Bee detection in bee hives using selective features from acoustic data
Multimedia Tools and Applications(2024)
摘要
Honeybees, a key pollinator of the world’s most cultivated crops, are experiencing colony collapses due to a variety of factors. The existence of honeybees and queens is critical to the sociality of a colony, and the presence of bees in agricultural settings is vital to the ecological balance. Moreover, beehives without a queen may lead to the decline of an entire colony therefore finding them through effective and an accurate approach is a critical task. In this scenario, we analyzed acoustic/sound data of various classes (i.e. Bee, NoBee, and NoQueen) from beehive colonies. This study examines five distinct features including spectral centroid, zero-crossing rate, Mel-frequency cepstral coefficients (MFCC), chromagram, and constant Q-transform characteristics for their suitability in detecting bees using the acoustic data. In addition, selective features using principal component analysis (PCA), Chi-square analysis (Chi2), and singular value decomposition (SVD) are used. Moreover, the study proposes hybrid features where selective PCA, Chi2, and SVD characteristics are integrated to create a suitable feature set. Experimental results exhibit the suitability of the hybrid feature set which outperformed individual features for “Bee”, “NoBee” and “NoQueen” classes prediction. Cross-validation and T-test results also confirm the superior performance of hybrid MFCC features. The results indicate that RF and KNN show better performance than other machine learning models with maximum accuracy scores of 0.82 and 0.83, respectively.
更多查看译文
关键词
Bee detection,Acoustic analysis,Feature engineering,Mel-frequency cepstral features
AI 理解论文
溯源树
样例
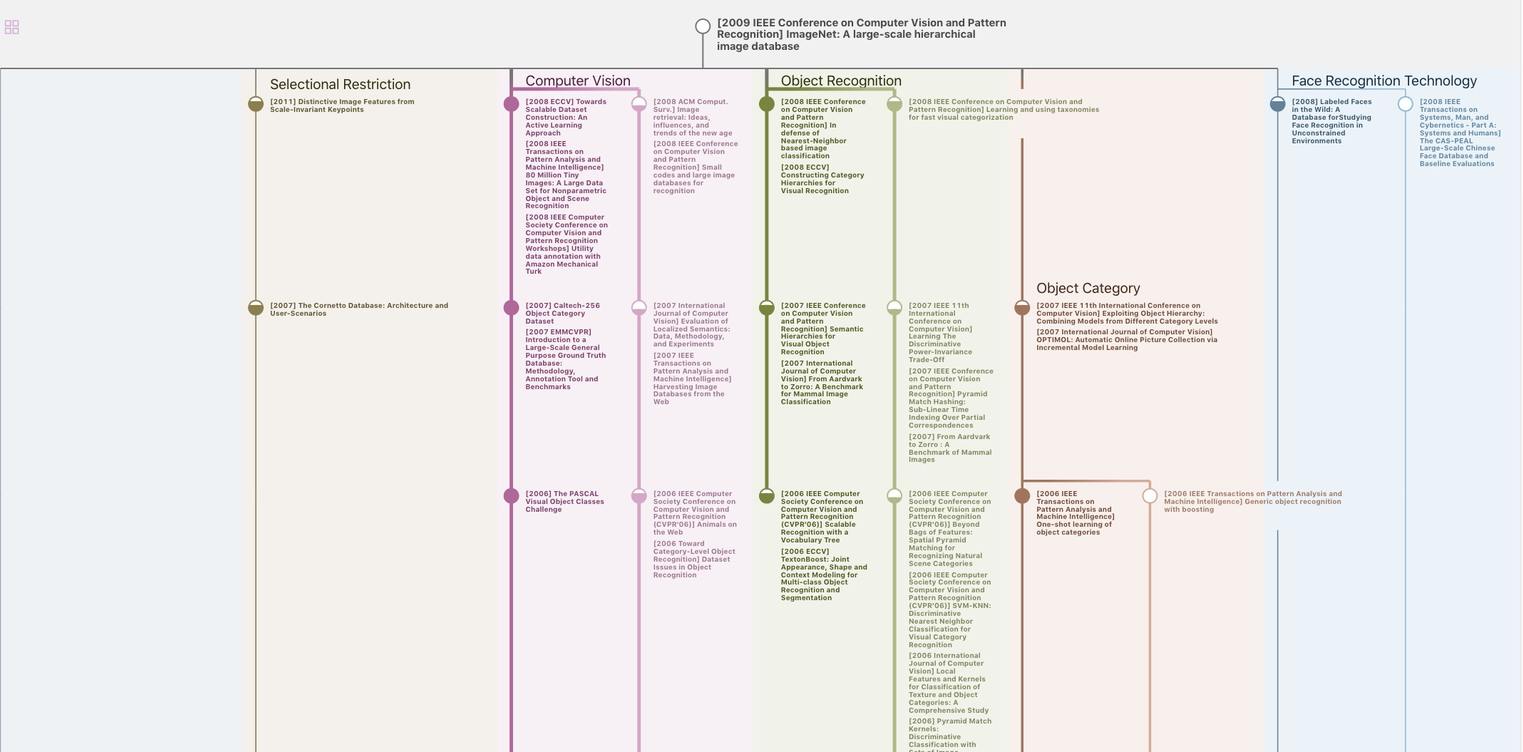
生成溯源树,研究论文发展脉络
Chat Paper
正在生成论文摘要