A Novel Dual-Center-Based Intuitionistic Fuzzy Twin Bounded Large Margin Distribution Machines
IEEE Transactions on Fuzzy Systems(2023)
摘要
Intuitionistic fuzzy (IF) set theory combined with twin support vector machines (TSVM) has shown highly advantageous performance in robust and fast classification. However, the existing methods have some inherent flaws: 1) The inaccurate confidence caused by the single-center-based IF strategy degrades the classification performance of the model. 2) The nonmembership degree is time-consuming and the threshold is difficult to determine, as it depends on sample's local neighborhood set. 3) Recent theoretical studies have demonstrated that optimizing the margin distribution achieve better generalization performance than maximizing the minimum margin in SVM-based models (SVMs). In view of the aforementioned shortcomings, we propose a novel dual-center based IF (DC-IF) function, and introduce the margin distribution into IFTSVM, forming a DC-IF twin bounded large margin distribution machines (DC-IFTBLDM). Initially, the DC-IF strategy references the contribution of one sample to both categories, which is time efficient and more accurately reflects the credibility of the samples. Moreover, the margin mean and variance are used to optimize the margin distribution, and a regularization term is utilized to minimize structural risk. Therefore, DC-IFTBLDM has a good performance in noise immunity and classification capability. Comparative experiments based on synthetic datasets, UCI benchmark datasets, and datasets with different noise ratios verify the effectiveness of the proposed model. The parameter sensitivity analysis further proves the high accuracy and stability of DC-IFTBLDM.
更多查看译文
关键词
Antinoise performance,intuitionistic fuzzy,margin distribution,twin support vector machines
AI 理解论文
溯源树
样例
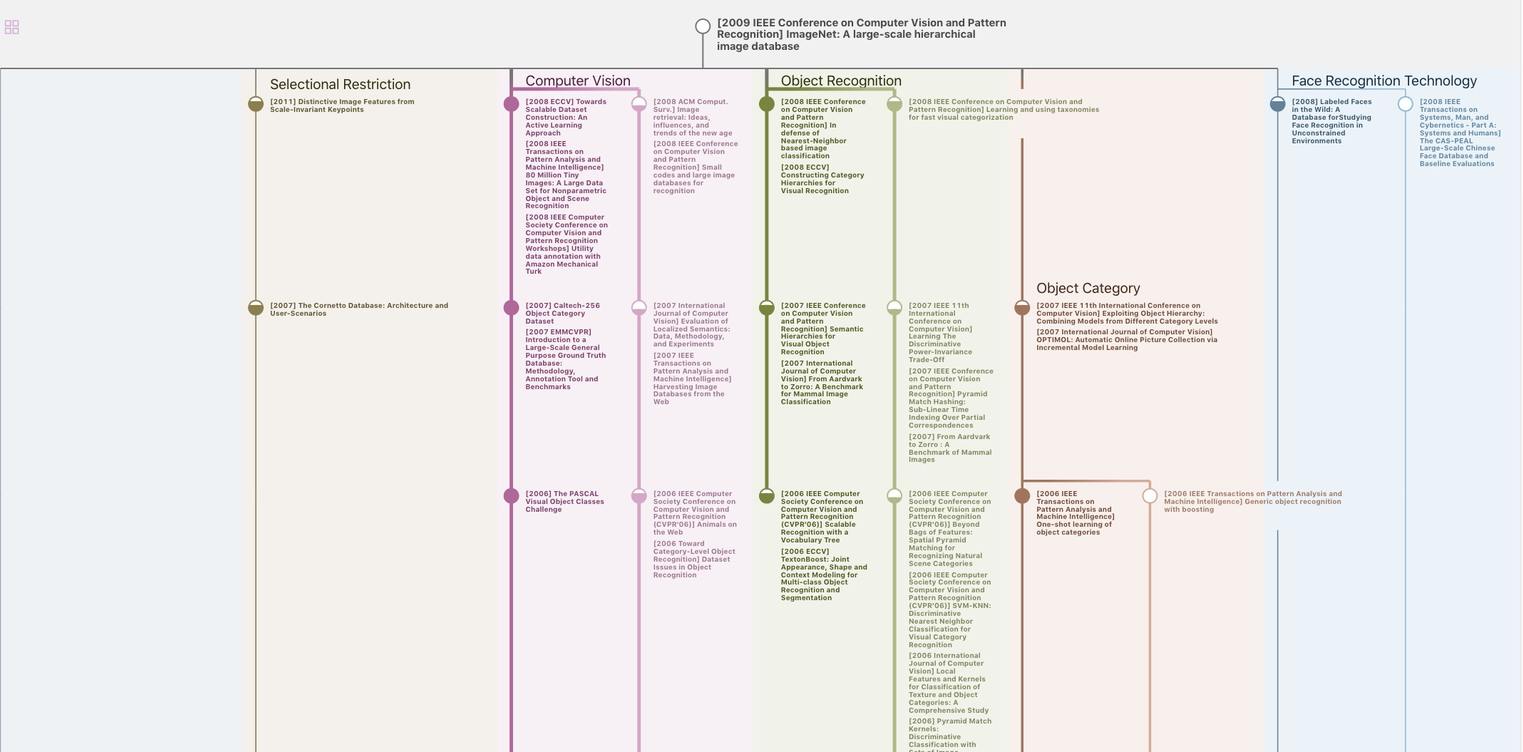
生成溯源树,研究论文发展脉络
Chat Paper
正在生成论文摘要