Learning Complete Protein Representation by Deep Coupling of Sequence and Structure
bioRxiv (Cold Spring Harbor Laboratory)(2023)
摘要
Learning effective representations is crucial for understanding proteins and their biological functions. Recent advancements in language models and graph neural networks have enabled protein models to leverage primary or tertiary structure information to learn representations. However, the lack of practical methods to deeply co-model the relationships between protein sequences and structures has led to suboptimal embeddings. In this work, we propose CoupleNet, a network that couples protein sequence and structure to obtain informative protein representations. CoupleNet incorporates multiple levels of features in proteins, including the residue identities and positions for sequences, as well as geometric representations for tertiary structures. We construct two types of graphs to model the extracted sequential features and structural geometries, achieving completeness on these graphs, respectively, and perform convolution on nodes and edges simultaneously to obtain superior embeddings. Experimental results on a range of tasks, such as protein fold classification and function prediction, demonstrate that our proposed model outperforms the state-of-the-art methods by large margins.
### Competing Interest Statement
The authors have declared no competing interest.
更多查看译文
关键词
complete protein representation,deep coupling,structure
AI 理解论文
溯源树
样例
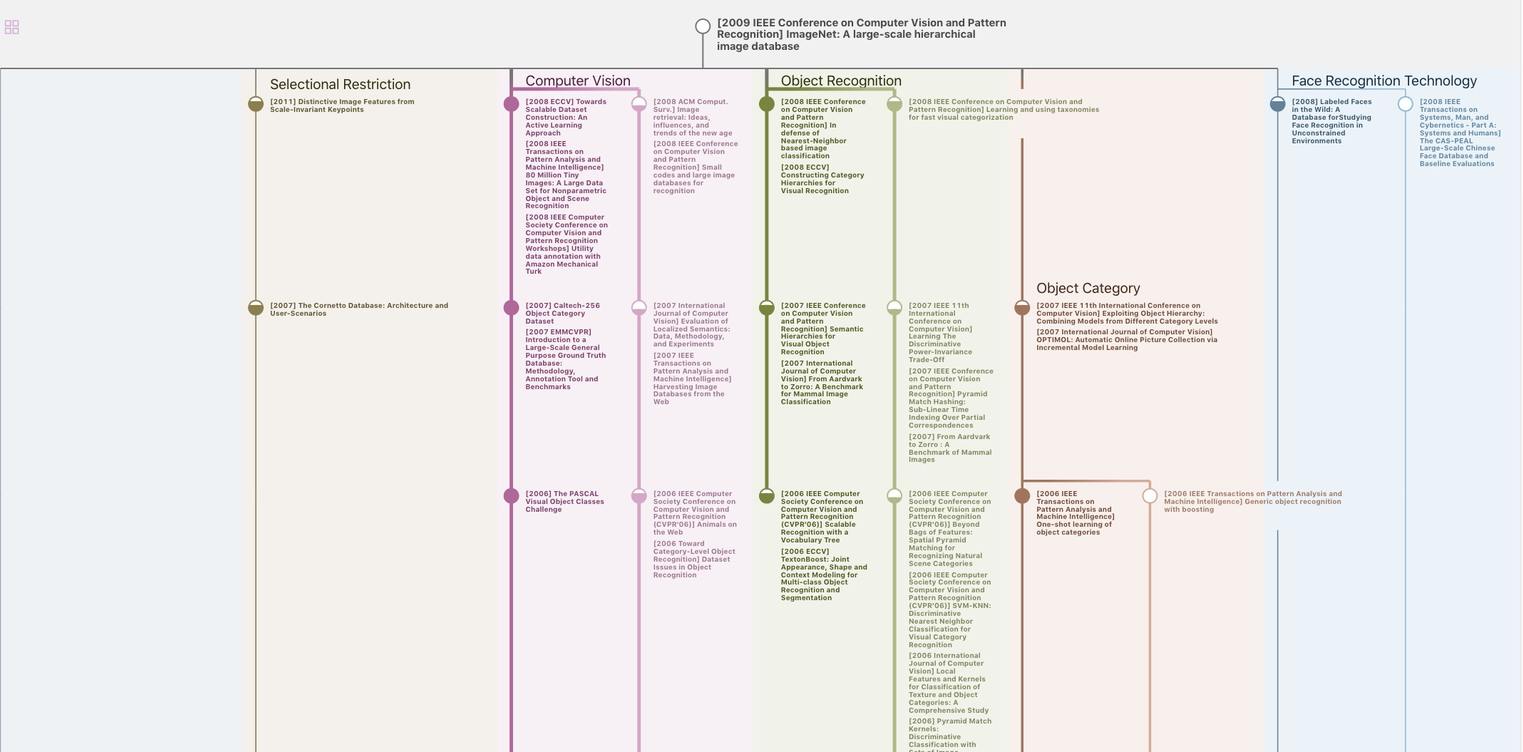
生成溯源树,研究论文发展脉络
Chat Paper
正在生成论文摘要