Q-Drug: a Framework to bring Drug Design into Quantum Space using Deep Learning
arXiv (Cornell University)(2023)
摘要
Optimizing the properties of molecules (materials or drugs) for stronger toughness, lower toxicity, or better bioavailability has been a long-standing challenge. In this context, we propose a molecular optimization framework called Q-Drug (Quantum-inspired optimization algorithm for Drugs) that leverages quantum-inspired algorithms to optimize molecules on discrete binary domain variables. The framework begins by encoding the molecules into binary embeddings using a discrete VAE. The binary embeddings are then used to construct an Ising energy-like objective function, over which the state-of-the-art quantum-inspired optimization algorithm is adopted to find the optima. The binary embeddings corresponding to the optima are decoded to obtain the optimized molecules. We have tested the framework for optimizing drug molecule properties and have found that it outperforms other molecular optimization methods, finding molecules with better properties in 1/20th to 1/10th of the time previously required. The framework can also be deployed directly on various quantum computing equipment, such as laser pulses CIMs, FPGA Ising Machines, and quantum computers based on quantum annealing, among others. Our work demonstrates a new paradigm that leverages the advantages of quantum computing and AI to solve practically useful problems.
更多查看译文
关键词
q-drug design,quantum space,deep
AI 理解论文
溯源树
样例
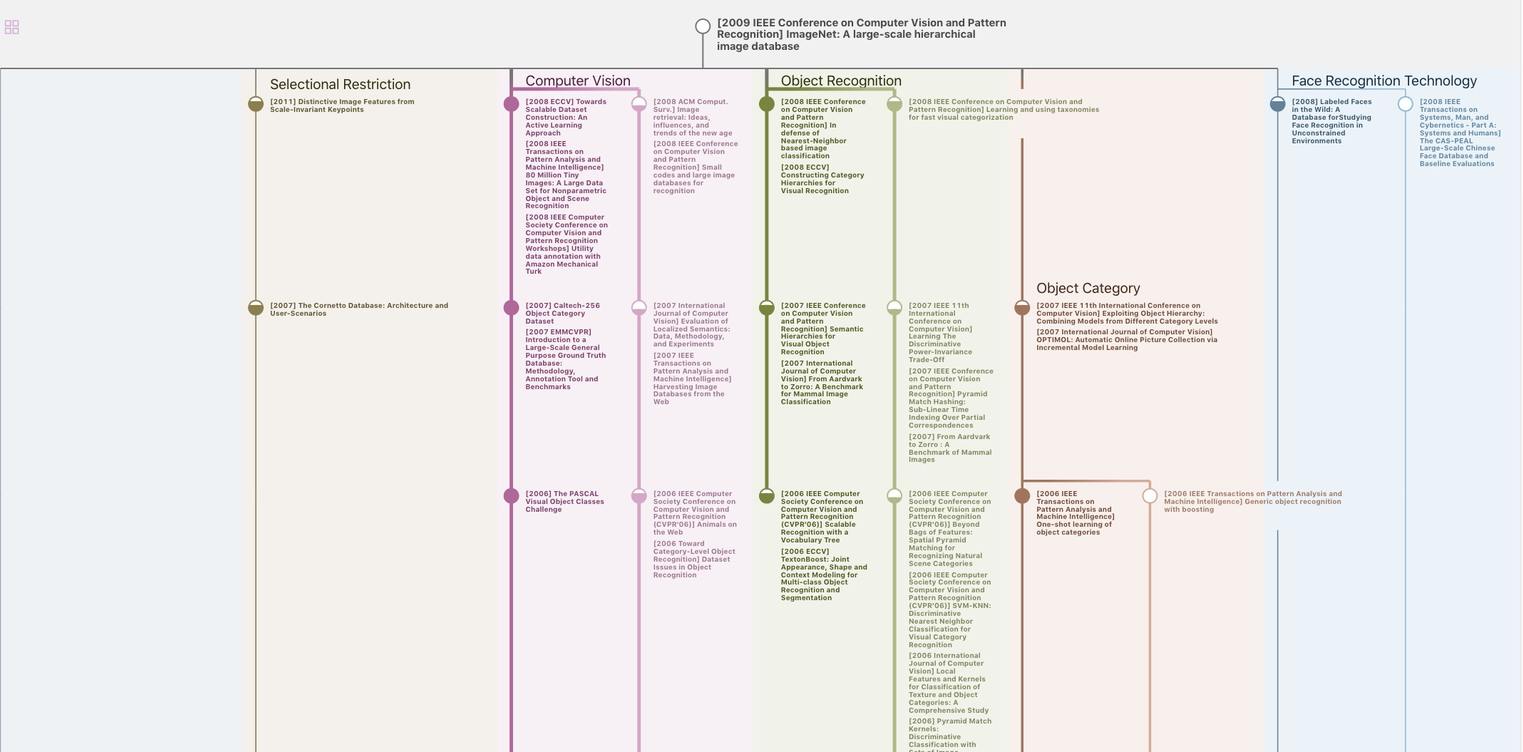
生成溯源树,研究论文发展脉络
Chat Paper
正在生成论文摘要