Tabular data synthesis with generative adversarial networks: design space and optimizations
The VLDB Journal(2024)
摘要
The proliferation of big data has brought an urgent demand for privacy-preserving data publishing. Traditional solutions to this demand have limitations on effectively balancing the trade-off between privacy and utility of the released data. To address this problem, the database community and machine learning community have recently studied a new problem of tabular data synthesis using generative adversarial networks (GANs) and proposed various algorithms. However, a comprehensive comparison between GAN-based methods and conventional approaches is still lacking, making it unclear why and how GANs can outperform conventional approaches in synthesizing tabular data. Moreover, it is difficult for practitioners to understand which components are necessary when building a GAN model for tabular data synthesis. To bridge this gap, we conduct a comprehensive experimental study that investigates applying GAN to tabular data synthesis. We introduce a unified GAN-based framework and define a space of design solutions for each component in the framework, including neural network architectures and training strategies. We provide optimization techniques to handle difficulties in training GAN in practice. We conduct extensive experiments to explore the design space, comparing with traditional data synthesis approaches. Through extensive experiments, we find that GAN is very promising for tabular data synthesis and provide guidance for selecting appropriate design choices. We also point out limitations of GAN and identify future research directions. We make all code and datasets public for future research.
更多查看译文
关键词
Tabular data synthesis,Generative adversarial networks,GAN optimizations,Data privacy
AI 理解论文
溯源树
样例
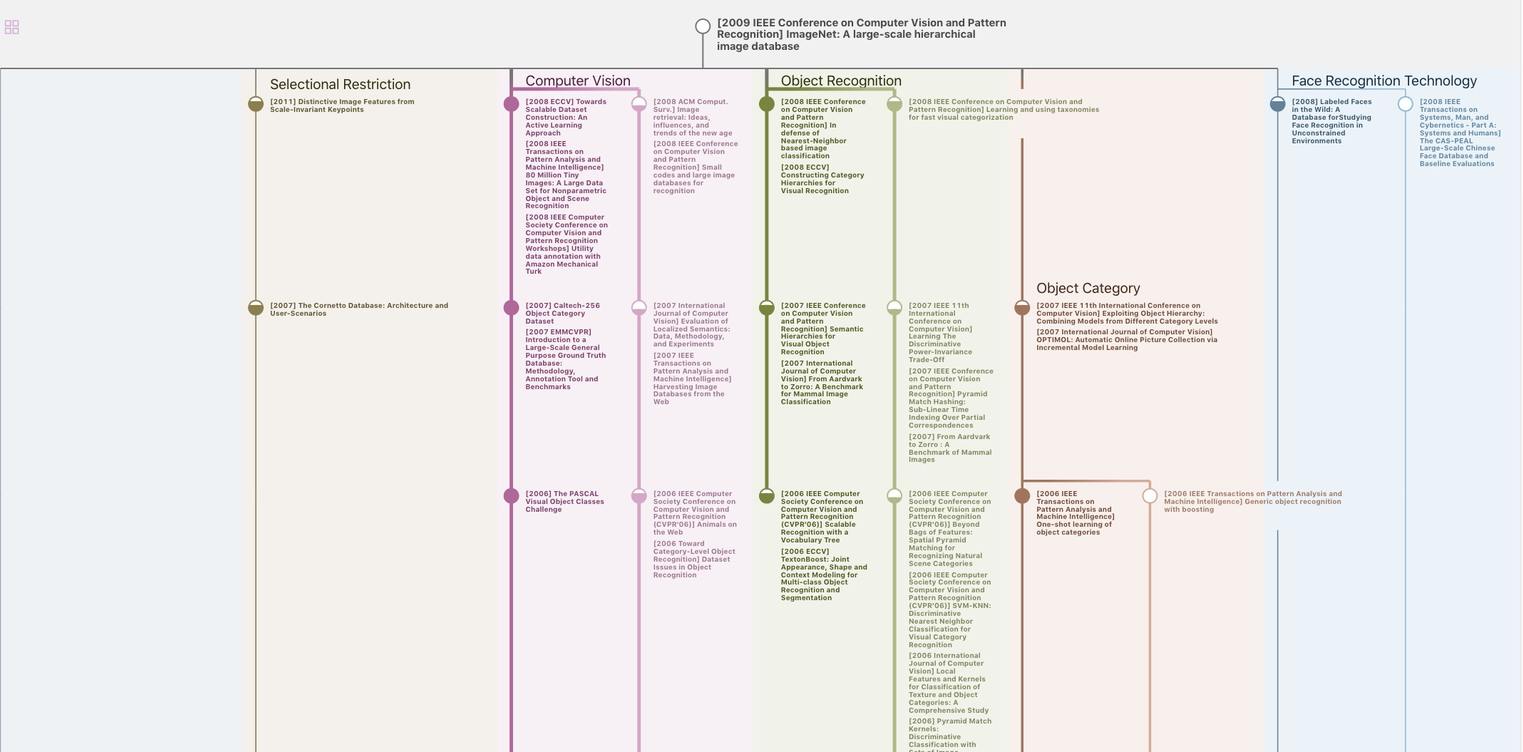
生成溯源树,研究论文发展脉络
Chat Paper
正在生成论文摘要