Determining prescriptions in electronic healthcare record data: methods for development of standardized, reproducible drug codelists
JAMIA OPEN(2023)
摘要
Objective: To develop a standardizable, reproducible method for creating drug codelists that incorporates clinical expertise and is adaptable to other studies and databases. Materials and Methods: We developed methods to generate drug codelists and tested this using the Clinical Practice Research Datalink (CPRD) Aurum database, accounting for missing data in the database. We generated codelists for: (1) cardiovascular disease and (2) inhaled Chronic Obstructive Pulmonary Disease (COPD) therapies, applying them to a sample cohort of 335 931 COPD patients. We compared searching all drug dictionary variables (A) against searching only (B) chemical or (C) ontological variables. Results: In Search A, we identified 165 150 patients prescribed cardiovascular drugs (49.2% of cohort), and 317 963 prescribed COPD inhalers (94.7% of cohort). Evaluating output per search strategy, Search C missed numerous prescriptions, including vasodilator anti-hypertensives (A and B:19 696 prescriptions; C:1145) and SAMA inhalers (A and B:35 310; C:564). Discussion: We recommend the full search (A) for comprehensiveness. There are special considerations when generating adaptable and generalizable drug codelists, including fluctuating status, cohort-specific drug indications, underlying hierarchical ontology, and statistical analyses. Conclusions: Methods must have end-to-end clinical input, and be standardizable, reproducible, and understandable to all researchers across data contexts.
更多查看译文
关键词
code sets,value sets,electronic medical records,epidemiology,health data science,misclassification bias
AI 理解论文
溯源树
样例
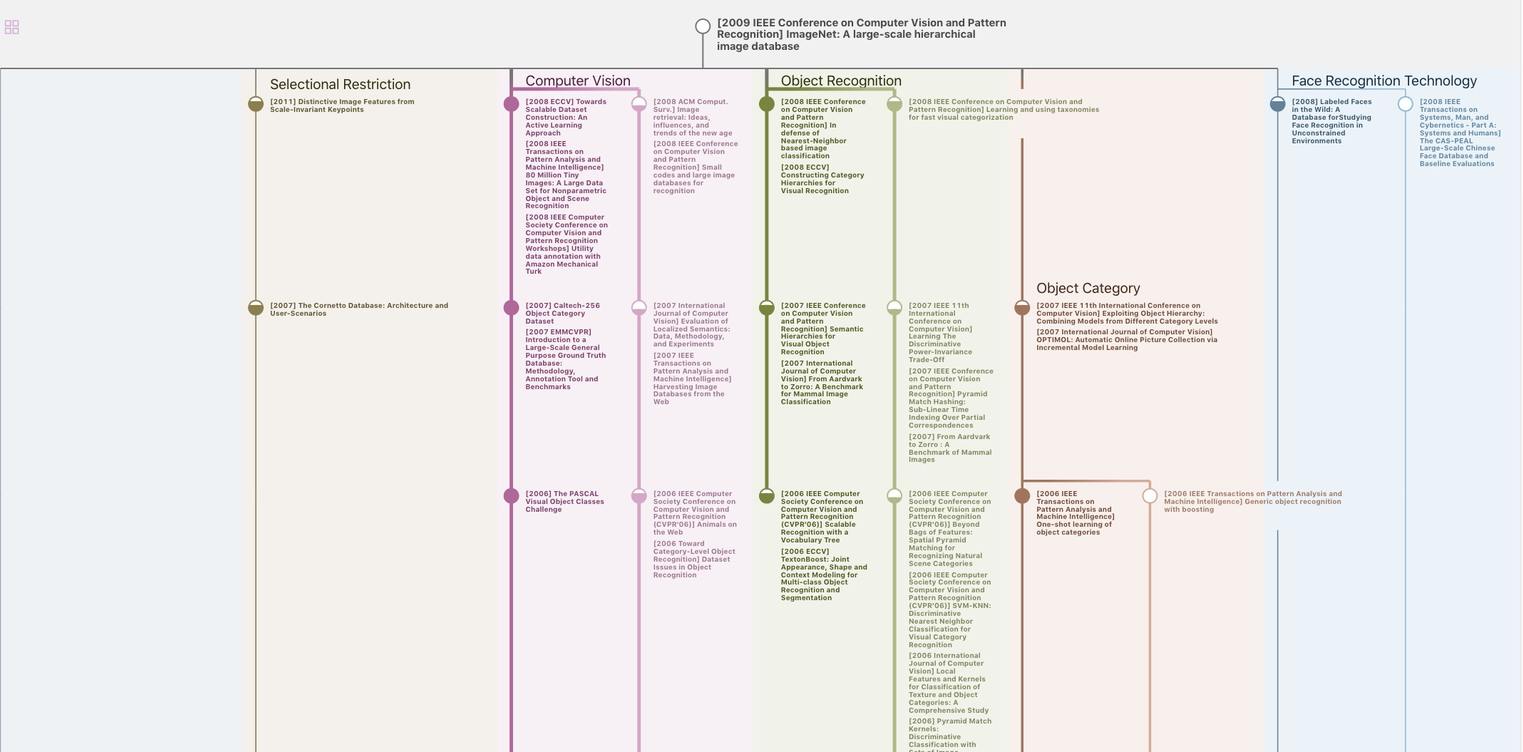
生成溯源树,研究论文发展脉络
Chat Paper
正在生成论文摘要