Estimating the instantaneous reproduction number (Rt) by using particle filter
Infectious Disease Modelling(2023)
摘要
Background Monitoring the transmission of coronavirus disease 2019 (COVID-19) requires accurate estimation of the effective reproduction number ( R t ). However, existing methods for calculating R t may yield biased estimates if important real-world factors, such as delays in confirmation, pre-symptomatic transmissions, or imperfect data observation, are not considered.
Method To include real-world factors, we expanded the susceptible-exposed-infectious-recovered (SEIR) model by incorporating pre-symptomatic (P) and asymptomatic (A) states, creating the SEPIAR model. By utilizing both stochastic and deterministic versions of the model, and incorporating predetermined time series of R t , we generated simulated datasets that simulate real-world challenges in estimating R t . We then compared the performance of our proposed particle filtering method for estimating R t with the existing EpiEstim approach based on renewal equations.
Results The particle filtering method accurately estimated R t even in the presence of data with delays, pre-symptomatic transmission, and imperfect observation. When evaluating via the root mean square error (RMSE) metric, the performance of the particle filtering method was better in general and was comparable to the EpiEstim approach if perfectly deconvolved infection time series were provided, and substantially better when R t exhibited short-term fluctuations and the data was right truncated.
Conclusions The SEPIAR model, in conjunction with the particle filtering method, offers a reliable tool for predicting the transmission trend of COVID-19 and assessing the impact of intervention strategies. This approach enables enhanced monitoring of COVID-19 transmission and can inform public health policies aimed at controlling the spread of the disease.
### Competing Interest Statement
The authors have declared no competing interest.
### Funding Statement
This research was partly supported by Government-wide R&D Fund project for infectious di disease research (GFID), Republic of Korea (grant number: HG18C0088) and National Institute for Mathematical Sciences (NIMS) grant funded by the Korean Government (NIMS-B21910000)
### Author Declarations
I confirm all relevant ethical guidelines have been followed, and any necessary IRB and/or ethics committee approvals have been obtained.
Yes
The details of the IRB/oversight body that provided approval or exemption for the research described are given below:
Source data were publicly available at Korea Disease Control Agency.
I confirm that all necessary patient/participant consent has been obtained and the appropriate institutional forms have been archived, and that any patient/participant/sample identifiers included were not known to anyone (e.g., hospital staff, patients or participants themselves) outside the research group so cannot be used to identify individuals.
Yes
I understand that all clinical trials and any other prospective interventional studies must be registered with an ICMJE-approved registry, such as ClinicalTrials.gov. I confirm that any such study reported in the manuscript has been registered and the trial registration ID is provided (note: if posting a prospective study registered retrospectively, please provide a statement in the trial ID field explaining why the study was not registered in advance).
Yes
I have followed all appropriate research reporting guidelines, such as any relevant EQUATOR Network research reporting checklist(s) and other pertinent material, if applicable.
Yes
All data produced are available online at
更多查看译文
关键词
Particle filter, Sequential Monte Carlo, Effective reproduction number, COVID-19, Transmission model, Compartment model
AI 理解论文
溯源树
样例
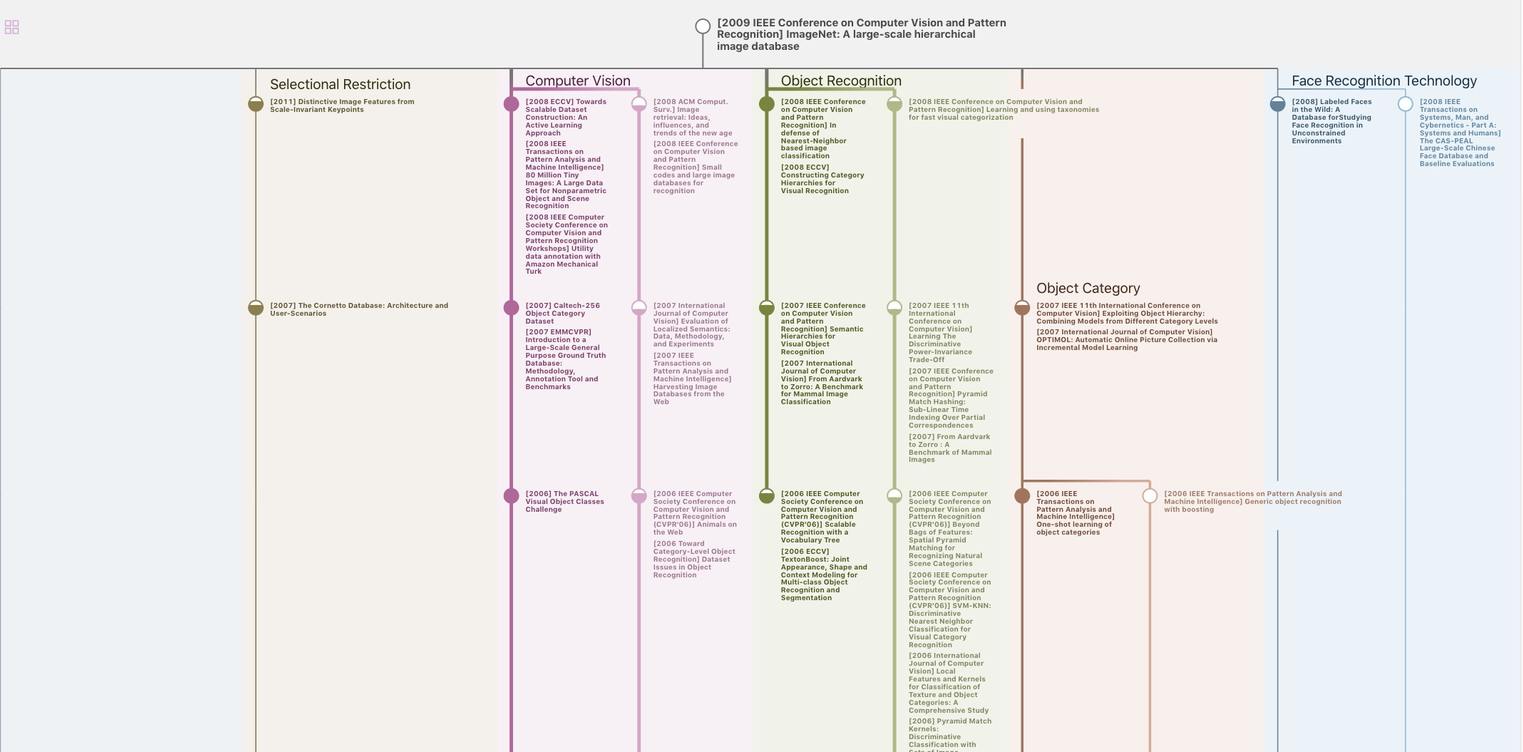
生成溯源树,研究论文发展脉络
Chat Paper
正在生成论文摘要