Optimization of Process Families for Deployment of Carbon Capture Processes using Machine Learning Surrogates
Computer-aided chemical engineering(2023)
摘要
Traditional process design approaches focus on exploiting economies of scale but are inefficient when designing a large number of similar processes for decentralized applications. By optimizing each process individually, these approaches do not allow for manufacturing standardization or make use of economies of numbers. In this work, we design a family of processes (i.e., multiple processes) of a carbon capture facility with various performance requirements. We identify sub-components within the process and create a small set of sub-component designs that can be shared across all the processes in the process family. We formulate this optimization problem as a nonlinear generalized disjunctive program (GDP) and, in previous work, developed two approaches for reformulating and solving this problem: one based on full-discretization of the design space (Zhang et al., 2022) and one that used Machine Learning (ML) surrogates to replace the nonlinear process models (Stinchfield et al., 2022). Using ML surrogates to predict required system costs and performance allows us to reformulate the nonlinearities in the GDP to generate an efficient MILP formulation. In this work, we apply the ML surrogate approach to design a family of carbon capture systems to cover a set of different flue gas flow rates and inlet CO2 concentrations, where we consider the absorber and stripper as sub-component types.
更多查看译文
关键词
carbon capture processes,carbon capture,machine learning,optimization
AI 理解论文
溯源树
样例
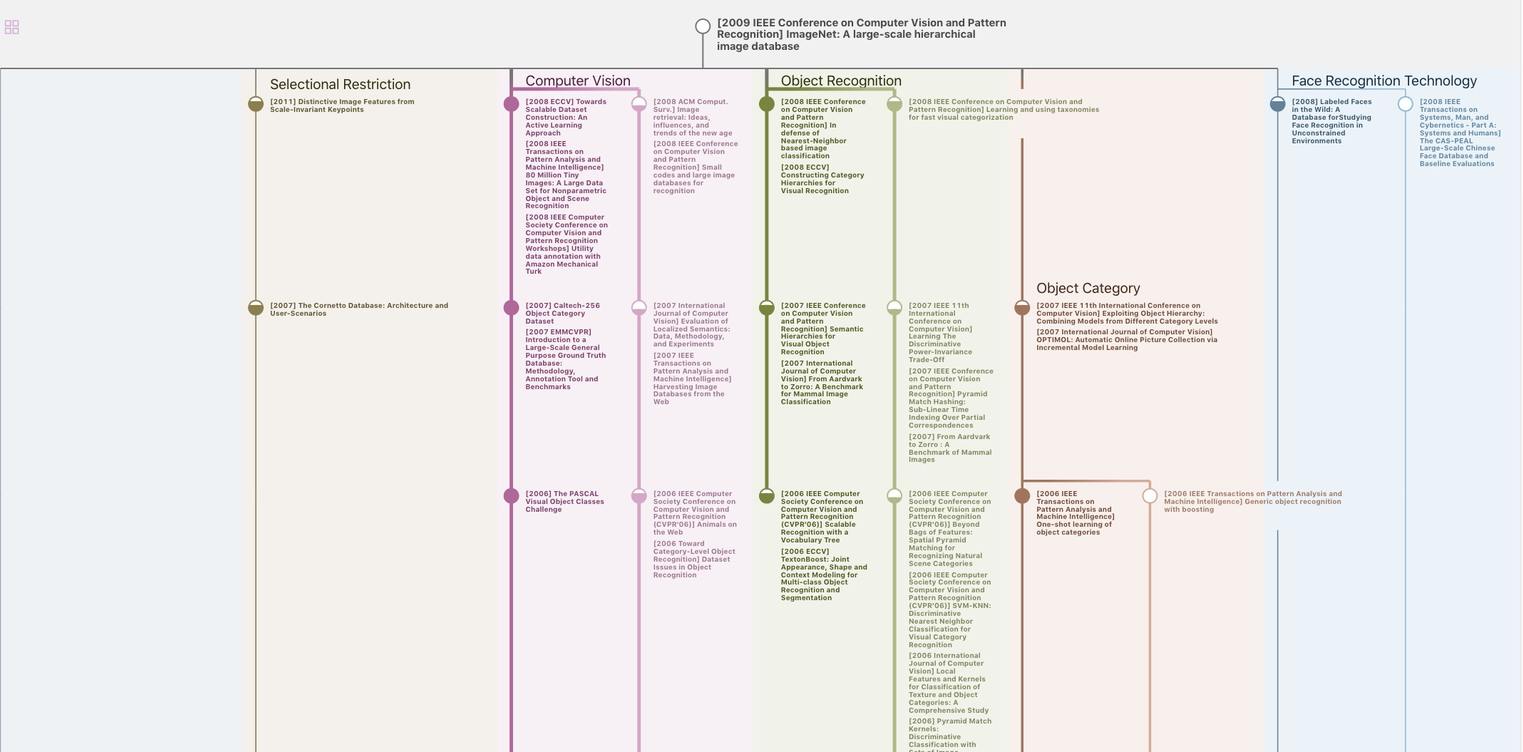
生成溯源树,研究论文发展脉络
Chat Paper
正在生成论文摘要