Graph-based Alignment and Uniformity for Recommendation
PROCEEDINGS OF THE 32ND ACM INTERNATIONAL CONFERENCE ON INFORMATION AND KNOWLEDGE MANAGEMENT, CIKM 2023(2023)
摘要
Collaborative filtering-based recommender systems (RecSys) rely on learning representations for users and items to predict preferences accurately. Representation learning on the hypersphere is a promising approach due to its desirable properties, such as alignment and uniformity. However, the sparsity issue arises when it encounters RecSys. To address this issue, we propose a novel approach, graph-based alignment and uniformity (GraphAU), that explicitly considers high-order connectivities in the user-item bipartite graph. GraphAU aligns the user/item embedding to the dense vector representations of high-order neighbors using a neighborhood aggregator, eliminating the need to compute the burdensome alignment to high-order neighborhoods individually. To address the discrepancy in alignment losses, GraphAU includes a layer-wise alignment pooling module to integrate alignment losses layer-wise. Experiments on four datasets show that GraphAU significantly alleviates the sparsity issue and achieves state-of-the-art performance. We open-source GraphAU at https://github.com/YangLiangwei/GraphAU.
更多查看译文
关键词
Graph Neural Network,Recommendation System,Alignment
AI 理解论文
溯源树
样例
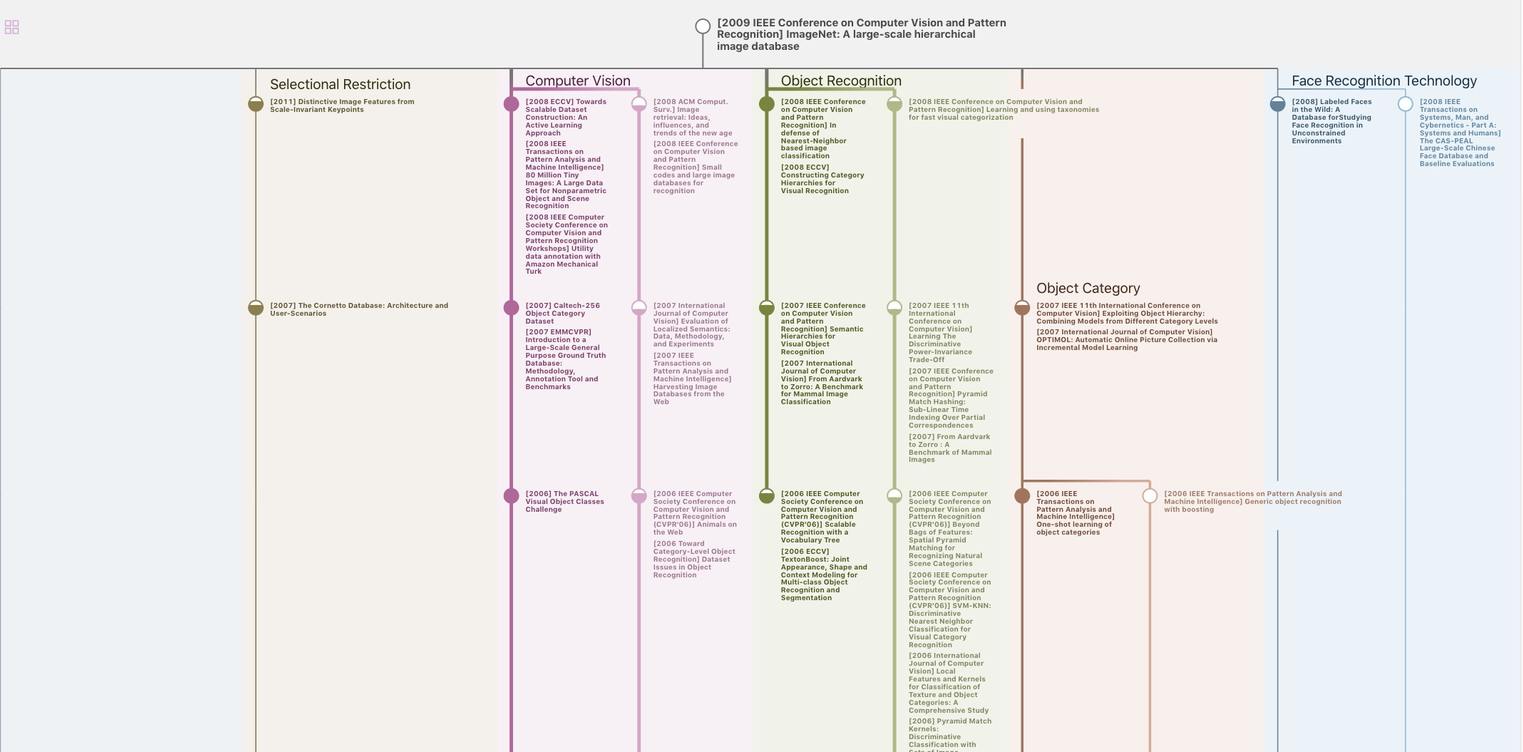
生成溯源树,研究论文发展脉络
Chat Paper
正在生成论文摘要