Conditional Molecular Generation Net Enables Automated Structure Elucidation Based on 13C NMR Spectra and Prior Knowledge
Analytical Chemistry(2023)
摘要
Structure elucidation of unknown compounds based on nuclear magnetic resonance (NMR) remains a challenging problem in both synthetic organic and natural product chemistry. Library matching has been an efficient method to assist structure elucidation. However, it is limited by the coverage of libraries. In addition, prior knowledge such as molecular fragments is neglected. To solve the problem, we propose a conditional molecular generation net (CMGNet) to allow input of multiple sources of information. CMGNet not only uses 13C NMR spectrum data as input but molecular formulas and fragments of molecules are also employed as input conditions. Our model applies large-scale pretraining for molecular understanding and fine-tuning on two NMR spectral data sets of different granularity levels to accommodate structure elucidation tasks. CMGNet generates structures based on 13C NMR data, molecular formula, and fragment information, with a recovery rate of 94.17% in the top 10 recommendations. In addition, the generative model performed well in the generation of various classes of compounds and in the structural revision task. CMGNet has a deep understanding of molecular connectivities from 13C NMR, molecular formula, and fragments, paving the way for a new paradigm of deep learning-assisted inverse problem-solving.
更多查看译文
关键词
automated structure elucidation,molecular
AI 理解论文
溯源树
样例
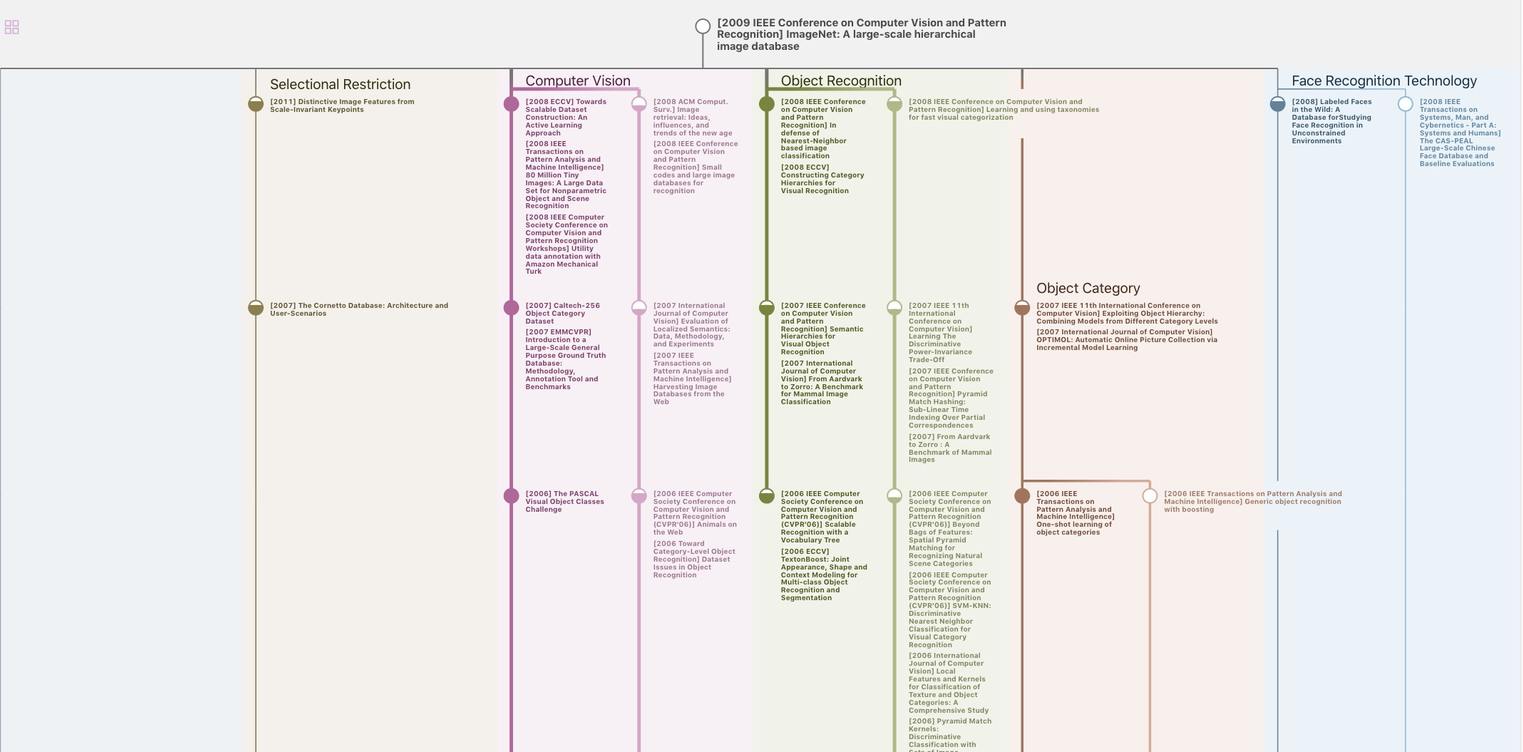
生成溯源树,研究论文发展脉络
Chat Paper
正在生成论文摘要