An Optimized Machine Learning Model for the Early Prognosis of Chronic Respiratory Diseases with specific focus On Asthma
Research Square (Research Square)(2023)
摘要
Abstract Clinicians and those into the field of clinical research often evaluate the benefits against costs and inconvenience of preventive interventions for asthma by performing risk identification and assessment through the application of Artificial Intelligence (AI) based predictive models. As such, a Weighted Feature Averaging Technique (WFAT), a novel feature extraction strategy that uniquely identifies the risk factors contributing to asthma, by taking into consideration the various aspects of weighing the importance of features with respect to their predictive capabilities. We introduce a weighted feature averaging technique to extract the most relevant and predominant features that score well on their relevance with respect to asthma outcome, the target for the problem under study. To strengthen the classification performance, ensemble machine learning techniques centric to AI techniques that are widely known to do so are explored and a new ensemble machine learning model, which is seen to outperform the weak classifiers by deploying optimization techniques that yield comparatively better results has been proposed. The ensemble model proposed yielded a classification accuracy as high as 85% and 91% with two diverse datasets chosen for modeling early prognosis of asthma disease by deploying only 25% of the features in the reduced feature set.
更多查看译文
关键词
optimized machine learning model,asthma,chronic respiratory diseases,machine learning
AI 理解论文
溯源树
样例
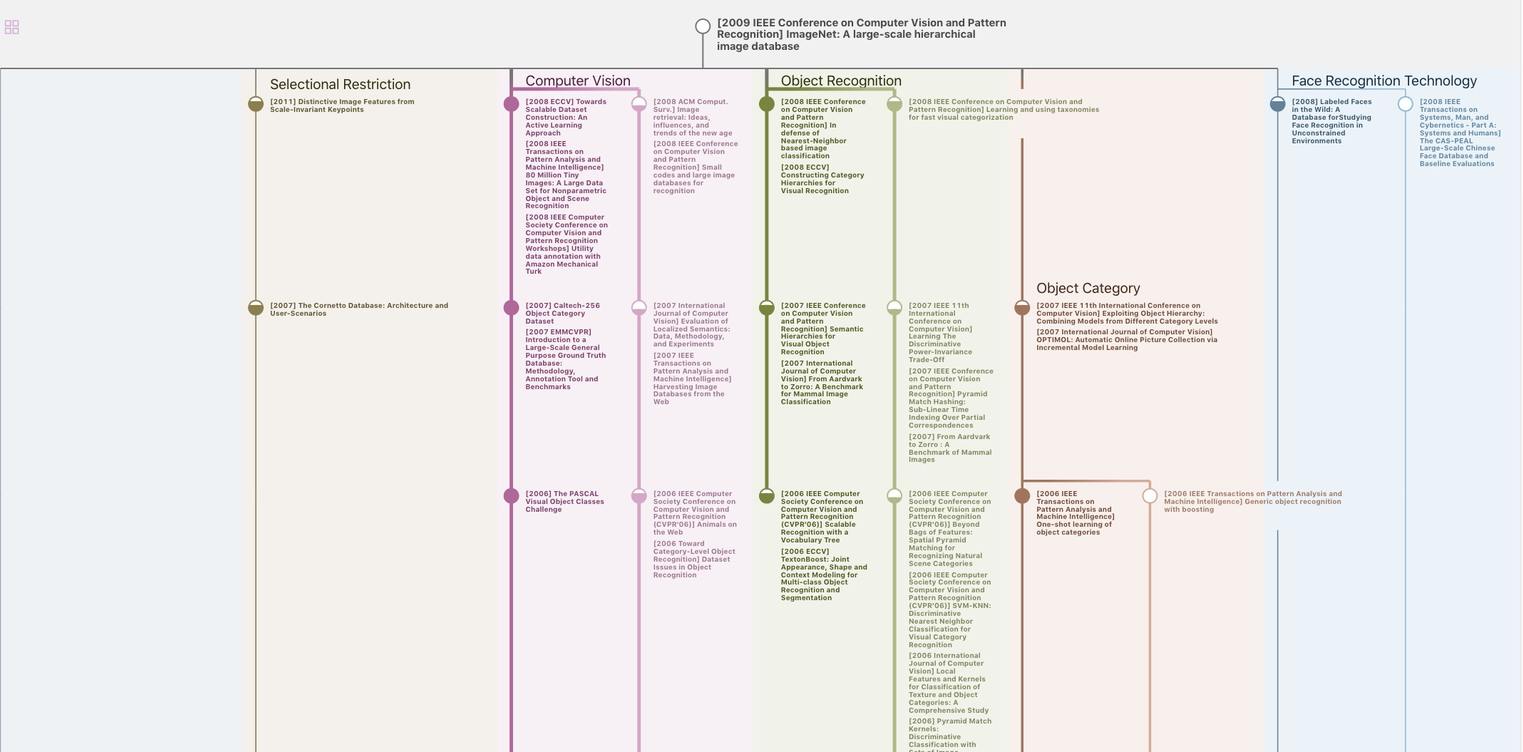
生成溯源树,研究论文发展脉络
Chat Paper
正在生成论文摘要