Edge-Coordinated On-Road Perception for Connected Autonomous Vehicles Using Point Cloud
2023 Biennial Symposium on Communications (BSC)(2023)
摘要
In this paper, we tackle the problem of selecting connected autonomous vehicles (CAVs) with the most valuable point cloud data for edge-coordinated on-road perception. Through extensive experiments, we find that adding a CAV for collaborative perception yields diminishing gain in understanding the on-road environments, while the generated point cloud data size grows linearly with the number of employed CAVs. Meanwhile, both vehicular mobility and diversified road topology lead to the dynamics of data size of the captured point clouds. Based on those findings, we then formulate an optimization problem that maximizes the utility of collaborative perception at edge nodes. Considering the submodularity of collaborative perception utility and heterogeneity of point cloud data size from individual vehicles, a CAV candidate selection algorithm is proposed. The marginal gain of processing the point cloud data of each candidate is firstly evaluated and ranked, based on which a subset of CAVs are selected subject to bandwidth capacity. Finally, experimental results on an open dataset are presented to demonstrate the superiority of the proposed algorithm under dynamic traffic conditions and bandwidth fluctuations.
更多查看译文
关键词
captured point clouds,CAV candidate selection algorithm,collaborative perception utility,collaborative perception yields,diversified road topology lead,edge nodes,edge-coordinated on-road perception,employed CAVs,generated point cloud data size,on-road environments,selecting connected autonomous vehicles,valuable point cloud data
AI 理解论文
溯源树
样例
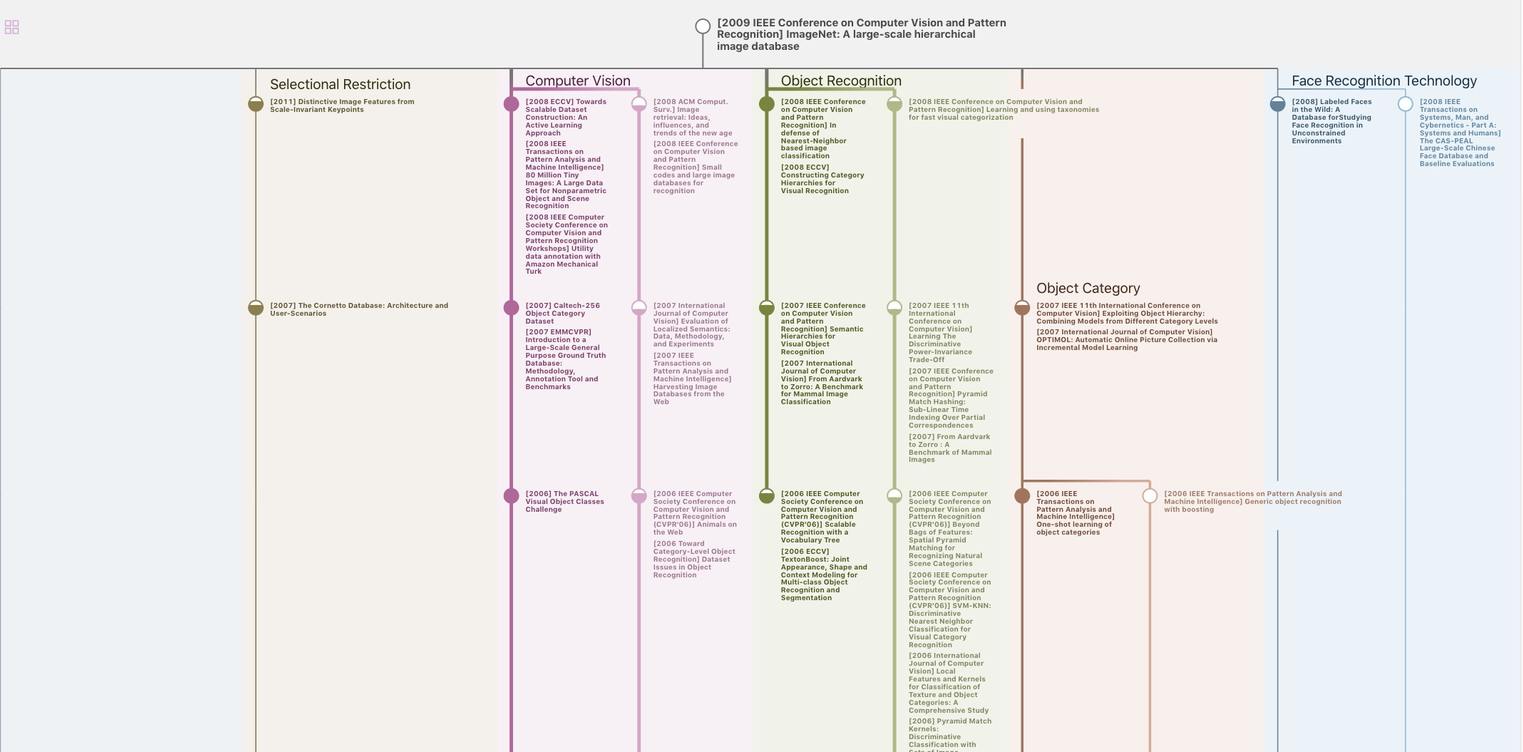
生成溯源树,研究论文发展脉络
Chat Paper
正在生成论文摘要