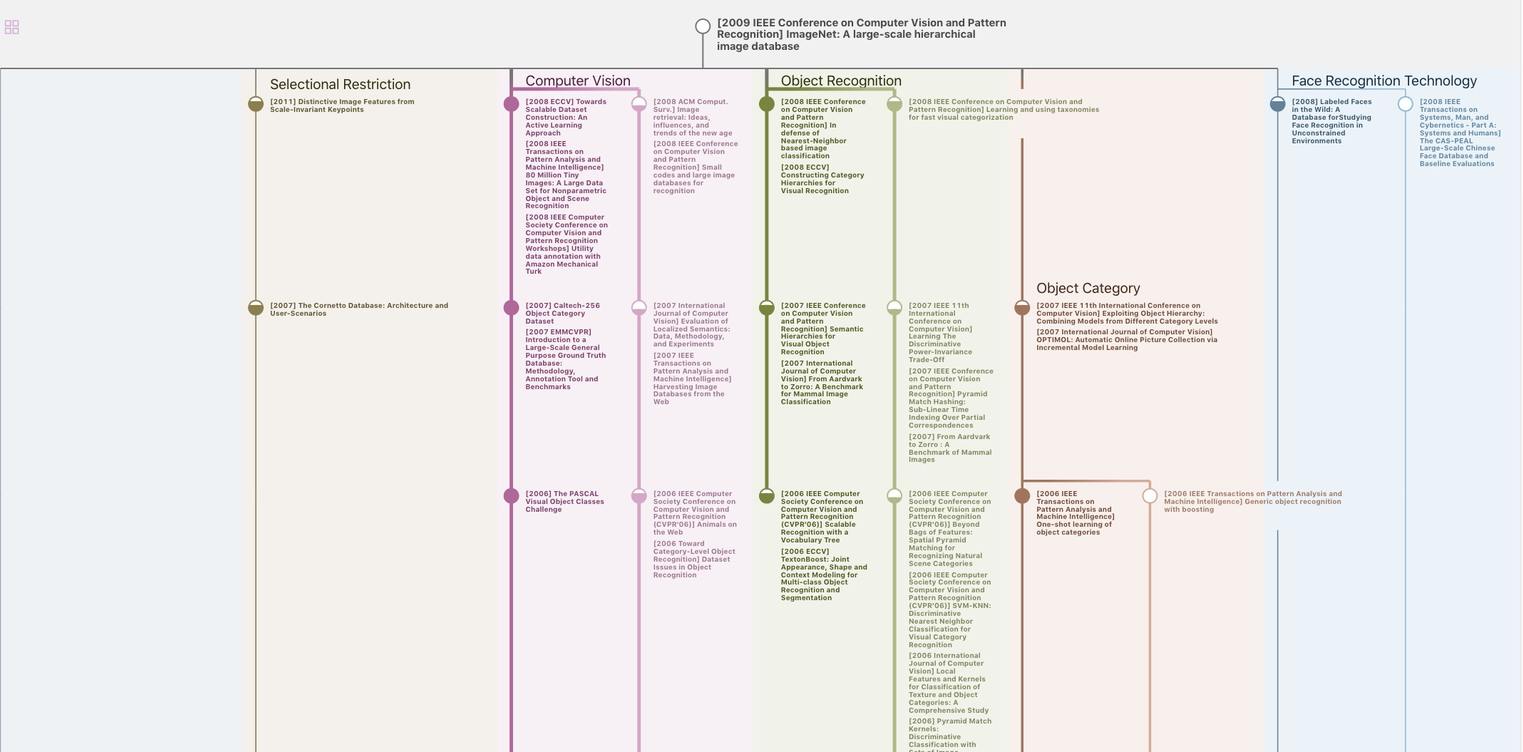
生成溯源树,研究论文发展脉络
Chat Paper
正在生成论文摘要