Augmenting Radar Data via Sampling from Learned Latent Space
2023 IEEE 3rd International Conference on Computer Communication and Artificial Intelligence (CCAI)(2023)
摘要
Data augmentation is a widely used technique to regularize deep learning models. It is especially famous in computer vision due to its simplicity to apply. Literature suggests numerous ways of transforming images without changing the characteristic semantics. However, for data coming from sensors such as radar these approaches are not applicable leading to data augmentation being not commonly performed. To solve this problem and close the gap we investigate how a Variational Autoencoder (VAE) can be trained on radar data to sample from the learned latent space and use the resulting data to regularize the training of a classifier. We run our experiments on two radar gesture datasets and show that the introduction of generated data can increase generalization. We investigate whether the learned embedded space is sufficient and propose how to sample from the latent space while preserving labels for successful supervised training.
更多查看译文
关键词
radar,artificial intelligence,variational autoencoder,data augmentation,regularization
AI 理解论文
溯源树
样例
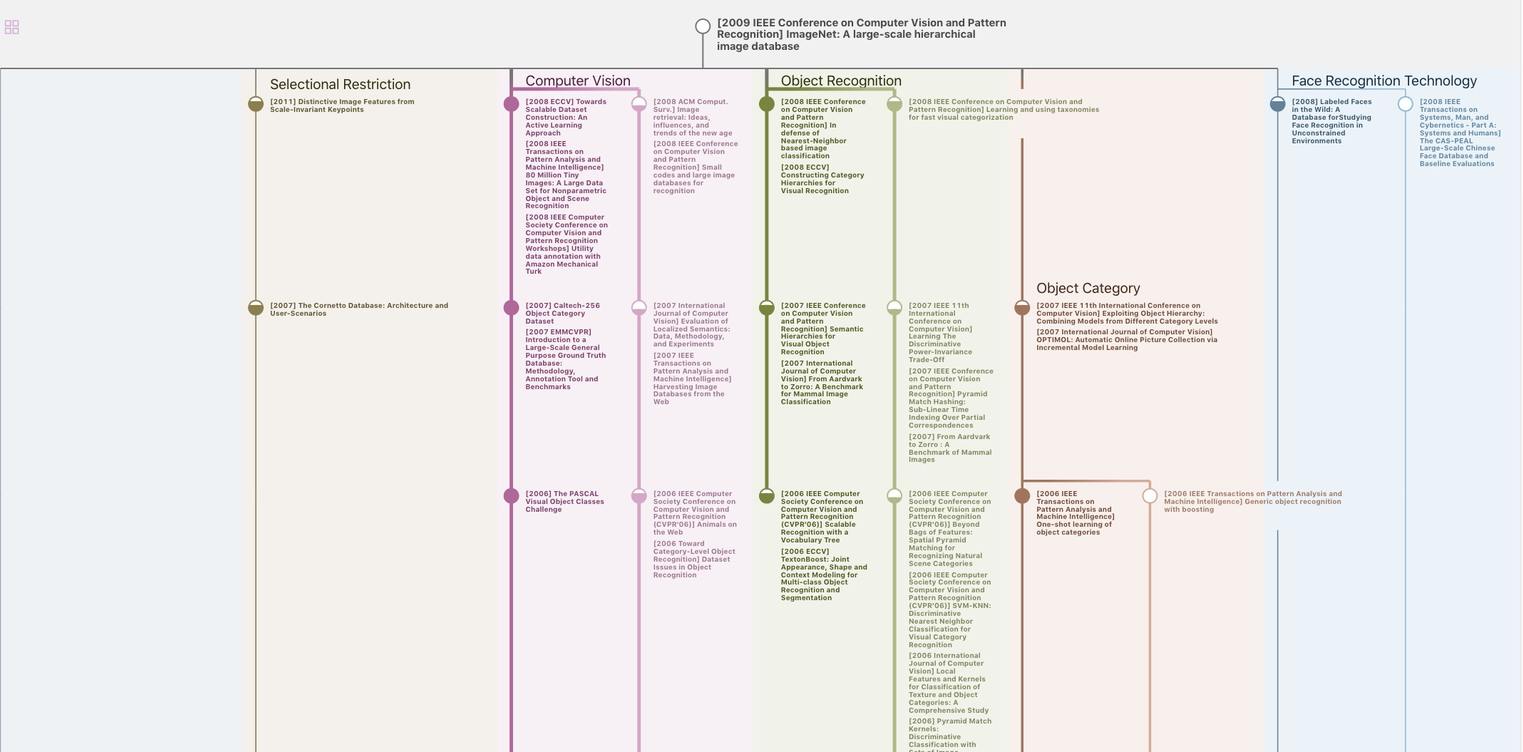
生成溯源树,研究论文发展脉络
Chat Paper
正在生成论文摘要